The healthcare industry is rapidly evolving, and one of the most exciting advancements is the use of Artificial Intelligence (AI) in predictive analytics. This technology is transforming how we predict, diagnose, and treat various health conditions. By analyzing vast amounts of data, AI can provide insights that were previously unimaginable, making healthcare more proactive and personalized. In this article, we will explore the innovative ways AI-driven predictive analytics is revolutionizing healthcare, improving patient outcomes, and shaping the future of medical practices.
The Rise of AI in Healthcare
AI has been making waves across different industries, and healthcare is no exception. The ability of AI to process and analyze massive datasets quickly and accurately has opened new possibilities for predictive analytics in medicine. This shift is moving healthcare from a reactive approach to a more proactive one, focusing on prevention and early intervention.
Enhancing Diagnosis Accuracy
One of the most significant impacts of AI in healthcare is its ability to enhance diagnostic accuracy. Traditional diagnostic methods rely heavily on the expertise and experience of healthcare professionals, which can sometimes lead to errors. AI, however, can analyze medical images, lab results, and patient history with incredible precision.
For example, AI algorithms can detect patterns in imaging scans that might be missed by the human eye. In cases of diseases like cancer, early detection is crucial. AI can identify abnormal growths or tissues in their early stages, enabling timely intervention and increasing the chances of successful treatment.
Predicting Disease Outbreaks
Predictive analytics is not just limited to individual patient care; it also plays a vital role in public health. AI can analyze data from various sources, including social media, travel patterns, and weather conditions, to predict disease outbreaks. By identifying potential hotspots and trends, healthcare authorities can take preventive measures to contain the spread of infectious diseases.
For instance, during the COVID-19 pandemic, AI models were used to predict the spread of the virus, helping governments and health organizations make informed decisions about lockdowns, resource allocation, and vaccination strategies. This proactive approach can save lives and reduce the burden on healthcare systems.
Personalizing Treatment Plans
Every patient is unique, and so are their health needs. AI-driven predictive analytics can help create personalized treatment plans tailored to individual patients. By analyzing genetic information, lifestyle factors, and medical history, AI can predict how a patient will respond to different treatments.
For example, in the field of oncology, AI can analyze a patient’s genetic makeup to determine the most effective chemotherapy regimen. This personalized approach increases the chances of treatment success and minimizes adverse effects. It also reduces the trial-and-error approach often seen in traditional treatment methods.
Reducing Hospital Readmissions
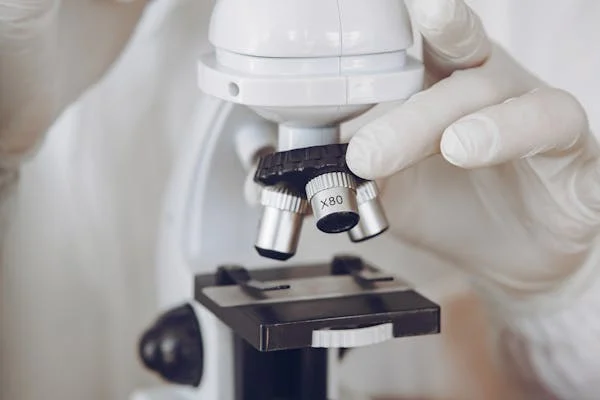
Hospital readmissions are a significant challenge for healthcare systems, often leading to increased costs and patient dissatisfaction. Predictive analytics can help reduce readmission rates by identifying patients at high risk of complications after discharge.
AI can analyze patient data to predict the likelihood of readmission and suggest preventive measures. For instance, patients with chronic conditions like heart disease or diabetes can be closely monitored through remote health management systems. These systems can alert healthcare providers to potential issues, allowing for timely intervention and reducing the need for readmission.
Optimizing Resource Allocation
Efficient resource allocation is critical in healthcare, where resources are often limited. AI-driven predictive analytics can help hospitals and clinics optimize their resources by predicting patient influx and resource needs.
For example, during flu season, AI can analyze historical data and current trends to predict the number of patients likely to visit emergency rooms. This information helps healthcare facilities allocate staff, beds, and medical supplies more effectively, ensuring that they are prepared to handle the increased demand.
AI in Predictive Healthcare: Key Innovations
Early Detection of Chronic Diseases
Chronic diseases such as diabetes, cardiovascular diseases, and hypertension are leading causes of morbidity and mortality worldwide. Early detection and management of these conditions are crucial for improving patient outcomes. AI in predictive analytics is playing a transformative role in this aspect.
For instance, AI algorithms can analyze electronic health records (EHRs) to identify patients at high risk for developing chronic diseases.
By examining patterns in the data, such as blood pressure readings, cholesterol levels, and lifestyle factors, AI can predict the likelihood of a patient developing a chronic condition. This enables healthcare providers to implement preventive measures, such as lifestyle modifications or medications, to delay or prevent the onset of the disease.
Predicting Patient Deterioration
In critical care settings, timely intervention can be the difference between life and death. AI-driven predictive analytics can help monitor patients in real-time and predict potential deterioration before it becomes critical.
For example, in intensive care units (ICUs), AI systems can continuously analyze data from various sources, including vital signs, lab results, and nurse notes.
These systems can detect subtle changes that may indicate a patient is at risk of deterioration, such as sepsis or respiratory failure. Early warnings from AI can prompt healthcare providers to take immediate action, improving patient outcomes and reducing mortality rates.
Enhancing Mental Health Care
Mental health is an area where AI-driven predictive analytics can make a significant impact. Mental health conditions are often underdiagnosed and undertreated due to the stigma and lack of awareness. AI can help bridge this gap by analyzing data from various sources to predict and identify mental health issues early.
For instance, AI algorithms can analyze social media activity, online behavior, and digital health records to identify patterns indicative of mental health conditions such as depression or anxiety. By detecting these patterns early, healthcare providers can offer timely interventions, such as counseling or medication, to manage the condition before it worsens.
Predictive Analytics in Genomics
Genomics is a rapidly evolving field, and AI-driven predictive analytics is at the forefront of its advancements. By analyzing genetic data, AI can predict an individual’s susceptibility to certain diseases and their likely response to treatments.
For example, AI can analyze a person’s genome to predict their risk of hereditary conditions like breast cancer or Alzheimer’s disease. This information can guide preventive measures, such as more frequent screenings or lifestyle changes.
Additionally, in personalized medicine, AI can predict how patients will respond to specific drugs based on their genetic makeup, ensuring that treatments are tailored to their unique needs.
AI in Drug Development
The process of developing new drugs is time-consuming and expensive. AI-driven predictive analytics is revolutionizing this process by making it more efficient and cost-effective. AI can analyze vast amounts of data from clinical trials, research papers, and patient records to identify potential drug candidates and predict their efficacy and safety.
For example, AI algorithms can predict how different compounds will interact with biological targets, speeding up the drug discovery process.
Additionally, predictive analytics can help design more efficient clinical trials by identifying the most suitable patient populations and predicting potential side effects. This accelerates the development of new drugs and brings them to market faster, benefiting patients who need them.
Improving Population Health Management
Population health management focuses on improving health outcomes for groups of individuals. AI-driven predictive analytics plays a vital role in this area by identifying trends and patterns that can inform public health strategies.
For example, AI can analyze data from various sources, including EHRs, social determinants of health, and community health surveys, to identify at-risk populations.
This information can guide targeted interventions, such as vaccination campaigns or health education programs, to improve overall health outcomes. Predictive analytics can also help healthcare providers allocate resources more effectively, ensuring that interventions reach those who need them most.
The Role of AI in Operational Efficiency
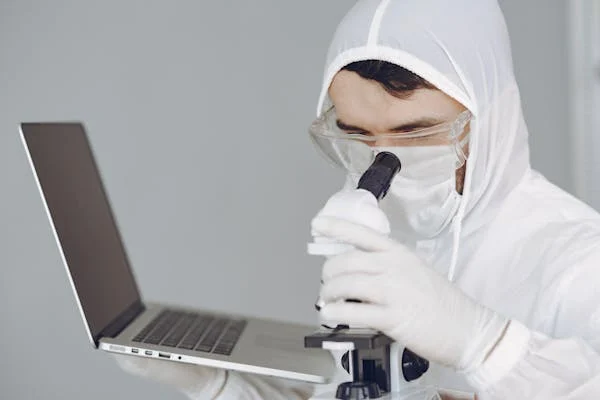
For startup founders in the healthcare industry, leveraging AI in predictive analytics to enhance operational efficiency can be a game-changer. Here’s how AI can strategically transform your operations and help you navigate the competitive healthcare landscape.
Strategic Patient Flow Management
One of the critical challenges in healthcare is managing patient flow efficiently. For startups, optimizing patient flow can significantly enhance patient satisfaction and operational efficiency.
AI-driven predictive analytics can forecast patient arrivals, identify peak times, and suggest optimal staff allocation. This foresight allows for the strategic planning of resources, reducing waiting times and ensuring that patients receive timely care.
By implementing AI solutions that predict patient flow, you can create a more responsive and flexible scheduling system.
This not only improves the patient experience but also maximizes the utilization of healthcare professionals, reducing downtime and overwork. As a startup, being able to efficiently manage patient flow can differentiate you from established players who might struggle with outdated systems.
Intelligent Appointment Scheduling
For healthcare startups, offering an exceptional patient experience from the first point of contact is crucial. AI can transform appointment scheduling by predicting no-shows and cancellations, allowing for overbooking strategies that minimize idle time for practitioners. AI can also recommend appointment slots based on historical patient preferences and patterns, increasing the likelihood of patient attendance and satisfaction.
By integrating AI into your appointment scheduling system, you can optimize the schedule to accommodate more patients without overwhelming your staff. This strategic approach can enhance patient throughput, leading to higher revenue and improved patient satisfaction. Ensuring that your scheduling system is both efficient and patient-centric can set your startup apart in the competitive healthcare market.
Resource Optimization and Cost Management
For startups, efficient resource utilization is vital for sustainability and growth. AI-driven predictive analytics can provide insights into inventory management, helping you maintain optimal levels of medical supplies and medications. Predictive models can forecast usage patterns, enabling you to order supplies just in time, reducing storage costs and minimizing waste.
Additionally, AI can analyze operational data to identify cost-saving opportunities. For example, by predicting high and low demand periods, you can strategically plan staff shifts and reduce overtime costs.
This level of resource optimization ensures that you are not only meeting patient needs efficiently but also managing your budget effectively. For startups, maintaining a lean operation without compromising on quality is essential for long-term success.
Enhancing Clinical Workflow
Streamlining clinical workflows is crucial for delivering high-quality care while maintaining efficiency. AI can automate routine tasks such as patient data entry, billing, and reporting, freeing up healthcare professionals to focus on patient care. By reducing the administrative burden, AI allows clinicians to spend more time on direct patient interactions, improving the overall quality of care.
Implementing AI in clinical workflows can also enhance communication and coordination among healthcare teams. Predictive analytics can suggest the most efficient pathways for patient care, ensuring that all necessary tests and consultations are scheduled promptly.
This coordination reduces delays and redundancies, providing a smoother patient journey. For a startup, adopting AI to streamline clinical workflows can result in higher patient satisfaction and better clinical outcomes.
Proactive Equipment Maintenance
For healthcare startups, the maintenance of medical equipment is a significant concern. Unexpected equipment failures can disrupt operations and affect patient care. AI-driven predictive maintenance can analyze usage patterns and performance data to predict when equipment is likely to fail.
This proactive approach allows you to schedule maintenance before issues arise, reducing downtime and ensuring that your equipment is always in optimal condition.
By investing in AI-powered predictive maintenance, you can avoid costly repairs and extend the lifespan of your equipment. This strategic move not only saves money but also ensures that your operations run smoothly without interruptions. For startups, maintaining reliable equipment is crucial for building a reputation for quality and dependability.
Strategic Data Utilization
Data is a valuable asset for any healthcare startup. AI-driven predictive analytics can help you make the most of your data by providing actionable insights. By analyzing patient data, operational metrics, and market trends, AI can identify opportunities for improvement and growth.
For example, predictive analytics can reveal patterns in patient demographics and behaviors, helping you tailor your services to meet specific needs. This targeted approach can attract more patients and improve retention rates.
Additionally, AI can provide insights into market trends, guiding your strategic decisions on service expansions or new offerings. Leveraging data strategically ensures that your startup remains agile and responsive to changing market demands.
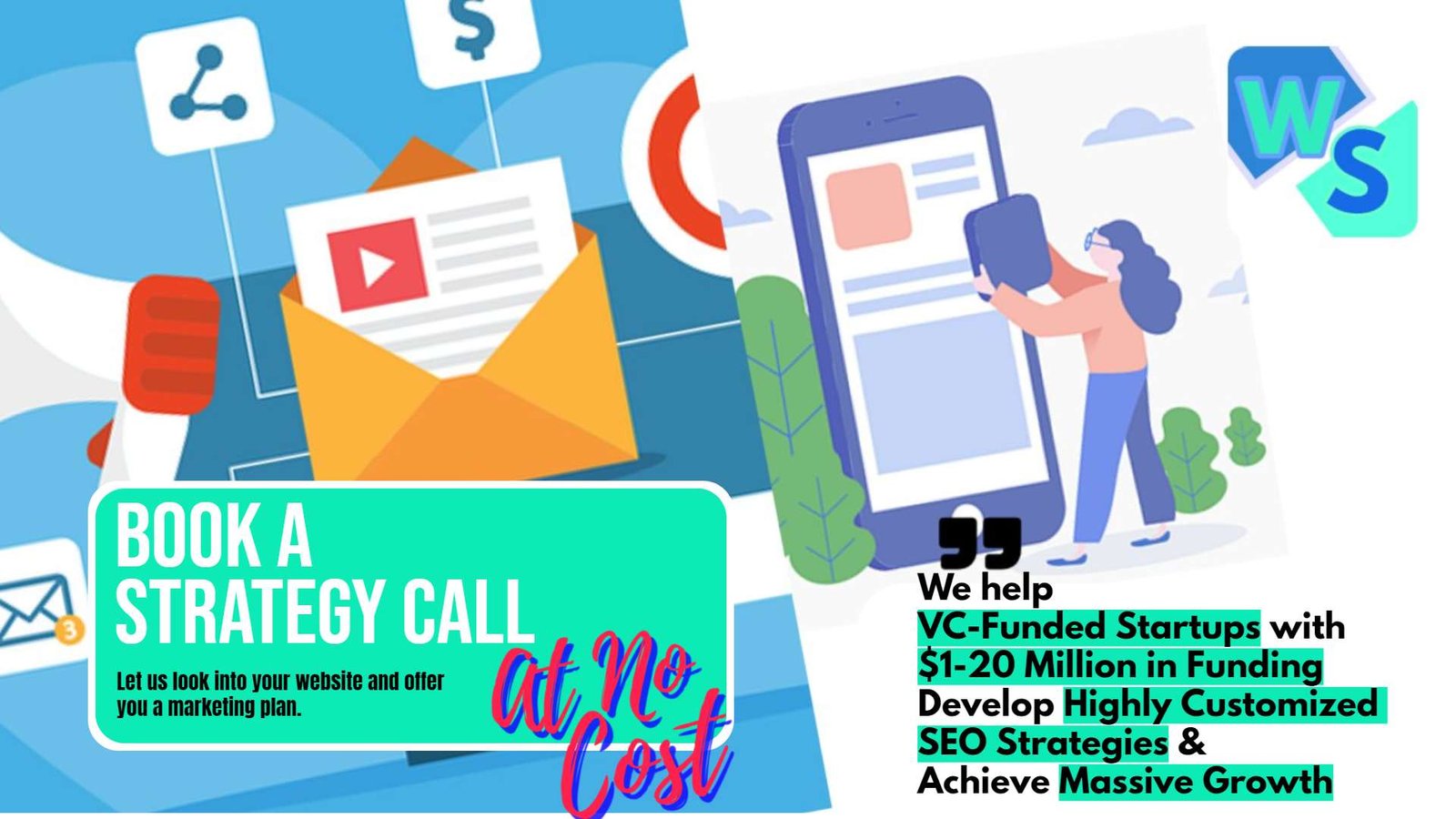
Related: Check out our free tools:
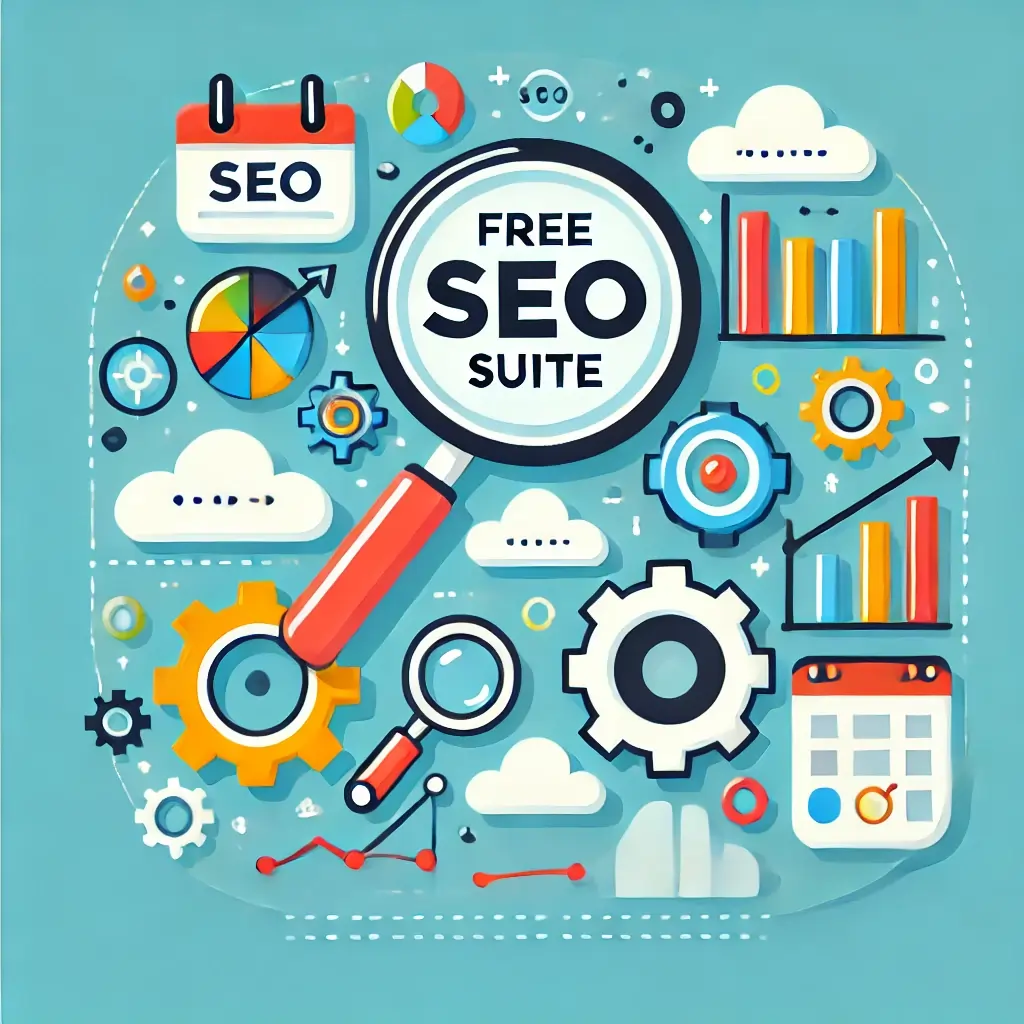
Cultivating a Culture of Innovation
For healthcare startups, fostering a culture of innovation is essential for staying competitive. Integrating AI into your operations not only improves efficiency but also positions your startup as a forward-thinking leader in the industry. Encourage your team to embrace AI-driven solutions and continuously seek ways to innovate and improve.
By creating an environment that values innovation, you can attract top talent and build a reputation for cutting-edge healthcare solutions. This culture of continuous improvement ensures that your startup remains at the forefront of technological advancements, driving sustained growth and success.
AI in Personalized Medicine
Tailoring Treatments to Individual Patients
Personalized medicine aims to tailor treatments to individual patients based on their unique characteristics. AI-driven predictive analytics is a powerful tool in achieving this goal. By analyzing genetic data, lifestyle factors, and medical history, AI can predict how a patient will respond to different treatments.
For example, in oncology, AI can analyze a patient’s genetic profile to identify mutations that drive cancer growth. This information helps oncologists choose targeted therapies that are more likely to be effective. Similarly, in pharmacogenomics, AI can predict how patients will metabolize certain drugs, reducing the risk of adverse reactions and improving treatment outcomes.
Predicting Disease Progression
Understanding how a disease is likely to progress is crucial for effective management and treatment. AI-driven predictive analytics can analyze patient data to predict disease progression and guide treatment decisions.
For instance, in chronic conditions like diabetes, AI can predict how the disease will evolve based on factors such as blood glucose levels, lifestyle, and comorbidities. This information allows healthcare providers to adjust treatment plans proactively, preventing complications and improving patient outcomes. Predictive analytics can also help in monitoring disease progression and adjusting therapies as needed.
Enhancing Preventive Care
Preventive care focuses on preventing diseases before they occur. AI-driven predictive analytics plays a significant role in enhancing preventive care by identifying risk factors and suggesting preventive measures.
For example, AI can analyze data from wearable devices to monitor physical activity, sleep patterns, and vital signs. This information can identify individuals at risk of developing chronic conditions like hypertension or obesity.
Healthcare providers can then recommend lifestyle changes, such as increased physical activity or dietary modifications, to mitigate these risks. Predictive analytics helps in creating personalized preventive care plans that are more effective in maintaining health.
Improving Clinical Decision Support
Clinical decision support systems (CDSS) assist healthcare providers in making informed decisions about patient care. AI-driven predictive analytics enhances CDSS by providing real-time insights and recommendations based on patient data.
For example, AI can analyze patient symptoms, medical history, and lab results to suggest potential diagnoses and treatment options.
This support helps healthcare providers make accurate and timely decisions, reducing diagnostic errors and improving patient outcomes. Predictive analytics can also identify potential drug interactions and contraindications, ensuring patient safety.
AI in Predictive Analytics for Population Health
Monitoring Public Health Trends
AI-driven predictive analytics is revolutionizing public health by enabling continuous monitoring of health trends. By analyzing data from multiple sources such as electronic health records (EHRs), social media, and health surveys, AI can identify emerging health threats and trends.
For example, during the flu season, AI can monitor the spread of influenza in real-time, predicting which areas are likely to experience outbreaks. This information allows public health officials to deploy resources and interventions where they are needed most. Continuous monitoring helps in early detection of public health issues, leading to timely responses that can mitigate the impact on communities.
Identifying At-Risk Populations
Certain populations are at higher risk for specific health conditions due to factors like genetics, lifestyle, and socioeconomic status. AI-driven predictive analytics can identify these at-risk populations, enabling targeted interventions.
For instance, AI can analyze demographic data, health records, and social determinants of health to identify communities at risk for conditions like diabetes or cardiovascular diseases. Public health programs can then be tailored to these communities, focusing on preventive measures such as health education, screenings, and lifestyle modifications. Targeted interventions improve health outcomes and reduce healthcare disparities.
Enhancing Vaccination Campaigns
Vaccination campaigns are crucial for preventing infectious diseases. AI-driven predictive analytics can enhance these campaigns by predicting vaccination needs and optimizing distribution.
For example, AI can analyze historical vaccination data, population demographics, and disease prevalence to forecast the demand for vaccines. This information helps public health officials plan vaccination campaigns more effectively, ensuring that vaccines are distributed to areas with the highest need. Predictive analytics can also identify gaps in vaccination coverage, guiding efforts to reach underserved populations.
Managing Health Crises
In times of health crises, such as pandemics or natural disasters, quick and effective response is vital. AI-driven predictive analytics can assist in managing these crises by providing actionable insights and forecasts.
For instance, during the COVID-19 pandemic, AI models were used to predict the spread of the virus, identify hotspots, and allocate medical resources. This proactive approach helped in flattening the curve and managing healthcare capacity.
In natural disasters, AI can predict the health impacts on affected populations, guiding relief efforts and resource allocation. Predictive analytics ensures that responses are timely and well-coordinated, minimizing the impact on public health.
Improving Health Policy Decisions
Effective health policy decisions are based on accurate and comprehensive data. AI-driven predictive analytics provides policymakers with the insights needed to make informed decisions that improve public health.
For example, AI can analyze the impact of existing health policies, identify areas for improvement, and predict the outcomes of proposed policies.
This evidence-based approach ensures that policies are effective in addressing public health challenges. Predictive analytics also helps in evaluating the long-term effects of health interventions, guiding sustainable health policy development.
Challenges and Ethical Considerations
Data Privacy and Security
The use of AI in predictive analytics involves handling vast amounts of sensitive health data. Ensuring data privacy and security is paramount to protect patient confidentiality and maintain trust.
For example, healthcare providers must comply with regulations such as the Health Insurance Portability and Accountability Act (HIPAA) to safeguard patient data. This includes implementing robust security measures like encryption and access controls.
Additionally, patients should be informed about how their data is used and given the option to consent to its use. Ensuring data privacy and security protects patients and fosters trust in AI-driven healthcare solutions.
Bias and Fairness
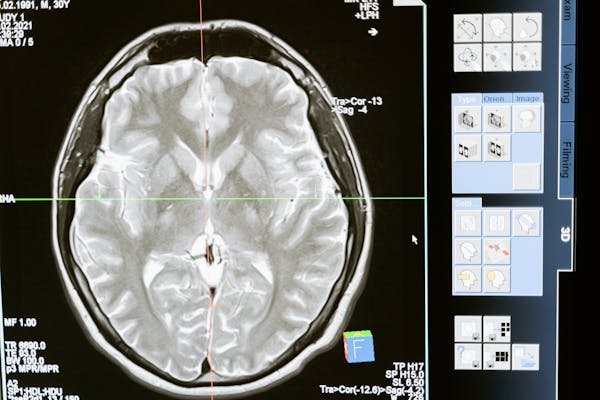
AI algorithms are only as good as the data they are trained on. If the training data contains biases, the AI models may produce biased outcomes, leading to unfair treatment.
For example, if an AI model is trained predominantly on data from one demographic group, it may not perform well for other groups. This can result in disparities in healthcare outcomes. To address this issue, it is crucial to use diverse and representative datasets for training AI models.
Additionally, regular audits and evaluations of AI systems can help identify and mitigate biases. Ensuring fairness in AI-driven predictive analytics promotes equitable healthcare for all.
Transparency and Accountability
AI-driven predictive analytics must be transparent and accountable. Healthcare providers and patients should understand how AI models make predictions and the rationale behind their decisions.
For instance, if an AI model predicts that a patient is at high risk for a condition, healthcare providers should be able to explain the factors contributing to this prediction. This transparency helps in building trust and allows for informed decision-making.
Additionally, there should be mechanisms in place to hold AI systems accountable for their performance and outcomes. Regular monitoring and updates ensure that AI models remain accurate and reliable.
Ethical Use of AI
The ethical use of AI in predictive analytics involves considering the broader implications of its deployment. This includes ensuring that AI is used to enhance healthcare without replacing the human touch.
For example, while AI can provide valuable insights and recommendations, healthcare providers should continue to exercise their clinical judgment and maintain the patient-provider relationship.
Ethical considerations also involve addressing the potential for AI to exacerbate existing inequalities in healthcare access and outcomes. By prioritizing ethical principles, healthcare providers can ensure that AI-driven predictive analytics benefits all patients.
Conclusion
AI-driven predictive analytics is revolutionizing healthcare, offering transformative solutions that enhance operational efficiency, improve patient outcomes, and drive innovation. For startup founders in the healthcare sector, leveraging these technologies is not just an option but a necessity for staying competitive and delivering superior care.
Read Next:
- AI in Copywriting: Practical Tips and Tricks
- How AI Transforms Your Content Strategy
- Master AI-Powered Content Creation Techniques
- AI for SEO-Optimized Content Creation
- Enhance Your Blogs with AI Writing
Comments are closed.