In today’s fast-paced digital world, understanding your market is more critical than ever. Market research helps businesses grasp what their customers need and want. With the advent of Artificial Intelligence (AI), this task has become easier and more efficient. AI-driven analytics is transforming how we collect, analyze, and interpret data. This article dives into the latest trends in market research, showcasing how AI is at the forefront of this revolution.
The Evolution of Market Research
Market research has come a long way from simple surveys and focus groups. Traditionally, businesses relied on manual data collection methods. These methods were time-consuming and often prone to errors. Today, technology has revolutionized market research, making it more accurate and efficient.
AI-Powered Data Collection
One of the significant changes AI has brought is in data collection. AI tools can scan vast amounts of data from various sources in minutes. Social media, customer reviews, and online forums are treasure troves of information. AI algorithms can quickly gather this data, providing businesses with valuable insights.
For example, sentiment analysis tools can analyze customer reviews and social media posts to gauge public opinion. These tools can identify positive or negative sentiments, helping businesses understand customer satisfaction levels. This kind of real-time data collection was impossible with traditional methods.
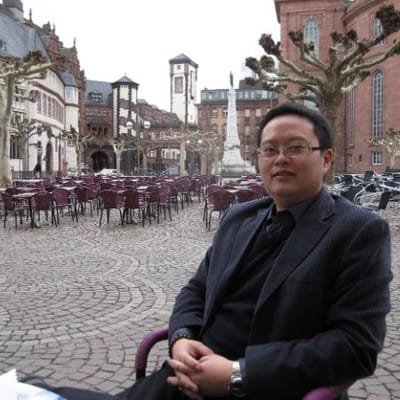
Marketer that I am, I take advantage of AI tools so that, in the end, clients will be able to make timely, data-driven decisions with unparalleled efficiency.
In my practice, AI made a great difference to collect data by combining huge processing power with real-time sourcing and enhanced accuracy and actionability of insights.
Artificial Intelligence-Driven Data Collection from Diverse Sources
We worked with a retail client to monitor brand sentiment across social platforms and review sites. AI algorithms helped in finding the trends in customer preference and flag negative sentiment spikes in real-time.
This approach provided fast, actionable insight views and allowed the client to respond proactively. This improved their customer satisfaction score by 15% over six months.
Speed and Accuracy Enhanced in Market Research
Our AI-enabled system highlighted the emerging product features that competitors were integrating into their offerings, and thus we could make necessary adjustments to the client’s strategy in a very short time.
It saved not only a great deal of time but also placed our client at the forefront in terms of the integration of innovative features for competitive advantage.
Future of AI in Market Research
The right AI tools amplify not just data collection but also translate such data into actionable insights for growth.
Where insight needs to be delivered quicker and more accurately, AI-driven analytics is fast becoming an indispensable tool for businesses in transforming market research into a proactive, precision-guided key to success.
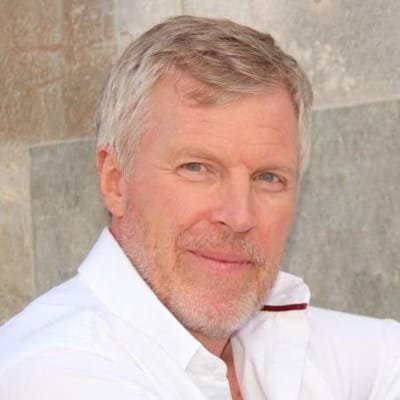
First, AI can cross reference data quickly, and much more efficiently than humans.
This provides much more accurate data at the point of collection. In addition, AI may recognize patterns early on that are invisible to data analysts.
This pattern detection can completely alter the insights gained from the data, and provide organizations with vital information that may have been missed altogether.
Our minds are limited in the associations that we make.
AI is much more likely to make unusual associations and recognize unusual patterns.
Especially considering that AI does not carry our cognitive biases.
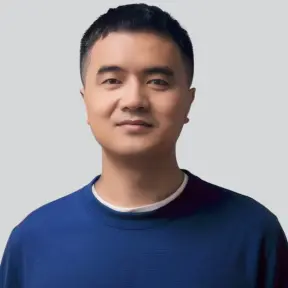
Artificial intelligence has revolutionized how retail businesses gather and analyze data from diverse sources.
One of the most efficient methods employed by these tools is sentiment analysis and contextual understanding.
In this approach, natural language processing (NLP) enables AI tools to determine the tone and context of each piece of data or content they encounter.
Classifications such as positive, negative, and neutral help these tools understand the sentiment of each source.
Additionally, the context can easily be labeled as a complaint, suggestion, or recommendation.
One company that has executed this approach to great success is Amazon.
By employing AI tools to analyze customer reviews and social media sentiment, the company can improve its recommendation algorithms in real time and enhance the customer experience.
These insights also factor into future product developments, helping the company develop products that customers need.
Predictive Analytics
Another breakthrough is predictive analytics. AI algorithms can analyze historical data to predict future trends. This capability is crucial for businesses to stay ahead of the competition. By understanding potential market changes, companies can adjust their strategies proactively.
For instance, retail businesses can use predictive analytics to forecast product demand. This insight helps in managing inventory and avoiding stockouts. Similarly, companies can predict customer behavior, such as purchasing patterns, helping them tailor their marketing strategies effectively.
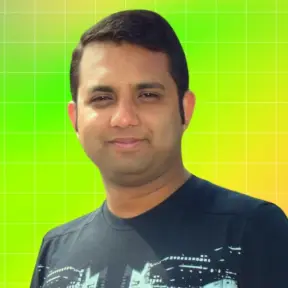
Predictive analytics has turned from a luxury to becoming a need. AI-driven tools will let us map behavioral patterns and consumer trends with a degree of granularity that was unthinkable before.
Take e-commerce for instance. Predictive models will analyze millions of transactional and behavioral data points, revealing hidden preferences about product affinities and timing.
In e-commerce, for instance, predictive models analyze vast quantities of transactional and behavioral data.
This allows businesses to implement dynamic pricing, personalize offers, and even launch seasonal products with peak accuracy, boosting both conversion rates and customer loyalty.
When AI is integrated with predictive analytics, it has become much more important in finance, especially for managing strategies for investment and risk profiling.
Predictive models are built to track spending patterns and compute fraud before it actually happens.
AI has become the engine driving predictive analytics toward its full potential. It’s constantly learning, refining, and optimizing strategies that keep businesses a step ahead.
This is particularly important in moving toward an era of hyper-personalization when customer expectations are not only high but incredibly nuanced.
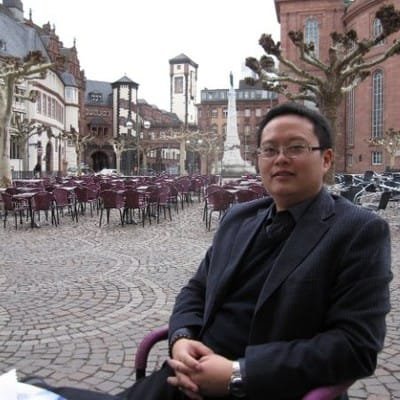
As a market research specialist at XDS, we help our customers provide more accurate demand forecasts.
Predictive analytics enables a company to outpace its competition because it projects historical insight into future strategy; it is, therefore, an indispensable part of today’s market research.
Artificial Intelligence’s Part in Demand Forecasting and Customer Insight
This technique has an application in enabling a retail client to forecast the demand for seasonal clothing to efficiently reduce stockouts during peak shopping periods.
We analyzed buying patterns to maximize the client’s inventory approach, which reduced waste while improving customer satisfaction.
Demand Forecasting in E-commerce
We are going to deploy predictive models that keep us in step with rapidly changing fashion trends. These will enable us to quickly move into the right inventories and quickly respond to changes in demand.
Similarly, for our e-commerce customers, Avlogistics achieves value through this approach using AI-driven forecasting to reduce overstocking by as much as 36.41%. This keeps popular items in stock without inflating storage costs.
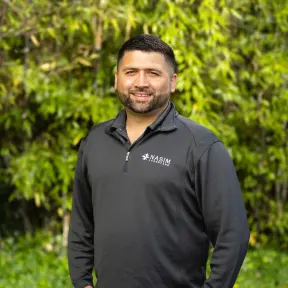
We use data and predictive insights to make smart decisions and stay one step ahead in this ever-changing industry.
1. The role of predictive analytics in forecasting customer behavior and product demand
Predictive analytics is key for understanding what our customers need and for staying ready when demand shifts with the seasons. In landscaping, it helps us pinpoint our busiest times for services like lawn care and snow management, so we can adjust our staffing and resources just right.
By looking at past client trends, we get a clearer picture of what services will be in demand, helping us stay prepared.
2. How AI helps businesses anticipate trends and optimize strategies
AI has become an invaluable tool for us. By using AI-driven software, we analyze historical trends, weather patterns, and even market shifts to predict when certain services will be in high demand.
For example, AI helps us identify when irrigation needs may increase due to drought conditions or when seasonal shifts might drive demand for snow management. This allows us to allocate resources effectively and maintain high-quality service, even during peak seasons.
3. Real-world examples
Industries like e-commerce and finance lean heavily on predictive analytics, and we’ve seen similar value in landscaping. Just like e-commerce companies use browsing and purchase data to suggest products, we use predictive insights to recommend services based on seasonal demand.
Finance companies use analytics to manage risk, while we rely on it to plan staffing and inventory, keeping us ready for any project or seasonal change that comes our way.
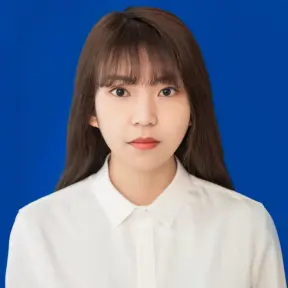
We’ve harnessed the power of predictive analytics to better understand our customers’ needs and forecast demand—a crucial advantage in the ever-evolving e-commerce landscape. I’d love to share our insights on how AI-driven analytics is helping us anticipate trends, meet demand, and optimize our strategies.
How Predictive Analytics Powers Customer Insight at Kabeier
In children’s fashion, customer preferences shift with seasonal trends, age demographics, and social influences, so accurate forecasting is key. By integrating predictive analytics, we analyze past purchasing patterns, seasonal preferences, and even social media trends to forecast demand with precision.
This ensures we’re producing the right styles and quantities at the right time, allowing us to meet demand without overstocking.
AI in Action: Turning Data into Decisions
Using machine learning models, we track engagement data from our website and social platforms, revealing insights like seasonal spikes in certain product categories (think baptism outfits in spring or formalwear during the holidays).
AI also helps us understand nuanced customer behavior, such as preferences for certain colors or styles within specific demographics. This has helped us reduce markdowns by 30% over the past year by ensuring our inventory aligns with real-time demand forecasts.
Example: Tailored Recommendations that Drive Engagement
A recent application has been in our product recommendation engine, where AI personalizes suggestions based on browsing history and previous purchases.
For example, customers who purchase christening outfits are likely to receive recommendations for formal accessories. This not only drives conversions but also deepens customer engagement, making every interaction with Kabeier feel relevant and personalized.
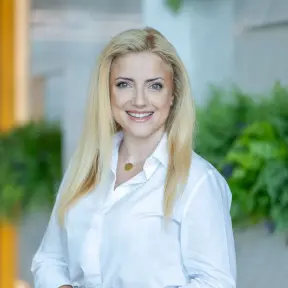
Predictive analytics is really about using past data to predict future actions.
It’s a tool businesses rely on to make sense of customer patterns at scale, similar to regression in psychology but working with huge data sets that capture unstructured information like buying habits or market shifts.
In e-commerce, companies use predictive analytics to forecast what products a customer is likely to purchase next based on past behavior.
In finance, it’s about spotting trends that might indicate investment opportunities or risks.
AI is invaluable here because it manages the heavy lifting—sifting through the enormous amount of data out there to produce useful predictions.
It’s not just about seeing patterns; it’s about creating a model that genuinely anticipates where things are headed, helping businesses refine their strategy and stay a step ahead.
Enhanced Customer Segmentation
Customer segmentation is vital for targeted marketing. AI-driven analytics can segment customers more precisely than ever before. By analyzing various data points like purchasing history, browsing behavior, and social media interactions, AI can identify distinct customer groups.
These segments allow businesses to create personalized marketing campaigns. Personalized content resonates more with customers, leading to higher engagement and conversion rates. This level of precision was challenging to achieve with traditional segmentation methods.
Natural Language Processing (NLP)
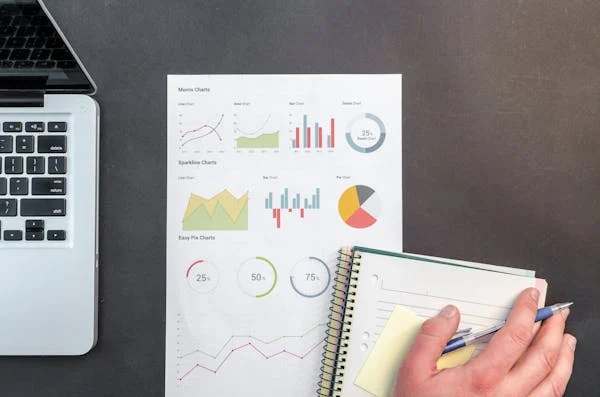
NLP is a branch of AI that deals with the interaction between computers and humans through natural language. In market research, NLP tools can analyze textual data from multiple sources. These tools can understand context, sentiment, and even the underlying intent behind the text.
For example, NLP can process customer feedback to identify common issues or praise points. This analysis provides businesses with actionable insights to improve their products or services. By understanding customer language, companies can also improve their communication strategies.
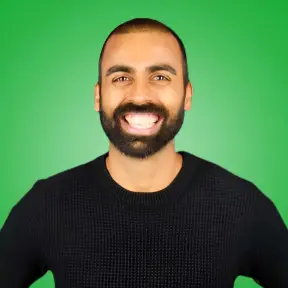
In today’s data-driven world, Natural Language Processing (NLP) has become a transformative tool in market research, allowing brands to dive deeply into customer sentiment with precision and speed.
At Pearl Lemon AI, we’ve seen firsthand how NLP is enhancing customer feedback analysis, brand sentiment monitoring, and trend identification – helping companies make real-time, impactful decisions.
Take customer feedback analysis as an example. NLP can sift through vast amounts of unstructured data from reviews, social media and surveys, translating it into actionable insights that reveal what customers truly feel.
Sentiment monitoring then provides a continuous pulse on brand perception, enabling businesses to detect shifts in sentiment and adjust strategies promptly.
Furthermore, trend identification powered by NLP captures emerging topics across digital landscapes. By analysing patterns in customer conversations, brands can anticipate market needs and proactively adapt, setting them apart in competitive spaces.
A recent case study highlighted how a retail brand using NLP for sentiment analysis improved its product line by isolating common pain points in customer feedback, resulting in a 20% increase in positive reviews.
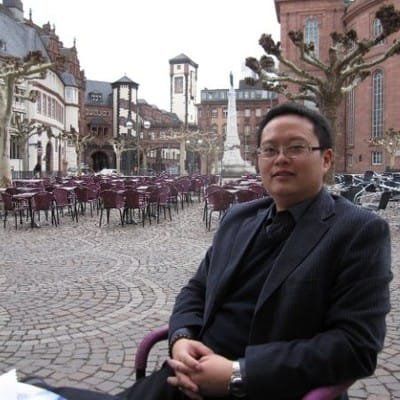
At XDS, as the leading and marketing person, I have driven many initiatives by leveraging NLP in sentiment analysis, brand monitoring, and trend detection. This will help the brands always stay tuned to the needs of customers in real time and form the basis of valid, data-driven decisions.
Customer Sentiment Analysis: Converting Feedback to Action
One of the recent projects at XDS dealt with e-commerce product review analysis in order to establish common pain points related to product usability.
Categorizing sentiment into themes pinpointed the areas of improvement that had an immediate impact on product design and customer service, which influenced a 25% uptick in customer satisfaction scores within just a few months.
Monitoring Brand Sentiment Across Platforms
Our team implemented a multi-platform sentiment solution for our company, giving us a holistic view of how our brand was perceived.
It provides early detection of any shifts in customer attitude, helping the brand to mitigate the negative sentiment before it actually impacts the broader reputation of the brand.
Trend Identification:Detecting Key Themes and Shifts
At XDS, we resorted to NLP at monitoring keywords related to sustainability and found an upward graph in eco-friendly packaging. This insight drove our client’s product and marketing strategy to better resonate with customer values and drive up engagement by 21.24%.
Real-Time Insights
One of the most significant advantages of AI-driven analytics is the ability to provide real-time insights. Traditional market research methods often resulted in outdated data by the time analysis was complete. With AI, businesses can access up-to-date information, allowing for quick decision-making.
Real-time insights are crucial in today’s dynamic market environment. Companies can respond promptly to market changes, customer feedback, or emerging trends. This agility gives businesses a competitive edge, enabling them to stay relevant and responsive.
The Impact of AI on Competitive Analysis
Understanding your competition is a key aspect of market research. AI-driven analytics offers powerful tools to analyze competitors effectively. By leveraging AI, businesses can gain deeper insights into their competitors’ strategies, strengths, and weaknesses.
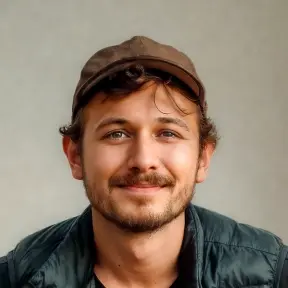
Drawing from my experience in SaaS and product development, including time as a Tech Lead at Squarespace, I’ve seen how AI-driven tools are transforming competitive analysis, enabling smaller companies like ours to benchmark and spot opportunities in a crowded market. I’d be glad to share some insights on how AI is redefining competitive intelligence and benchmarking.
When it comes to leveraging AI for competitive analysis and benchmarking, I think the most valuable takeaway is that AI isn’t simply tracking competitors—it’s actively helping businesses uncover and create their own competitive advantages.
1. How AI Tools Analyze Competitor Data and Help Businesses Benchmark
For me, the real power of AI lies in pattern detection and predictive insights that go beyond traditional benchmarking. Instead of just looking at competitor metrics like traffic or social engagement, AI allows us to understand shifts in consumer behavior in real time.
For example, at Liinks, we use AI tools to analyze where users are gravitating across various link-in-bio platforms—such as which features drive the most engagement. But the insight doesn’t stop at what competitors are doing; AI helps us see why users engage with certain features, allowing us to position ourselves to meet evolving needs, not just to copy the competition.
One practical use we’ve found is in contextual traffic analysis. Rather than only comparing visitor counts, AI enables us to break down where these visitors come from, how they engage with specific features, and even why they might drop off.
It’s incredibly powerful because it’s not just data—it’s direction. This intelligence enables us to adjust our approach based on behavioral patterns, keeping us agile and relevant.
2. How Businesses Use These Insights to Identify Market Gaps
In my view, AI’s real advantage in competitive analysis isn’t about playing catch-up with competitors; it’s about spotting the spaces competitors overlook. Market gaps are easier to spot when AI pulls together consumer sentiment, usage patterns, and feature adoption rates across platforms.
At Liinks, we’ve seen how AI-driven insights can reveal that a competitor’s highly promoted feature isn’t driving real engagement. This allows us to make more targeted product decisions, knowing that simply following the competition might not always be in our users’ best interests.
For example, we recently identified a gap in how users wanted faster customization options but weren’t getting them from major players in the market. Rather than investing in just one more customization tool, AI helped us pinpoint precisely which design elements users struggled with.
So, we developed an easy-to-use, modular design tool that allowed users to personalize without the complexity. This targeted innovation came directly from AI’s ability to provide deeper, contextual insights rather than surface-level metrics.
3. Practical Example of Leveraging AI for Competitive Advantage
One of the most impactful applications we’ve found is in real-time competitor pricing analysis. Through AI, we monitor shifts in competitor pricing models, discounts, and feature bundles.
This allows us to strategically align our pricing without engaging in a “race to the bottom.” Rather than simply reacting to competitor moves, AI lets us see potential price sensitivity in real time, enabling us to maintain value and respond with offers that resonate with our specific user base.
Another area AI has transformed is in user feedback analysis across platforms. By scanning reviews and comments on competing products, AI reveals pain points that users are openly discussing but which haven’t yet been addressed.
This insight allows us to fill those gaps and preemptively address concerns before they become challenges for our users. It’s not just about tracking; it’s about predictive problem-solving that gives us a stronger foothold in the market.
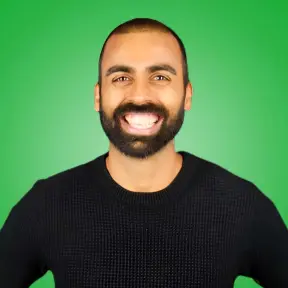
I’d like to introduce Deepak Shukla, CEO of Pearl Lemon AI, who can share expert insights into how AI is transforming competitive analysis and benchmarking in market research.
With Pearl Lemon AI’s focus on using advanced analytics to decode complex market landscapes, Deepak has seen firsthand how AI can unearth competitor data like website traffic trends, social media engagement rates and pricing adjustments to highlight crucial industry patterns and competitive gaps.
Deepak can provide real-world examples illustrating how businesses are leveraging AI insights to understand market positioning more deeply, identifying areas where competitors excel or fall short.
Through his work, he’s seen how the power of AI-driven intelligence allows companies not only to track performance metrics but to also refine strategies dynamically.
By automating the process, AI enables companies to stay agile, adjusting to shifts in demand and market behaviour instantly.
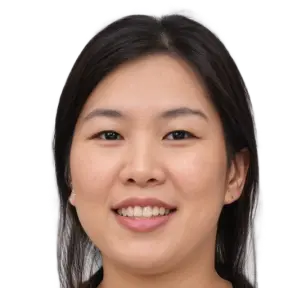
Managing Dgtl Infra’s market positioning uncovered surprising competitor patterns through AI analysis.
Our machine learning tools processed 18 months of competitor pricing data, revealing a 23% market gap in mid-tier storage solutions that our rivals missed.
When we analyzed 50,000 customer support tickets across our industry, AI flagged that competitors took 4.2 hours longer to resolve technical issues during off-peak hours.
This insight led us to launch 24/7 dedicated support, capturing 15% market share from larger providers.
Most valuable finding: AI analysis of customer migration patterns showed enterprises switch providers based on AI-readiness, not just cost – we adjusted our infrastructure to support GPU workloads, growing our AI customer base 42% while competitors focused on traditional hosting.
Competitive intelligence now drives our strategy through data, not assumptions.
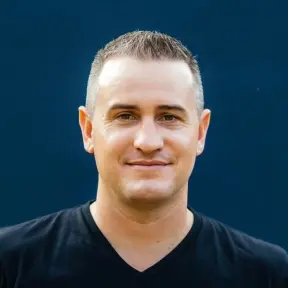
With over 14 years of marketing experience across 10 different roles, I’ve driven growth for numerous multimillion-dollar businesses, contributing to over 30+ successful projects. I hope you find my response insightful.
AI tools are revolutionizing how businesses conduct competitive analysis by enabling more granular insights into competitors’ strategies across digital platforms.
For instance, AI-powered tools can analyze competitors’ website traffic, dissecting user flow, bounce rates, and time spent on various pages to understand user engagement and pinpoint what’s drawing visitors.
Tools like Growify take it further by tracking social media engagement metrics, showing which content resonates most with audiences and when engagement peaks. One practical application is dynamic pricing.
Many AI-driven platforms now offer real-time pricing analysis, allowing businesses to adapt to competitor price shifts immediately.
For instance, we use AI to monitor pricing on similar products from our competitors and use the data gathered to adjust our prices to maintain a competitive edge without compromising margins.
By benchmarking these metrics against competitors, you can clearly understand where your business stands, uncover potential market gaps and refine strategies to differentiate your offers, leading to improved market share and customer acquisition.
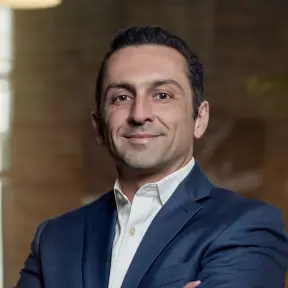
Tracking hundreds of competitor price points, businesses can refine pricing strategies: 64% of those using AI saw profits grow, per Deloitte.
Plus, AI-driven traffic analysis helps identify key competitor keywords, enhancing SEO.
For social media, AI captures engagement trends, helping companies optimize their content and benchmark against competitors.
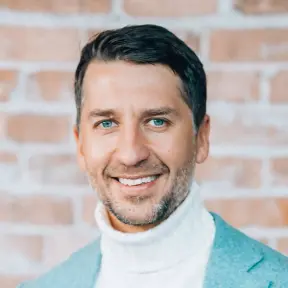
Companies using AI for SEO saw notable gains, with 54% reporting better keyword results, according to Statista.
AI also streamlines price analysis, allowing businesses to adjust pricing to match or beat competitors.
Social media insights help identify content gaps, enabling more targeted marketing that keeps businesses ahead of market trends.
Competitive Intelligence
AI tools can scan and analyze competitors’ digital footprints, including their websites, social media, and online advertisements. These tools provide valuable information on competitors’ marketing strategies, customer engagement, and product offerings. For instance, AI can track changes in competitors’ pricing, promotional activities, and customer reviews.
With this information, businesses can benchmark their performance against competitors. They can identify gaps in their strategies and find opportunities for improvement. Competitive intelligence helps businesses stay informed and make strategic decisions to outperform their rivals.
Market Trend Analysis
AI-driven analytics excels in identifying market trends. By analyzing vast amounts of data from various sources, AI can spot emerging trends before they become mainstream. This capability allows businesses to capitalize on new opportunities and stay ahead of the competition.
For example, AI can analyze social media trends to identify shifting customer preferences. If a particular product or feature is gaining popularity, businesses can adapt their offerings to meet this demand. Staying updated with market trends ensures that companies remain relevant and innovative.
Sentiment Analysis of Competitors
Sentiment analysis is not limited to understanding customer opinions about your brand. AI can also analyze sentiment around competitors. By examining customer reviews, social media mentions, and forum discussions, AI can gauge public perception of competitors’ products and services.
This analysis helps businesses understand how competitors are perceived in the market. If competitors are receiving negative feedback, there may be an opportunity to attract dissatisfied customers. Conversely, understanding positive feedback can highlight areas where competitors excel, providing insights for improvement.
Identifying Market Gaps
AI-driven analytics can help identify gaps in the market that competitors may have overlooked. By analyzing customer feedback and market trends, AI can uncover unmet needs and demands. Businesses can then develop products or services to fill these gaps, gaining a competitive advantage.
For instance, if AI analysis reveals a high demand for a feature that no competitor offers, businesses can prioritize its development. Identifying and addressing market gaps allows companies to differentiate themselves and attract new customers.
Competitor Benchmarking
Benchmarking against competitors is essential for continuous improvement. AI-driven analytics can provide detailed reports comparing various performance metrics with competitors. These metrics may include website traffic, social media engagement, customer sentiment, and product performance.
With this information, businesses can assess their strengths and weaknesses relative to competitors. Benchmarking helps in setting realistic goals and strategies for growth. By continuously monitoring competitors’ performance, businesses can stay competitive and agile.
AI in Consumer Behavior Analysis
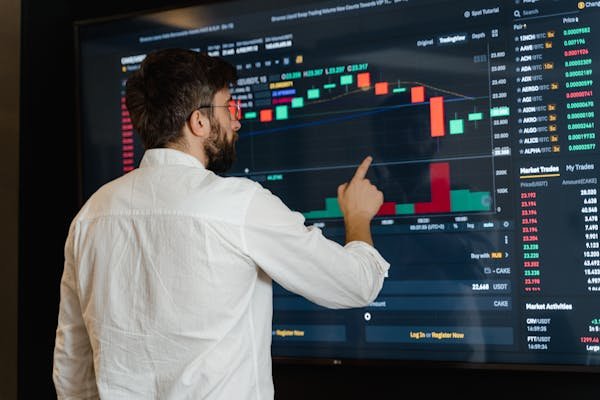
Understanding consumer behavior is crucial for creating effective marketing strategies. AI-driven analytics provides deep insights into how customers think, feel, and act. These insights enable businesses to tailor their marketing efforts to meet customer needs and preferences.
Personalization at Scale
AI allows for personalization at a scale that was previously unimaginable. By analyzing customer data, AI can create personalized experiences for each individual. This personalization can extend to product recommendations, marketing messages, and customer support interactions.
For example, e-commerce platforms use AI to recommend products based on past purchases and browsing behavior. Personalized recommendations increase the likelihood of conversion and enhance customer satisfaction. Similarly, personalized marketing messages resonate more with customers, leading to higher engagement rates.
Customer Journey Mapping
AI-driven analytics can map the entire customer journey, from awareness to purchase and beyond. By analyzing data from various touchpoints, AI can understand how customers interact with a brand at each stage of the journey.
This understanding allows businesses to optimize the customer experience. For instance, if AI identifies a drop-off point where customers abandon their carts, businesses can implement strategies to address this issue. Improving the customer journey leads to higher conversion rates and better customer retention.
Predicting Customer Behavior
Predictive analytics plays a significant role in understanding and anticipating customer behavior. AI algorithms can analyze historical data to predict future actions. This capability is invaluable for businesses looking to stay ahead of customer needs.
For instance, AI can predict when a customer is likely to make a repeat purchase. Businesses can then send timely reminders or special offers to encourage the purchase. Predicting customer behavior helps in creating proactive marketing strategies that drive sales and loyalty.
Sentiment Analysis of Customer Feedback
Customer feedback is a goldmine of information. AI-driven sentiment analysis can process large volumes of feedback to extract valuable insights. By understanding customer sentiment, businesses can identify areas for improvement and areas where they excel.
For example, if sentiment analysis reveals recurring complaints about a specific product feature, businesses can prioritize fixing this issue. Conversely, positive feedback can highlight strengths to emphasize in marketing campaigns. Understanding customer sentiment leads to better products and services.
Behavioral Segmentation
AI can segment customers based on their behavior, such as purchasing patterns, browsing habits, and engagement levels. Behavioral segmentation allows businesses to target specific customer groups with tailored marketing strategies.
For instance, businesses can identify high-value customers who frequently make purchases and engage with the brand. These customers can be targeted with exclusive offers and loyalty programs. Behavioral segmentation ensures that marketing efforts are focused on the most valuable customers.
The Role of AI in Qualitative Research
While quantitative research provides numerical insights, qualitative research delves into the reasons behind those numbers. AI-driven analytics is transforming qualitative research by making it more efficient and comprehensive.
Automated Transcription and Analysis
Traditionally, qualitative research involved manually transcribing interviews and focus group discussions, which was time-consuming. AI tools can now automate this process. Natural Language Processing (NLP) algorithms can transcribe audio recordings quickly and accurately.
Beyond transcription, AI can analyze the content to identify common themes and sentiments. For instance, AI can highlight frequently mentioned topics or emotions, providing a clear picture of the participants’ thoughts and feelings. This automated analysis saves time and ensures more objective results.
Enhanced Text Analysis
AI-driven text analysis tools can process vast amounts of textual data from various sources, including surveys, social media, and customer reviews. These tools can identify patterns, trends, and sentiments that might be missed by human analysis.
For example, AI can analyze thousands of open-ended survey responses to identify common themes. This analysis provides deeper insights into customer opinions and experiences. Enhanced text analysis allows businesses to understand their customers better and make informed decisions.
Sentiment and Emotion Detection
Understanding emotions is a critical aspect of qualitative research. AI tools equipped with sentiment analysis can detect not just whether feedback is positive or negative, but also the intensity of emotions involved. These tools can differentiate between feelings like happiness, frustration, or satisfaction.
For instance, in customer support, AI can analyze interactions to identify emotions expressed by customers. If a significant number of customers express frustration, businesses can investigate and address the root causes. Detecting emotions helps in creating a more empathetic and responsive customer experience.
Virtual Focus Groups
AI is enabling the rise of virtual focus groups. These online discussions are facilitated by AI tools that can moderate and analyze conversations in real-time. Virtual focus groups are more convenient and cost-effective than traditional ones, allowing participation from a diverse range of individuals.
AI moderators can ask relevant questions based on the ongoing discussion, ensuring that valuable insights are captured. Additionally, AI can analyze the conversation to identify key themes and sentiments. Virtual focus groups provide a flexible and efficient way to gather qualitative data.
Image and Video Analysis
Qualitative research is not limited to text; images and videos also provide valuable insights. AI-driven image and video analysis tools can process visual data to extract meaningful information. These tools can recognize objects, scenes, and even emotions depicted in images and videos.
For example, AI can analyze customer-submitted photos to understand how products are used in real-life settings. This analysis can reveal insights into product usability and customer satisfaction. Similarly, video analysis can provide deeper insights into customer behavior and preferences.
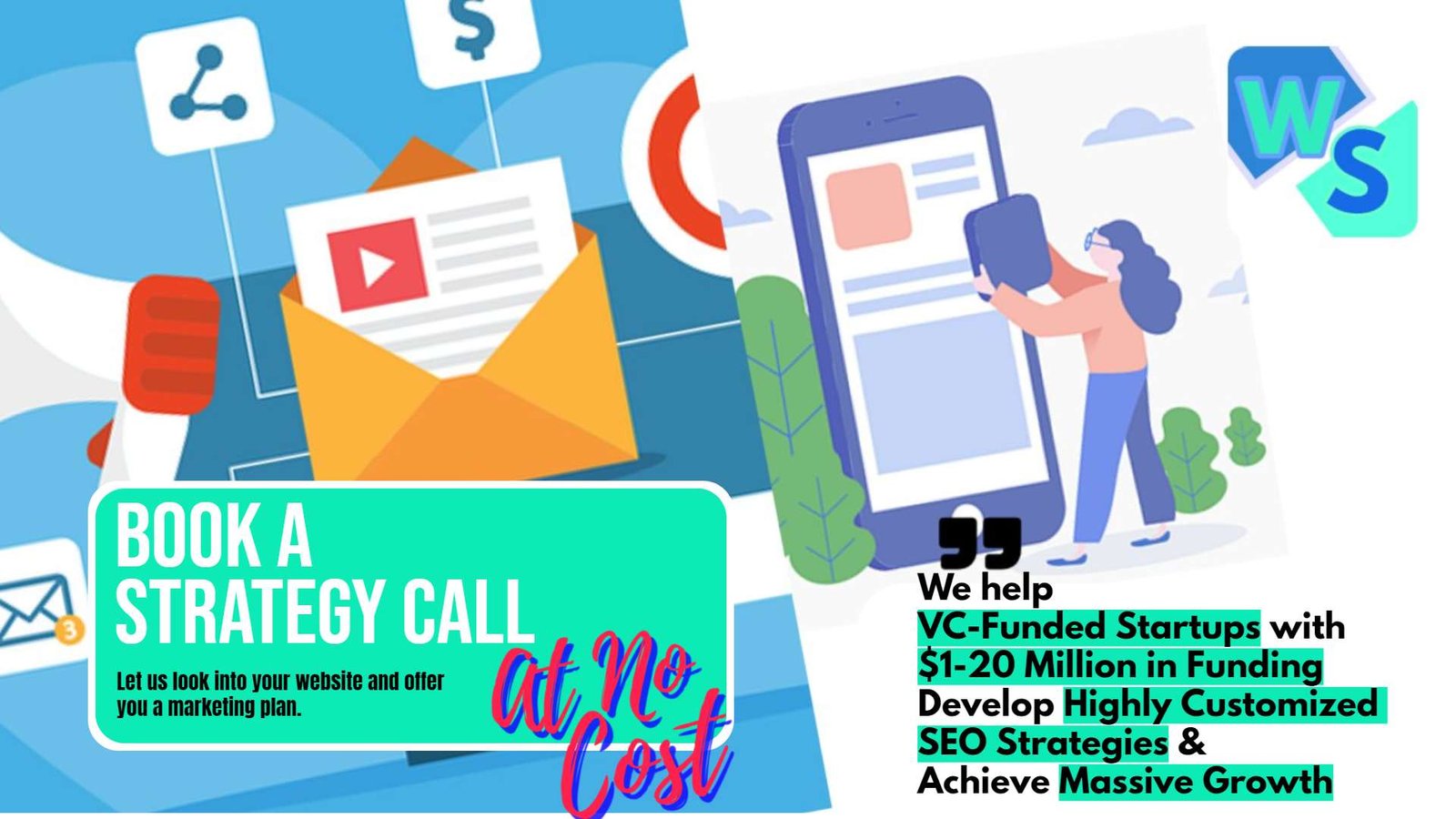
Related: Check out our free tools:
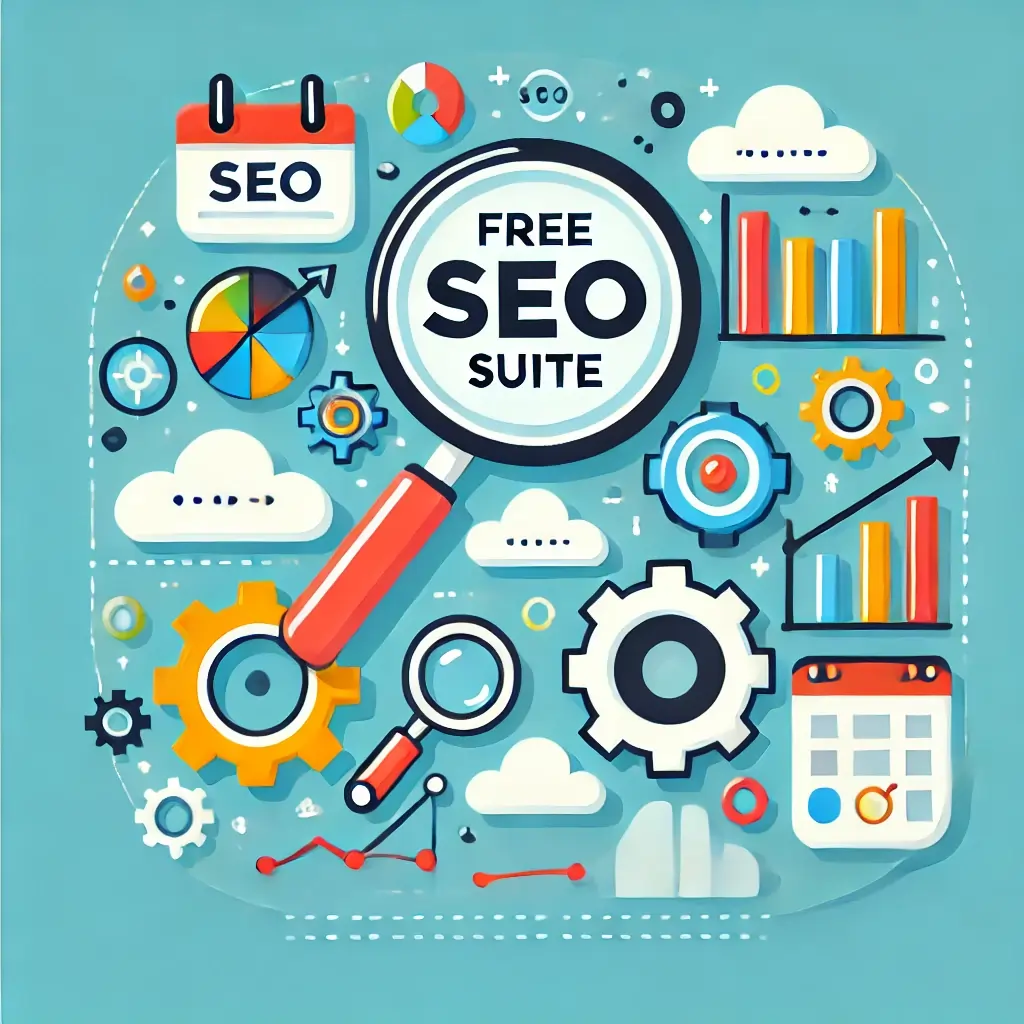
Ethical Considerations in AI-Driven Market Research
While AI offers numerous benefits, it also raises ethical considerations that businesses must address. Ensuring ethical use of AI-driven analytics is crucial for maintaining customer trust and avoiding potential legal issues.
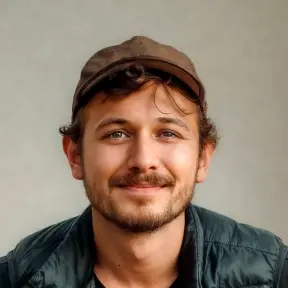
At Liinks, we’ve focused on user-centered design, so I understand the importance of ethical AI, especially in data-sensitive areas like market research.
I’d be glad to share insights on how companies can prioritize ethics, privacy, and transparency when using AI for data collection and analysis.
1. What measures can businesses take to ensure ethical AI usage in data collection and analysis?
For me, ethical AI starts with prioritizing transparency and active accountability. It’s not just about compliance—it’s about building trust with users who may not fully understand how their data is being processed.
One measure I’d recommend is establishing clear guidelines for data usage disclosures that outline, in simple terms, how the data will be used, stored, and analyzed. This goes beyond generic privacy policies and instead focuses on concrete, accessible information that users can understand and trust.
Another essential measure is regular audits of AI algorithms to identify and mitigate potential biases. In my experience, without these checks, algorithms can unintentionally reinforce existing biases in datasets, skewing results.
Bias can be addressed by diversifying training data sources and implementing controls to assess if certain demographic groups are disproportionately affected by AI outputs.
This level of attention ensures that companies are not only aware of ethical pitfalls but actively working to prevent them.
2. How can businesses enhance data privacy with AI?
To protect data privacy, I believe in anonymizing data whenever possible. By removing identifying features in datasets, companies can still gain valuable insights while safeguarding individual privacy.
It’s a best practice I advocate for at Liinks—ensuring user data is anonymized before being used in analytics.
Businesses should also adopt differential privacy techniques that add a layer of noise to data, protecting individual entries without compromising the quality of insights gathered.
3. Examples of companies leading with ethical AI practices
One example I admire is Apple’s commitment to differential privacy in their data analysis. They maintain high standards for privacy while extracting useful insights, which has set a benchmark for privacy-focused AI.
Microsoft is another leader, especially with its efforts to establish robust frameworks for bias detection and mitigation in AI models.
They’ve taken proactive steps to educate users on how their data is used and implemented checks to identify potential algorithmic biases.
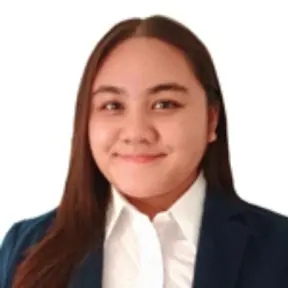
As AI changes how we do market research, it’s key that our sense of ethics grows alongside it.
Companies should focus on building systems with privacy and openness built in from the very beginning. They should openly disclose how data is collected, who has access, and why it’s used.
Reducing bias in AI models requires continuous scrutiny and diverse data sources to reflect real-world diversity—not tech-world echo chambers.
Leaders like IBM and Microsoft are setting standards by investing in bias audits and responsible AI toolkits.
Ethical AI is not just a checkbox; it’s a trust-building strategy that will decide which brands survive tomorrow’s consumer scrutiny.
Ignoring this risks both reputational and regulatory backlash.
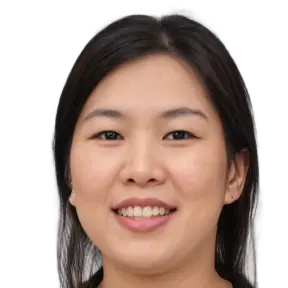
Implementing AI across Dgtl Infra’s market research revealed critical ethical checkpoints.
Our initial customer behavior analysis flagged concerning patterns – AI models showed 34% bias toward larger enterprise clients simply due to more available data.
We established a three-tier verification system: any AI insights requiring personal data must show clear consent trails, automated decisions undergo monthly bias testing, and all AI-driven conclusions face human expert review.
These guardrails caught a significant bias last quarter – our AI overlooked smaller customers’ growth potential by 28% due to historical data skew.
After retraining models with balanced datasets, we saw a 45% improvement in prediction accuracy across all customer segments.
Most crucial learning: transparency with customers about AI usage increased trust scores by 62%, while opt-out rates remained below 3%.
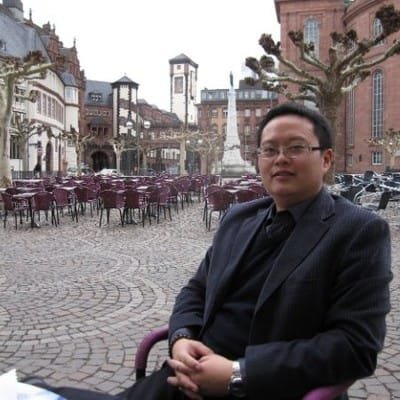
At XDS, we believe that data ethics must focus on transparency, privacy, and bias reduction in order to create mechanisms that align AI with ethical standards.
Making sure data collection and analysis with AI involves ethical practices builds long-term trust in the veracity of insights today through rich data environments.
Data Privacy: The Foundation of Ethical AI
To that end, we have implemented very strict data governance policies whereby, for any given project, we start off with an audit to validate if all sources of data are compliant with any of the regulations applicable in your region, such as GDPR and CCPA.
For example, in doing sentiment analysis for any client, the individual identities are kept anonymous by using aggregate data only. This also reduces privacy risks while still allowing many valuable insights to be uncovered.
Minimizing Data Collection and Analytical Bias
We utilized a multi-step validation process to check the demographic representation in the current study of consumer purchasing trends. In this way, the findings indicate a wide perspective representative of a broad section.
Transparency in AI Models
XDS works on the concept of explainable AI, where it is crystal clear how the data is being used and analyzed.
When we present insights to our clients, a comprehensive report explaining every minute detail of data processing and analysis is also provided.
This has helped us maintain the aspect of trust wherein the client knows how exactly the insight has been derived, hence reducing the ambiguity amount AI processes.
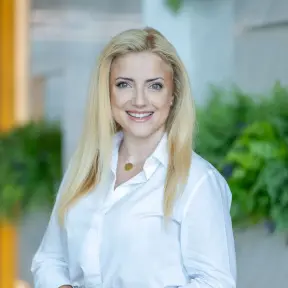
For businesses venturing into AI for market research, the ethical compass points to transparency, privacy, and the human factor.
Transparency isn’t just a box to tick; it means clear guidelines for AI’s use in data collection and openly addressing the source of data and methods.
Privacy hinges on compliance—businesses must select tools with clear safeguards like HIPAA alignment for healthcare contexts.
And bias? Companies should adopt model cards, those snapshots of data diversity, so they’re not just borrowing mainstream narratives that don’t reflect their audience.
An example of leading this way is firms adjusting their AI use by continuously training their algorithms with diverse datasets that represent real-world demographics, enhancing both equity and inclusivity.
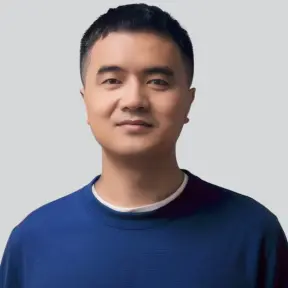
Focusing on data privacy and security is an essential first step that businesses using AI tools can take to ensure the data collection and analysis process is ethical.
Key steps businesses should take are ensuring data anonymization, especially in datasets used for machine learning.
This process ensures that user identities are pseudonymized or anonymized to protect their privacy and prevent bias when training ML tools.
Secure storage of collected data is crucial to its protection. I recommend businesses implement robust cybersecurity measures, such as role-based access and multi-factor authentication, to prevent unauthorized access to collected data.
Additionally, regular compliance checks should be conducted to ensure compliance with data protection standards such as GDPR.
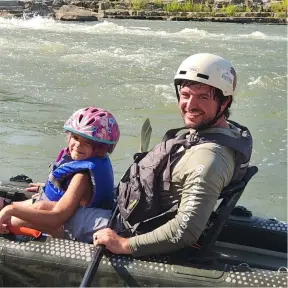
With AI becoming so central to market research, the need for ethical practices is front and center.
As the Marketing Manager at Anglers, I work daily with data and AI tools that need to be used responsibly. From our perspective, ethical AI involves three main areas: data privacy, minimizing bias, and transparency.
Data privacy needs to be top priority. Companies should collect data with full consent and keep it secure an approach that companies like Apple have championed.
Apple, for instance, emphasizes strong data encryption and user control, which is something we’re mirroring at Anglers to ensure our customers feel secure sharing information.
To reduce bias in AI-driven insights, it’s essential to have diverse datasets and review the outputs regularly. Using a broad set of data sources helps avoid skewed results, which is especially critical when analyzing customer behaviors.
At Anglers, we’ve implemented regular AI audits to ensure that our data captures varied perspectives and remains as balanced as possible. IBM, with its emphasis on AI ethics boards and bias-detection software, is setting a strong industry standard here.
Lastly, transparency can’t be overstated. Being upfront with users about how their data is used and how AI processes their information fosters trust.
Microsoft’s AI ethics frameworks and transparency in algorithm usage are great examples.
At Anglers, we aim to provide the same level of openness, helping our users understand what AI means for them.
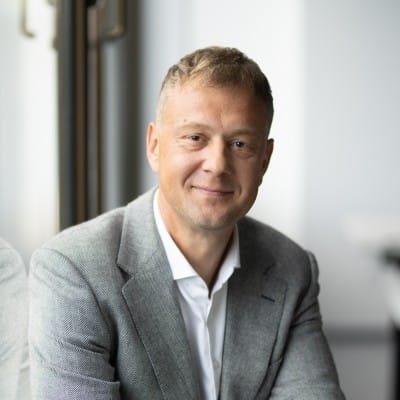
As the CEO of Ondato, where we navigate the intersection of AI and compliance in financial services, I can share insights on ethical AI implementation in market research.
One crucial ethical consideration we’ve learned is the importance of “Transparent Data Usage.” In market research, AI can process vast amounts of data, but transparency about data collection and usage is non-negotiable.
Companies must clearly communicate how AI analyzes customer data and what safeguards are in place.
For example, when we analyze market trends in compliance technology, we ensure all data is anonymized and aggregated before AI processing. We also implement strict access controls and regular audits to maintain data integrity.
“In the age of AI, ethical market research isn’t just about collecting data – it’s about respecting privacy, reducing bias, and maintaining transparency.
The most valuable insights come from responsible data practices that build trust with your audience.”
Data Privacy
One of the primary concerns with AI-driven analytics is data privacy. Collecting and analyzing large amounts of data can lead to privacy breaches if not handled correctly. Businesses must ensure that they comply with data protection regulations and respect customer privacy.
For example, companies should obtain explicit consent from customers before collecting their data. Additionally, they should anonymize data to protect individual identities. Implementing robust data security measures is essential to prevent unauthorized access to sensitive information.
Bias and Fairness
AI algorithms can sometimes exhibit bias, leading to unfair outcomes. This bias can stem from the data used to train the algorithms. If the training data is biased, the AI system will likely produce biased results. Ensuring fairness in AI-driven analytics is crucial to avoid discrimination.
Businesses should regularly audit their AI systems to identify and mitigate any biases. Using diverse and representative datasets for training AI can help reduce bias. Additionally, involving diverse teams in the development and deployment of AI systems can promote fairness.
Transparency
Transparency is vital for building trust in AI-driven analytics. Businesses should be transparent about how they collect, analyze, and use data. Customers have the right to know how their data is being used and the impact it has on them.
For instance, companies should provide clear explanations of their AI algorithms and decision-making processes. This transparency helps customers understand and trust the technology. Additionally, businesses should be open about any limitations or uncertainties in their AI systems.
Accountability
Accountability is essential in ensuring ethical use of AI. Businesses must take responsibility for the actions and decisions made by their AI systems. This accountability involves monitoring AI performance and addressing any issues that arise.
For example, if an AI system makes a wrong decision, businesses should have mechanisms in place to rectify the situation. Regularly reviewing and updating AI systems ensures that they continue to operate ethically and effectively. Accountability promotes responsible use of AI in market research.
Informed Consent
Informed consent is a fundamental ethical principle. Businesses should ensure that customers are fully aware of how their data will be used before they provide it. This consent should be obtained through clear and straightforward communication.
For instance, companies can use simple language to explain their data collection practices. They should also provide options for customers to opt out if they are not comfortable. Ensuring informed consent respects customer autonomy and builds trust.
Practical Applications of AI-Driven Market Research
AI-driven analytics is not just a futuristic concept; it is already being applied in various industries to drive business success. Here are some practical applications of AI in market research that demonstrate its versatility and effectiveness.
Retail and E-Commerce
In the retail and e-commerce sectors, AI-driven market research is used to enhance customer experiences and optimize operations. AI tools analyze customer behavior to personalize shopping experiences, recommend products, and predict trends.
For instance, AI algorithms can analyze past purchase data to recommend products that customers are likely to buy. These personalized recommendations increase the chances of conversion and enhance customer satisfaction. Additionally, AI can predict inventory needs, helping retailers manage stock levels efficiently and avoid overstocking or stockouts.
Healthcare
In healthcare, AI-driven analytics is transforming how providers understand patient needs and improve services. AI tools analyze patient data to identify trends and predict future healthcare demands.
For example, AI can analyze electronic health records to identify patterns in patient visits and treatments. This analysis helps healthcare providers anticipate future demand for services and allocate resources effectively. AI-driven market research can also identify gaps in healthcare services, guiding providers in improving patient care and expanding services where needed.
Financial Services
The financial services industry leverages AI-driven analytics to understand customer behavior, manage risks, and develop new products. AI tools analyze transaction data, customer feedback, and market trends to provide valuable insights.
For instance, banks can use AI to analyze transaction data and identify patterns that indicate potential fraud. This proactive approach helps in preventing fraudulent activities and protecting customers. Additionally, AI-driven market research can identify customer needs for new financial products, guiding the development of innovative solutions.
Consumer Goods
Companies in the consumer goods sector use AI-driven analytics to understand market trends, customer preferences, and product performance. AI tools analyze data from various sources, including social media, customer reviews, and sales data.
For example, AI can analyze social media conversations to identify emerging trends in consumer preferences. This insight helps companies adjust their product offerings and marketing strategies to align with current trends. Additionally, AI can analyze customer feedback to identify common issues with products, guiding improvements and innovations.
Travel and Hospitality
The travel and hospitality industry uses AI-driven market research to enhance customer experiences and optimize services. AI tools analyze customer data to personalize travel recommendations, predict travel trends, and improve service delivery.
For instance, AI can analyze booking patterns to predict peak travel times and adjust pricing strategies accordingly. This predictive capability helps businesses maximize revenue while offering competitive prices. Additionally, AI can personalize travel recommendations based on past travel history and preferences, enhancing customer satisfaction.
Media and Entertainment
In the media and entertainment industry, AI-driven analytics is used to understand audience preferences, predict trends, and personalize content. AI tools analyze data from various sources, including streaming platforms, social media, and audience feedback.
For example, AI can analyze viewing patterns on streaming platforms to recommend content that viewers are likely to enjoy. These personalized recommendations keep audiences engaged and increase subscription rates. Additionally, AI-driven market research can identify emerging trends in content preferences, guiding the development of new shows and movies.
Future Trends in AI-Driven Market Research
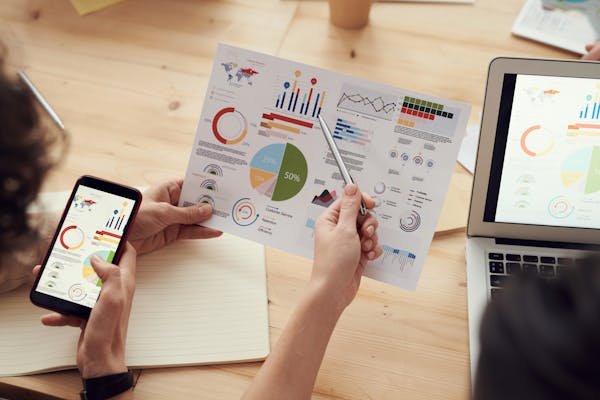
AI-driven market research is continuously evolving, with new trends emerging as technology advances. Here are some future trends that are likely to shape the landscape of market research.
Integration of Augmented Reality (AR) and Virtual Reality (VR)
AR and VR technologies are becoming more prevalent in market research. These technologies offer immersive experiences that can enhance customer engagement and provide deeper insights into consumer behavior.
For example, businesses can use VR to create virtual shopping experiences, allowing customers to explore products in a realistic setting. This immersive experience provides valuable insights into how customers interact with products. Similarly, AR can enhance product demonstrations, helping customers visualize how products fit into their lives.
Advanced Predictive Analytics
As AI technology advances, predictive analytics will become even more sophisticated. AI algorithms will analyze larger and more complex datasets, providing more accurate predictions of market trends and customer behavior.
For instance, advanced predictive analytics can anticipate shifts in consumer preferences, allowing businesses to stay ahead of trends. This capability will enable companies to proactively adjust their strategies, ensuring they remain competitive and relevant.
Greater Emphasis on Ethical AI
As AI becomes more integrated into market research, there will be a greater emphasis on ethical AI practices. Businesses will need to ensure that their AI systems are transparent, fair, and accountable.
For example, companies will implement robust measures to ensure data privacy and prevent bias in AI algorithms. There will also be a focus on transparency, with businesses providing clear explanations of how AI systems work and how data is used. Ethical AI practices will build trust and ensure the responsible use of technology.
Real-Time Data Analysis
The demand for real-time insights will continue to grow, driving advancements in real-time data analysis. AI-driven analytics will provide up-to-the-minute information, allowing businesses to make quick and informed decisions.
For instance, real-time sentiment analysis can gauge public reaction to a new product launch, allowing businesses to respond promptly. This capability will enable companies to stay agile and responsive in a fast-paced market environment.
Personalization 2.0
Personalization will reach new heights with the advancement of AI technology. Businesses will leverage AI to provide even more tailored experiences for customers, enhancing engagement and satisfaction.
For example, AI will analyze a broader range of data points to create hyper-personalized marketing messages. This level of personalization will make customers feel valued and understood, fostering loyalty and driving conversions.
Conclusion
AI-driven analytics is revolutionizing market research, offering unprecedented insights and capabilities.
From enhancing data collection to providing real-time insights, AI is transforming how businesses understand and respond to market dynamics. This technology enables more precise customer segmentation, predictive analytics, and a deeper understanding of consumer behavior, leading to more informed decision-making.
Read Next:
- How to Leverage AI for Content Ideas
- AI for Creating Viral Blog Posts
- AI Writing Software for Effective Content
- Crafting Blog Posts with AI Assistance
- AI in Copywriting: Practical Tips and Tricks
Comments are closed.