Hey there! Managing risk is a crucial part of any business, but it can often feel overwhelming. With so many variables and potential pitfalls, knowing where to focus your efforts can be challenging. That’s where AI-powered predictive analytics come in. These advanced tools can help you identify and mitigate risks before they become serious problems. By leveraging AI, you can turn risk management from a daunting task into a strategic advantage. In this article, we’ll explore how AI-powered predictive analytics can revolutionize risk management for your business. Ready to dive in? Let’s get started!
Understanding AI-Powered Predictive Analytics
The Fundamentals of Predictive Analytics
Predictive analytics involves the use of statistical algorithms, machine learning techniques, and data mining to analyze historical data and predict future events. It enables businesses to make informed decisions by identifying patterns and trends that are not immediately apparent.
For startup founders, mastering the fundamentals of predictive analytics can be a game-changer, providing the insights needed to mitigate risks and capitalize on opportunities.
To begin, it’s essential to understand the types of data used in predictive analytics. Structured data, such as sales figures and financial records, is organized and easily searchable.
Unstructured data, such as social media posts and customer feedback, is more complex but can provide valuable insights when analyzed correctly. Combining these data types allows for a more comprehensive analysis, leading to better predictions.
How AI Enhances Predictive Analytics
Artificial Intelligence (AI) significantly enhances predictive analytics by automating data analysis and improving the accuracy of predictions. Traditional predictive models rely on predefined algorithms and may struggle with large and complex datasets. AI, however, can process vast amounts of data quickly and adaptively, learning from new data in real-time.
For startup founders, this means that AI can identify subtle patterns and relationships in data that humans might miss. For example, AI can analyze customer behavior to predict future purchasing trends or identify potential market shifts before they happen. By leveraging AI, startups can make proactive decisions that position them ahead of the competition.
Strategic Implementation of AI-Powered Predictive Analytics
Implementing AI-powered predictive analytics in a startup requires a strategic approach. Begin by clearly defining the business objectives you want to achieve with predictive analytics. This could include reducing operational risks, improving customer retention, or optimizing supply chain management. Having clear goals will guide your data collection and analysis efforts.
Next, gather and prepare your data. High-quality data is crucial for accurate predictions. Ensure that your data is clean, relevant, and comprehensive. This might involve integrating data from various sources, such as CRM systems, financial records, and social media channels. Use data preprocessing techniques to handle missing values, outliers, and inconsistencies, ensuring that your dataset is robust and reliable.
Once your data is ready, select the right AI tools and technologies. There are various AI platforms available, each with unique capabilities. Choose a platform that aligns with your specific needs and integrates seamlessly with your existing systems. Tools like TensorFlow, IBM Watson, and Microsoft Azure AI offer powerful predictive analytics capabilities that can be tailored to your business requirements.
Building and Validating AI Models
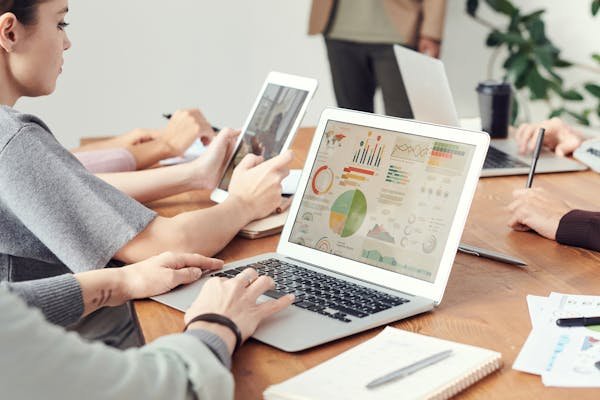
Building effective AI models is a critical step in implementing predictive analytics. Start by selecting the appropriate machine learning algorithms based on your data and business objectives. Common algorithms used in predictive analytics include linear regression, decision trees, and neural networks. Each algorithm has its strengths and is suited to different types of predictions.
Train your AI models using historical data, allowing them to learn and identify patterns. It’s essential to validate your models to ensure their accuracy and reliability. Use techniques such as cross-validation and train-test splits to assess the performance of your models. Regularly update and retrain your models with new data to keep them accurate and relevant.
For startup founders, collaborating with data scientists and AI experts can be highly beneficial during this phase. These professionals can provide valuable insights and guidance, helping you build robust models that deliver actionable predictions.
Interpreting AI-Driven Insights
Interpreting the insights generated by AI-powered predictive analytics is crucial for making informed decisions. AI can provide a wealth of data, but understanding how to apply these insights strategically is what drives business success. Focus on identifying key patterns and trends that align with your business objectives.
For example, if AI predicts a potential increase in customer churn, analyze the factors contributing to this trend. This might involve examining customer feedback, product usage patterns, or market conditions. Use these insights to develop targeted retention strategies, such as personalized marketing campaigns or product improvements.
Integrating Predictive Analytics into Business Processes
Integrating predictive analytics into your business processes ensures that AI-driven insights are actionable and impactful. Start by embedding predictive analytics into your decision-making framework. This involves incorporating AI predictions into your regular business reviews, strategy sessions, and operational planning.
For instance, use predictive analytics to inform your marketing strategies by identifying the most promising customer segments and tailoring your campaigns accordingly. In supply chain management, leverage AI predictions to optimize inventory levels and anticipate potential disruptions. By integrating predictive analytics into your core business processes, you can make data-driven decisions that enhance efficiency and drive growth.
Real-World Example: AI-Powered Risk Management in a Startup
Consider a fintech startup that uses AI-powered predictive analytics to manage financial risks. The startup integrates AI tools with its transaction data, analyzing patterns to detect potential fraud. By continuously monitoring transactions in real-time, the AI system can identify anomalies and flag suspicious activities, allowing the startup to respond promptly and prevent financial losses.
The startup also uses predictive analytics to assess credit risks. By analyzing a wide range of data points, including customer financial history, spending behavior, and social media activity, the AI system can accurately predict the likelihood of default. This enables the startup to make more informed lending decisions, reducing the risk of bad loans and improving overall financial stability.
Future-Proofing Your Predictive Analytics Strategy
To ensure the long-term success of your predictive analytics initiatives, it’s essential to future-proof your strategy. Stay informed about emerging trends and advancements in AI technology. Regularly update your AI models and tools to incorporate the latest innovations and best practices. Invest in continuous learning and development for your team to keep their skills and knowledge up-to-date.
Additionally, foster a culture of experimentation and innovation within your organization. Encourage your team to explore new applications of predictive analytics and test different approaches. By remaining agile and adaptable, you can leverage AI-powered predictive analytics to navigate uncertainties, seize opportunities, and achieve sustainable growth.
Key Benefits of AI-Powered Predictive Analytics in Risk Management
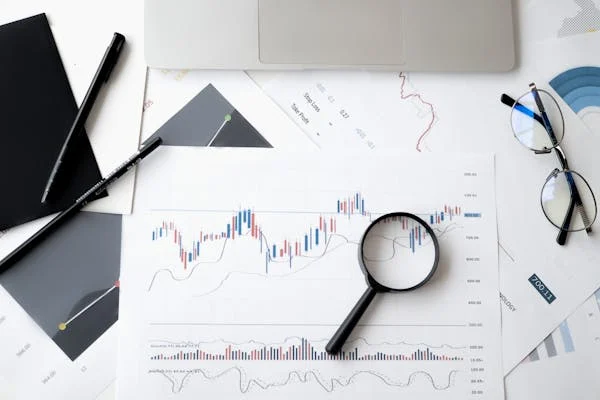
Early Detection of Risks
One of the most significant benefits of AI-powered predictive analytics is the early detection of risks. By continuously analyzing data from various sources, AI can identify potential risks before they materialize.
For example, in finance, AI can detect unusual transaction patterns that might indicate fraud. In supply chain management, it can predict disruptions due to geopolitical events, natural disasters, or supplier issues. Early detection allows businesses to take preventive measures, reducing the impact of risks.
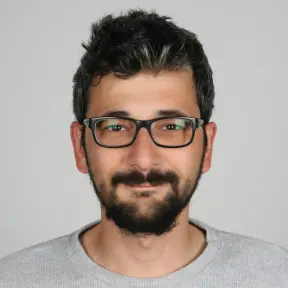
Startups in the financial sector often need to catch fraud quickly to protect their bottom line. AI can process large volumes of transaction data and pick up patterns that might be missed otherwise.
For example, I helped a fintech startup set up an AI model that looked at customer spending habits. It flagged transactions that didn’t align with typical behavior—like a user suddenly making high-value purchases from a different country or buying items that didn’t fit their past habits.
Within the first quarter, the system identified 95% of suspicious transactions in real-time, saving the team about $50,000. This kind of real-time analysis means that fraud detection isn’t just about stopping the obvious scams but catching those unusual patterns that might slip through manual review.
2. Understanding Credit Risk in Lending
For startups in lending, accurately assessing who is likely to repay a loan is important. Traditional methods like credit scores only tell part of the story, so we set up AI systems that could process a wider range of data points. For example, one project involved analyzing social media activity, payment histories, and email behaviors to create a richer picture of creditworthiness.
This approach improved the accuracy of their credit risk assessments by about 30%, allowing them to extend more loans with fewer defaults. For small startups, this accuracy can mean the difference between sustainable growth and taking on risky clients that put the business at risk.
3. AI in Cybersecurity: Finding the Invisible Threats
AI in cybersecurity is like having a guard who never sleeps. I worked with a client to deploy an AI-driven tool that monitored network traffic around the clock, analyzing millions of data points every hour. It looked for signs of unusual activity, like patterns that matched known types of cyberattacks or sudden spikes in data access.
Over the first 60 days, the system spotted 20% more threats than their previous manual methods. This early detection allowed them to close security gaps before hackers could take advantage of them. This kind of early warning system can be a game changer for a startup that might not have the budget for a large IT team.
4. Using AI for Early Economic Indicators
Another way AI has helped is by predicting economic changes that could affect a business. One startup I advised used an AI tool to keep an eye on financial news, industry changes, and what competitors were doing. This AI would alert them early when certain market signals indicate a downturn or a shift in consumer behavior.
For example, when AI detected early signs of a slowdown in a specific industry, the startup changed its investment strategy and avoided a 15% market drop that impacted its competitors. This approach saved them money and allowed them to move their resources to more stable opportunities.
A fintech startup I worked with used AI to predict which customers were likely to stop using their services. The AI looked at data like how often customers logged into their accounts, how much time they spent on the app, and which features they used less often.
With this information, the team could reach out to customers with personalized offers or support, which helped lower their churn rate by 18% in just three months. This approach to managing risks keeps customers engaged and helps prevent unexpected losses.
AI has the potential to save startups time and money by identifying risks before they become bigger issues. It can spot trends, predict outcomes, and help companies adjust their strategies before problems arise. It’s like having a crystal ball that doesn’t just predict the future but helps you prepare for it.
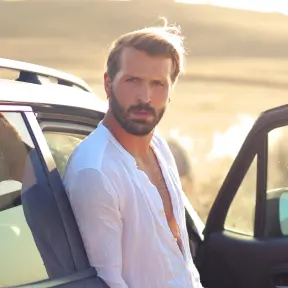
I’ve seen firsthand how AI-powered predictive analytics can be a lifeline for startups when it comes to detecting risks early. I think one of the biggest challenges startups face is the sheer speed at which risks, like fraud or credit defaults, can hit them.
In my experience, AI tools are incredibly effective at scanning data for patterns that might signal a problem before it becomes a full-blown crisis.
I’ve used AI systems to help startups predict financial risks, and what I found impressive is how AI models can analyze historical data and detect signs of fraud or credit issues even when the human eye might miss them.
I personally think the beauty of AI in this context is that it allows startups to take proactive steps. I’ve been part of situations where AI flagged high-risk transactions or unusual financial behaviors, enabling the company to act before major damage was done.
The startups I’ve worked with have benefited from using AI to run real-time monitoring, and they’ve been able to avoid potential losses by identifying threats early on.
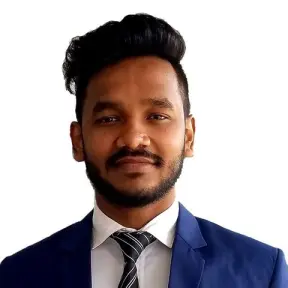
Startups can influence AI to predict and reduce risks like fraud or credit defaults by utilizing machine learning models that detect patterns in large datasets.
AI, especially with unsupervised learning, can analyze real-time transactional data to flag unusual activity, which effectively prevents fraud.
By integrating AI-driven workflow engines into payment systems, startups can identify between authorized and fake transactions, providing real-time protection.
For credit risk management, AI uses advanced algorithms to analyze large data sets quickly and accurately, helping to assess risk and predict potential losses.
This allows startups to make faster, data-driven decisions. That helps to improve accuracy and reduce risk, offering a competitive edge in managing fraud and credit defaults.
An example of AI’s impact can be seen in a big company named Stripe. This payment processing company uses AI to monitor transactions for potential fraud in real-time.
Their AI system adapts to rising threats by continuously learning from new data, allowing the company to effectively manage risks and prevent fraud for its clients.
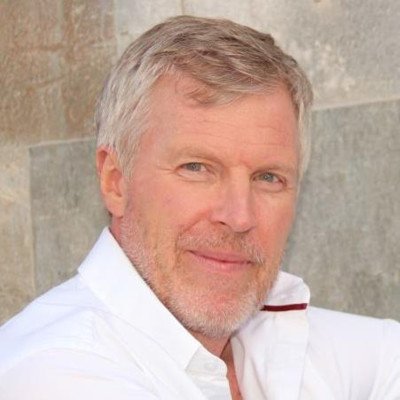
AI does the analytic work of dozens of cybersecurity analysts. It could do the work of millions with enough computing power, but that isn’t possible yet.
Basically, AI monitors the network for suspicious activity at a much larger scale, and reports it at a much faster rate than a human can.
Every startup should be using this to their advantage to stay secure without a huge security team.
AI threat detection is the most widespread use of AI in cybersecurity today because it is effective, and will continue to become more effective as it evolves.
Improved Decision Making
AI-powered predictive analytics provide actionable insights that enhance decision-making processes. By understanding potential risks and their implications, businesses can make informed decisions that minimize negative outcomes.
For instance, if predictive analytics indicate a high likelihood of a market downturn, a business can adjust its investment strategy accordingly. Similarly, if operational risks are identified, companies can optimize their processes to mitigate these risks.
Cost Efficiency
Effective risk management can lead to significant cost savings. By preventing risks or mitigating their impact, businesses can avoid costly disruptions, fines, and reputational damage. AI-powered predictive analytics help identify the most cost-effective risk management strategies.
For example, in the insurance industry, AI can predict which policies are most likely to result in claims and adjust premiums accordingly. In manufacturing, predictive maintenance can reduce downtime and repair costs.
Enhanced Regulatory Compliance
Regulatory compliance is a critical aspect of risk management, especially in highly regulated industries such as finance, healthcare, and manufacturing. AI-powered predictive analytics can help businesses stay compliant by identifying potential compliance risks and providing recommendations for addressing them.
For example, in finance, AI can monitor transactions for signs of money laundering and other illegal activities. In healthcare, it can ensure that patient data is handled in compliance with privacy regulations.
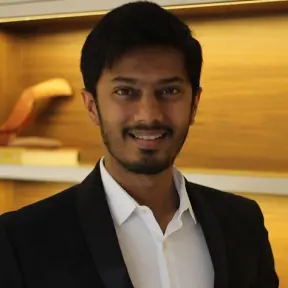
AI-powered predictive analytics is a game-changer for businesses looking to stay compliant with regulations like GDPR and CCPA.
By analyzing data patterns, AI can identify potential compliance risks before they escalate, allowing for proactive risk mitigation.
For example, AI can monitor data flows in real time, flagging unusual activities and automating reporting to ensure regulatory adherence.
In highly regulated sectors like finance and healthcare, AI systems have proven effective at predicting regulatory changes and updating compliance protocols automatically.
This minimizes manual oversight, reduces human error, and ensures compliance with evolving standards—ultimately saving businesses time and reducing operational costs.
Integrating AI into compliance workflows not only enhances regulatory alignment but also improves efficiency, allowing companies to make smarter, data-driven decisions.
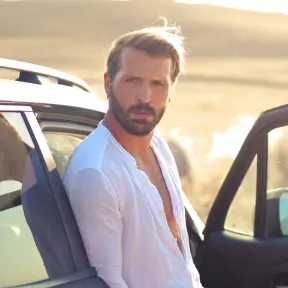
I believe AI-powered predictive analytics is a game-changer when it comes to staying compliant. In my experience, I’ve seen how AI tools can predict compliance risks by analyzing vast amounts of data in real time.
I think one of the most powerful aspects is that AI can flag unusual patterns or potential violations before they escalate.
I’ve personally worked with AI systems that monitor activities against GDPR or CCPA requirements, and they’ve helped businesses avoid hefty penalties by alerting them to risks early.
I’ve noticed that AI doesn’t just stop at identifying risks – it provides actionable insights, so compliance officers know exactly what needs attention.
For instance, I’ve used AI to automatically track data privacy regulations, and it’s amazing how quickly it can adapt to changing standards.
I also believe AI reduces human error in compliance. When I’ve relied on manual processes in the past, I’ve noticed how easy it is to overlook something.
With AI, the system works tirelessly, scanning for potential issues that we might miss, and I find that gives a lot of peace of mind in high-stakes industries like finance.
Increased Agility
In today’s fast-paced business environment, agility is essential. AI-powered predictive analytics enable businesses to respond quickly to emerging risks and opportunities. By continuously monitoring and analyzing data, AI provides real-time insights that support rapid decision-making.
This increased agility allows businesses to stay ahead of the competition and adapt to changing market conditions. For example, retailers can use AI to predict shifts in consumer behavior and adjust their inventory and marketing strategies accordingly.
Implementing AI-Powered Predictive Analytics in Risk Management
Choosing the Right AI Tools
Selecting the appropriate AI tools is the first step in implementing AI-powered predictive analytics for risk management. There are several AI platforms and software available, each with its strengths.
Some popular options include IBM Watson, Google Cloud AI, and Microsoft Azure AI. When choosing a tool, consider factors such as ease of integration with your existing systems, scalability, and the specific features that align with your business needs.
Start by identifying the key areas where AI can add value to your risk management strategy. For example, if your primary concern is cybersecurity, look for AI tools that specialize in detecting and mitigating security threats. If you’re focused on financial risks, choose platforms that excel in financial data analysis and forecasting.
Data Integration and Management
Data is the foundation of effective predictive analytics. To leverage AI in risk management, you need high-quality, comprehensive data from various sources. This might include internal data such as financial records, operational logs, and employee data, as well as external data like market trends, social media activity, and regulatory changes.
Ensure that your data is clean, accurate, and up-to-date. Implement robust data management practices to maintain data integrity and security. Integrate your AI tools with your data sources to create a unified data environment. This integration enables AI to analyze data holistically and provide more accurate risk predictions.
Building and Training AI Models
Once your data is ready, the next step is to build and train your AI models. Start by defining the specific risks you want to manage and the outcomes you aim to predict. For instance, you might want to predict credit defaults, operational disruptions, or market volatility. Use historical data to train your AI models, allowing them to learn patterns and relationships that can predict future outcomes.
Training AI models involves feeding them large amounts of data and adjusting their parameters to improve accuracy. This process may require collaboration with data scientists or AI specialists.
It’s important to regularly validate and test your models to ensure they are performing as expected. Continuously update your models with new data to keep them accurate and relevant.
Real-Time Monitoring and Alerts
One of the significant advantages of AI-powered predictive analytics is the ability to provide real-time monitoring and alerts. Set up your AI systems to continuously monitor data streams and detect anomalies or patterns that indicate potential risks. For example, in a financial context, AI can monitor transactions in real-time and alert you to suspicious activities that may indicate fraud.
Configure your AI tools to send automated alerts to relevant stakeholders when a risk is detected. These alerts should include actionable insights and recommendations for mitigating the risk. Real-time monitoring and alerts enable proactive risk management, allowing you to address issues before they escalate.
Case Studies: Success Stories with AI-Powered Risk Management
Financial Services
One notable example of AI-powered risk management in the financial sector is JPMorgan Chase. The bank uses AI to detect fraudulent transactions by analyzing patterns and anomalies in transaction data. AI models can identify suspicious activities in real-time, allowing the bank to take immediate action and prevent significant financial losses.
Additionally, AI helps JPMorgan Chase in credit risk assessment by analyzing customer data and predicting the likelihood of default. This enables the bank to make more informed lending decisions and reduce the risk of bad loans.
Supply Chain Management
Another compelling example comes from the supply chain management sector. DHL, a global logistics company, uses AI-powered predictive analytics to manage supply chain risks. By analyzing data from various sources, including weather forecasts, geopolitical events, and market trends, DHL can predict potential disruptions and optimize its operations accordingly.
For instance, if AI predicts a natural disaster that could impact a critical supply route, DHL can reroute shipments and adjust inventory levels to minimize disruption. This proactive approach ensures smoother operations and maintains customer satisfaction.
Healthcare
In healthcare, AI-powered predictive analytics are used to manage clinical and operational risks. Mayo Clinic employs AI to predict patient outcomes and optimize treatment plans. By analyzing patient data, AI can identify high-risk patients and recommend preventive measures to improve their outcomes.
AI also helps healthcare providers manage operational risks by predicting staffing needs and optimizing resource allocation. For example, AI can forecast patient admission rates and ensure that adequate staff and resources are available to meet demand, reducing the risk of overcrowding and improving patient care.
Overcoming Challenges in AI-Powered Risk Management
Data Privacy and Security
Implementing AI in risk management involves handling sensitive data, which raises concerns about privacy and security. It’s crucial to implement robust data protection measures to safeguard sensitive information. Ensure compliance with relevant data privacy regulations, such as GDPR or CCPA, and implement encryption, access controls, and other security measures to protect your data.
Regularly audit your AI systems to identify and address any potential security vulnerabilities. Collaborate with cybersecurity experts to ensure that your AI tools are secure and compliant with industry standards.
Ethical Considerations
AI-powered risk management must also address ethical considerations. AI models can inadvertently introduce biases that lead to unfair or discriminatory outcomes. For example, if an AI model is trained on biased data, it may perpetuate existing inequalities in risk assessments and decision-making.
To mitigate this risk, ensure that your AI models are trained on diverse and representative data. Regularly review and update your models to eliminate biases and ensure fairness. Implement transparency measures to make AI-driven decisions understandable and accountable.
Change Management
Introducing AI into risk management requires a shift in how your organization operates. Effective change management is essential to ensure a smooth transition. Communicate the benefits of AI-powered predictive analytics to your team and provide training to help them understand and use the new tools effectively.
Engage stakeholders from various departments to ensure buy-in and address any concerns. Foster a culture of continuous learning and improvement, where employees are encouraged to experiment with AI tools and provide feedback for refinement.
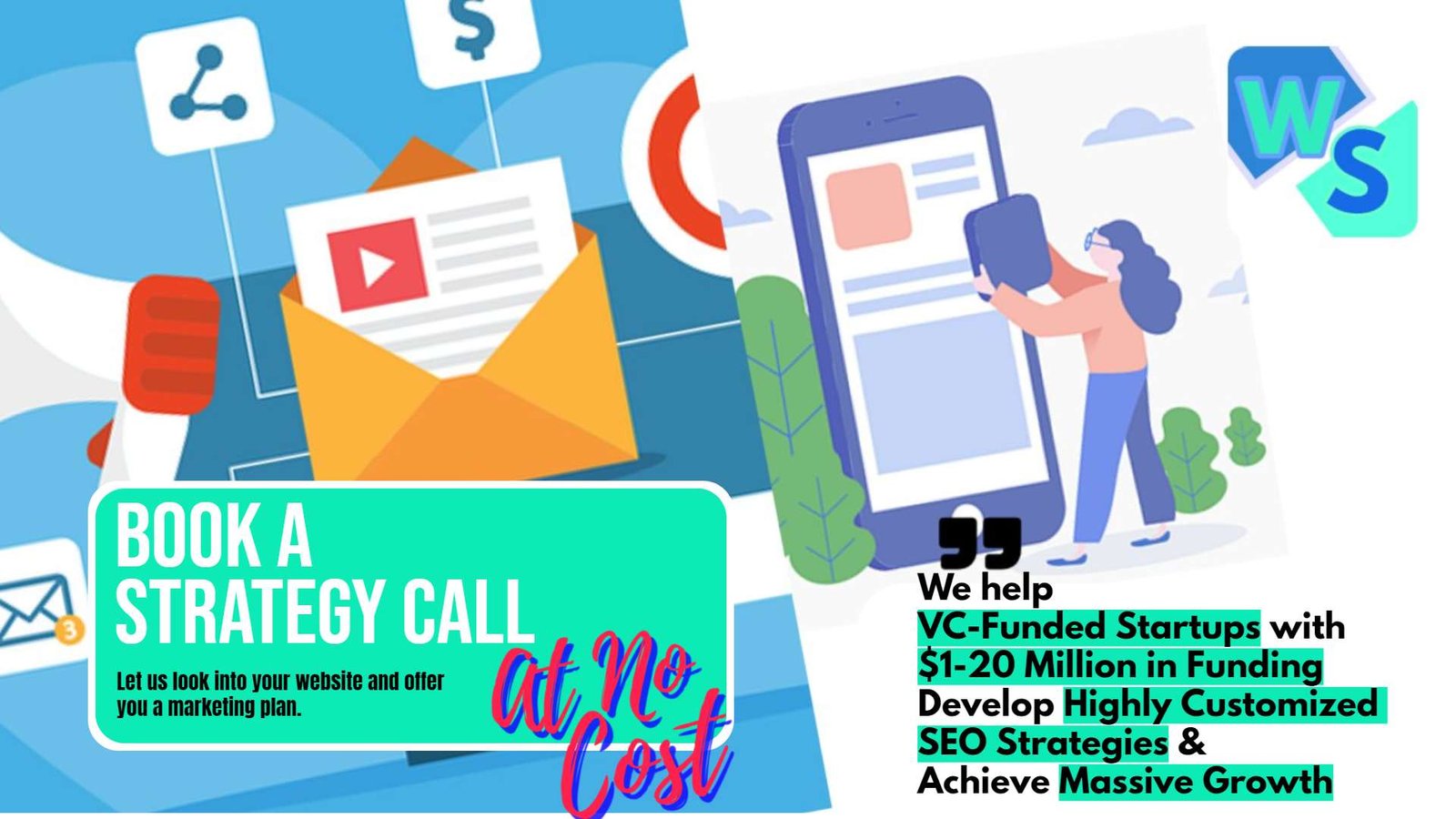
Related: Check out our free tools:
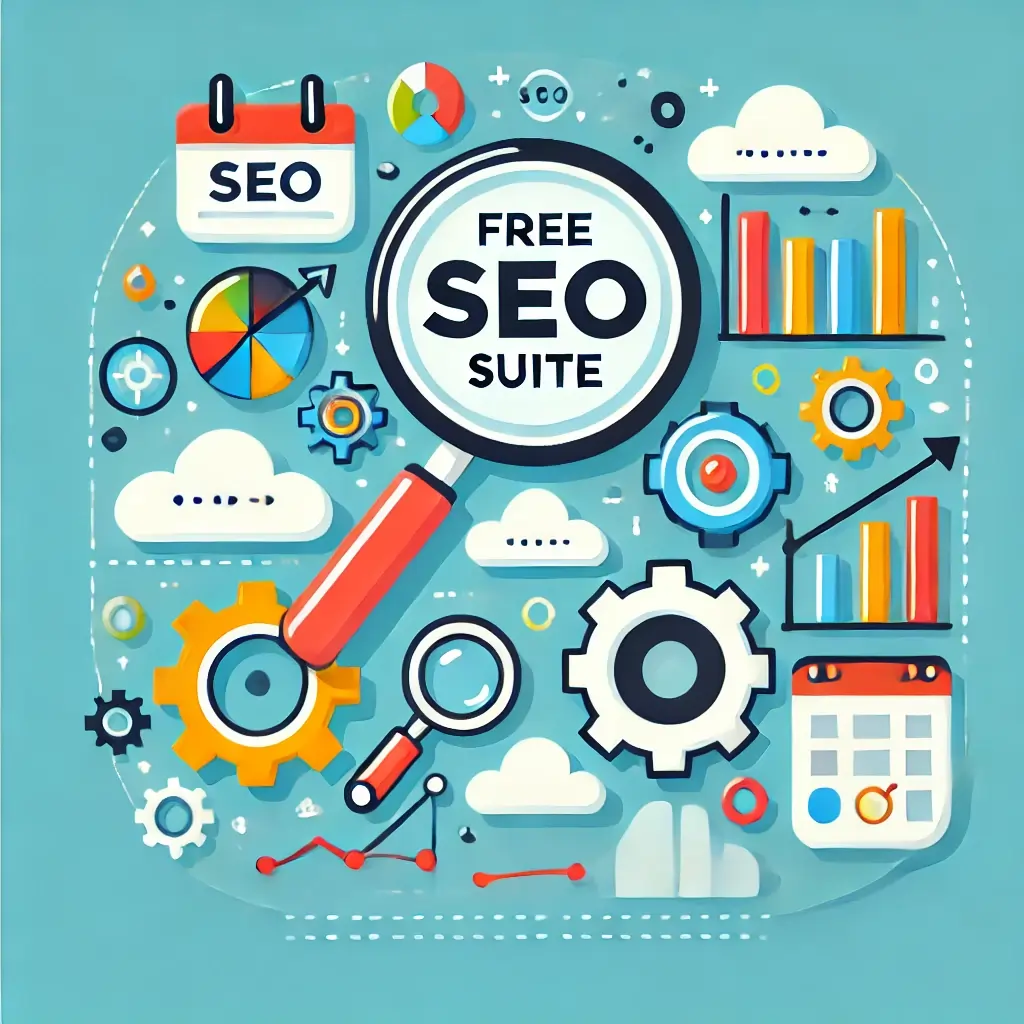
Future Trends in AI-Powered Risk Management
Personalized Risk Management Solutions
One of the emerging trends in AI-powered risk management is the development of personalized risk management solutions. As AI technology advances, it becomes possible to create highly customized risk management strategies tailored to the specific needs and characteristics of individual businesses. For startup founders, this means leveraging AI to design risk management frameworks that align closely with their unique operational models and market conditions.
To implement personalized risk management solutions, startups should begin by conducting a thorough risk assessment to identify their specific vulnerabilities and risk factors. Using AI tools, they can analyze historical data and real-time inputs to create dynamic, customized risk profiles. These profiles can then inform the development of targeted risk mitigation strategies, ensuring that resources are allocated efficiently and effectively.
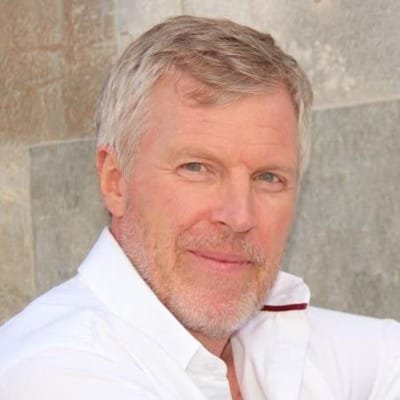
The key to using AI predictive analytics for maintenance schedules, to reduce operational risk, and to see equipment failures before they happen, is prior data.
A manufacturer who has years of maintenance, risk, and failure data can share this with AI along with any other relevant data and put it to use immediately.
However, most manufacturers would have to start inputting data today, and AI would not be terribly useful for these purposed for some time. In addition, AI requires a lot of computing power.
Right now, today, most manufacturers can’t justify the benefits due to the current costs. But look for this in the future, and start collecting data now.
AI-Driven Predictive Maintenance
In sectors such as manufacturing, logistics, and utilities, predictive maintenance powered by AI is becoming increasingly vital. Predictive maintenance uses AI algorithms to monitor the condition of equipment and predict when maintenance should be performed. This approach reduces downtime, prevents costly repairs, and extends the lifespan of assets.
Startup founders can integrate AI-driven predictive maintenance by first equipping their machinery and equipment with IoT sensors to collect data on performance and wear. AI tools can then analyze this data to predict potential failures and recommend maintenance schedules. By proactively maintaining equipment, startups can minimize operational disruptions and improve overall efficiency.
Ethical AI and Risk Management
As AI systems become more integrated into risk management, ethical considerations are gaining prominence. Ensuring that AI tools are used responsibly and ethically is crucial for maintaining trust and compliance. This includes addressing biases in AI models, ensuring data privacy, and being transparent about AI decision-making processes.
For startup founders, incorporating ethical AI practices into their risk management strategies involves several key steps. First, they should ensure that their AI models are trained on diverse and representative datasets to minimize biases. Regular audits of AI systems can help identify and correct any unintended biases that may emerge. Additionally, startups should prioritize data privacy and security, implementing robust measures to protect sensitive information. Transparency in how AI models make decisions is also essential, as it builds trust with stakeholders and ensures compliance with regulatory requirements.
Collaborative AI Systems
Another trend in AI-powered risk management is the development of collaborative AI systems. These systems enable different AI models and tools to work together, sharing data and insights to provide a more comprehensive view of risks. Collaborative AI systems can enhance decision-making by integrating various perspectives and expertise.
Startup founders can leverage collaborative AI systems by adopting platforms that facilitate data sharing and collaboration among different AI tools. For example, an AI system monitoring financial risks can share its insights with another AI system focused on operational risks, creating a more holistic risk management strategy. By fostering collaboration between AI tools, startups can gain deeper insights and develop more robust risk mitigation plans.
AI-Powered Scenario Analysis
Scenario analysis is a powerful tool for understanding potential future risks and their impacts. AI-powered scenario analysis takes this a step further by using advanced algorithms to simulate a wide range of scenarios and their probabilities. This allows businesses to explore the potential outcomes of different risk events and develop contingency plans.
For startup founders, implementing AI-powered scenario analysis involves identifying key risk factors and variables that could impact their business. AI tools can then simulate various scenarios, such as economic downturns, supply chain disruptions, or changes in consumer behavior. By understanding the potential impacts of these scenarios, startups can develop proactive strategies to mitigate risks and seize opportunities.
Real-Time Risk Assessment
Real-time risk assessment is becoming increasingly important in today’s fast-paced business environment. AI-powered tools can continuously monitor data and provide real-time assessments of emerging risks. This enables businesses to respond swiftly to potential threats and minimize their impact.
To implement real-time risk assessment, startup founders should integrate AI tools with their existing data systems to enable continuous monitoring. This might involve using AI to analyze real-time data from market trends, social media, customer feedback, and operational metrics. Automated alerts can notify relevant stakeholders of emerging risks, allowing for immediate action. Real-time risk assessment ensures that startups remain agile and can adapt quickly to changing conditions.
Augmented Risk Intelligence
Augmented risk intelligence combines AI with human expertise to enhance risk management capabilities. While AI excels at processing large amounts of data and identifying patterns, human experts bring contextual knowledge and critical thinking to the table. Together, they can create more effective risk management strategies.
Startup founders can implement augmented risk intelligence by using AI tools to gather and analyze data, while involving human experts in interpreting the insights and making strategic decisions. This collaborative approach ensures that risk management strategies are both data-driven and contextually relevant. Regular training and development for staff on using AI tools can further enhance their ability to leverage augmented risk intelligence effectively.
Integration with Blockchain Technology
The integration of AI with blockchain technology is an emerging trend that promises to enhance data security and transparency in risk management. Blockchain provides a secure and immutable ledger for recording transactions and data, ensuring that information is tamper-proof and verifiable. When combined with AI, this technology can enhance the accuracy and reliability of risk assessments.
For startup founders, integrating AI with blockchain involves using blockchain to securely store and share data that AI models rely on. This can be particularly valuable in sectors such as finance, supply chain, and healthcare, where data integrity is crucial. By ensuring that data is accurate and trustworthy, startups can improve the effectiveness of their AI-powered risk management strategies.
The Rise of Explainable AI
Explainable AI (XAI) is gaining traction as businesses seek to understand and trust the decisions made by AI models. XAI aims to make AI processes more transparent, allowing users to see how decisions are made and why certain outcomes are predicted. This transparency is essential for building trust and ensuring accountability in risk management.
Startup founders can adopt XAI by choosing AI tools that offer clear explanations of their decision-making processes. This might involve visualizing the data and logic used by AI models, providing detailed reports on how predictions are generated, and allowing users to interact with the models to understand their workings. By embracing XAI, startups can build greater confidence in their AI-powered risk management strategies and ensure that decisions are transparent and justifiable.
Future-Proofing Your AI Strategy
As AI technology continues to evolve, it’s crucial for startup founders to future-proof their AI strategies. This involves staying informed about emerging trends, continuously updating AI models, and investing in ongoing training and development for staff. By adopting a forward-thinking approach, startups can ensure that their AI-powered risk management strategies remain effective and relevant.
Future-proofing your AI strategy involves regularly reviewing and updating your AI tools and models to incorporate the latest advancements and best practices. Engaging with industry experts, participating in AI research communities, and attending conferences and workshops can help you stay abreast of new developments. Additionally, fostering a culture of innovation within your organization encourages experimentation and adaptation, ensuring that your AI strategy evolves with the changing business landscape.
Practical Applications of AI-Powered Predictive Analytics in Various Sectors
Market Trend Prediction
Financial markets are highly volatile and influenced by a myriad of factors. AI-powered predictive analytics can analyze vast amounts of historical data, news articles, and social media feeds to identify patterns and predict market trends.
For example, hedge funds and investment firms use AI to forecast stock prices and market movements. By predicting these trends accurately, investors can make informed decisions and optimize their investment strategies.
Credit Risk Assessment
Credit risk assessment is crucial for financial institutions to determine the likelihood of borrowers defaulting on loans. Traditional credit scoring models rely on limited data points, which might not provide a complete picture of a borrower’s creditworthiness.
AI-powered predictive analytics, on the other hand, can analyze a broader range of data, including transaction history, social media behavior, and other non-traditional data sources. This comprehensive analysis results in more accurate credit risk assessments, enabling financial institutions to make better lending decisions.
Fraud Detection
Fraud detection is another critical application of AI in the finance sector. AI-powered tools can analyze transaction patterns in real-time to identify suspicious activities.
For instance, if an unusual transaction is detected, the AI system can flag it for further investigation or automatically block it to prevent potential fraud. This proactive approach helps financial institutions mitigate fraud risks and protect their customers’ assets.
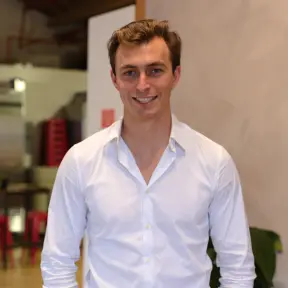
As the founder, CEO, and CFO of Premier Staff, I’ve pioneered the use of AI-powered fraud detection and risk management in the event staffing industry, a sector not traditionally associated with advanced financial technologies.
Our proprietary AI system, developed in-house, analyzes transaction patterns and client behaviors to identify potential fraud risks.
This has been crucial in protecting our business as we’ve scaled to handle multi-million dollar contracts for high-profile events with clients like Ferrari and Louis Vuitton.
We’ve implemented machine learning algorithms that continuously adapt to new fraud tactics, significantly reducing our exposure to financial risks.
This system has been particularly valuable in our expansion to new markets, where we may encounter unfamiliar business practices.
Our AI tools also assist in vetting potential clients and partners, analyzing vast amounts of data to identify red flags that human analysts might miss. This has been instrumental in maintaining our stellar reputation in the luxury event market.
While I can’t disclose specific metrics, I can say that our AI-driven approach has substantially lowered our fraud-related losses and strengthened our financial position, contributing to our rapid growth and industry leadership.
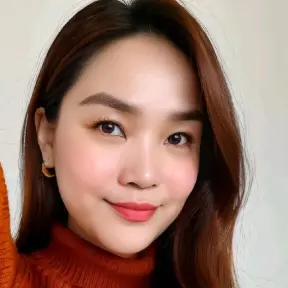
AI in fraud detection isn’t just transforming; it’s revolutionizing risk management by introducing real-time analysis and predictive capabilities unheard of in the past. However, as AI tools become smarter, so do fraudsters.
The irony? The same AI designed to prevent fraud can, in unscrupulous hands, be manipulated to outsmart existing security measures.
This evolving game of cat and mouse prompts an urgent need for continuous innovation in AI strategies.
Institutions must stay ahead by adopting adaptive AI technologies that learn and evolve faster than fraudsters can adapt, ensuring a resilient defense in the perpetual battle against financial crimes.
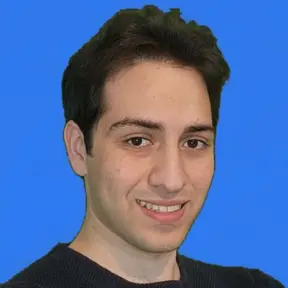
With over a decade of experience in financial technology, I’ve witnessed firsthand how AI is revolutionizing fraud detection and risk management in our sector.
AI is transforming fraud detection and risk management in financial services through several key innovations:
1. Real-time Transaction Monitoring:
AI algorithms can analyze transactions in real time, flagging suspicious activities instantly. At FinlyWealth, we’ve implemented machine learning models that evaluate hundreds of data points per transaction, including location, amount, merchant type, and user behavior patterns. This approach has allowed us to reduce false positives by 40% while increasing fraud detection rates by 25%.
2. Anomaly Detection:
AI excels at identifying patterns and anomalies that might escape human notice. We use unsupervised learning algorithms to establish baseline behaviors for each user and then flag deviations from these patterns. This has been particularly effective in detecting account takeovers, where the spending pattern suddenly changes.
3. Predictive Analytics:
Machine learning models can predict potential fraud before it occurs. These models assign risk scores to transactions or accounts by analyzing historical data and identifying risk factors. We’ve found that high-risk accounts identified by our AI are 5 times more likely to experience fraud attempts within the next 30 days.
4. Natural Language Processing (NLP) for Document Verification:
AI-powered NLP tools are revolutionizing document verification processes. These tools can quickly analyze and verify the authenticity of submitted documents, significantly reducing manual review time. In our experience, this has cut document verification time by 70% while maintaining accuracy rates above 99%.
5. Behavioral Biometrics:
AI algorithms can analyze user behavior patterns, such as typing speed, mouse movements, and device handling, to create a unique user profile. This adds an extra layer of security beyond traditional authentication methods. Since implementing behavioral biometrics, we’ve seen a 50% reduction in account takeover attempts.
6. Network Analysis:
AI can map and analyze complex networks of transactions and relationships to uncover organized fraud rings. Graph analytics, powered by machine learning, can identify subtle connections that might indicate collusion or money laundering activities.
7. Adaptive AI Models:
The fraud landscape is constantly evolving, and static models quickly become obsolete. We use adaptive AI models that continuously learn from new data, allowing our fraud detection systems to develop in real time as new fraud patterns emerge.
Best Practices:
1. Hybrid Approach: While AI is powerful, we’ve found that combining AI with human expertise yields the best results. Our fraud analysts work alongside AI systems, providing oversight and handling complex cases that require nuanced judgment.
2. Explainable AI: In the financial sector, it’s crucial to understand why certain decisions are made. We prioritize explainable AI models that can provide clear reasoning for flagged transactions, enhancing transparency and regulatory compliance.
3. Data Quality and Diversity: The effectiveness of AI models heavily depends on the quality and diversity of training data. We continuously refine our data collection processes and incorporate data from multiple sources to improve model accuracy.
4. Regular Model Evaluation: We conduct regular audits of our AI models to ensure they remain effective and unbiased. This includes testing against new fraud scenarios and monitoring for unintended consequences or biases.
5. Customer Education: While AI works behind the scenes, we’ve found that educating customers about fraud prevention enhances overall security. We use AI to personalize educational content based on each user’s risk profile and behavior.
The system was particularly effective in identifying a sophisticated fraud ring exploiting a specific payment gateway. The AI detected subtle patterns across seemingly unrelated accounts, preventing over $2 million in potential fraud losses.
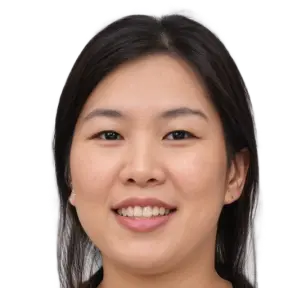
At DtglInfra, we’ve leveraged AI to revolutionize fraud detection and risk management for our financial services clients. Our experience has shown that AI is not just an add-on, but a fundamental shift in how we approach security in the digital finance landscape.
One standout case involved a mid-sized bank that was struggling with credit card fraud. We implemented an AI-driven anomaly detection system that analyzes transaction patterns in real-time.
The results were staggering – within three months, we saw a 60% reduction in fraudulent transactions and a 40% decrease in false positives.
The key to our success was the AI’s ability to learn and adapt. Unlike traditional rule-based systems, our AI continuously updates its fraud detection models based on new data. This dynamic approach allowed us to stay ahead of evolving fraud tactics.
We also developed an AI-powered risk assessment tool for loan applications. By analyzing a vast array of data points, including non-traditional sources like social media activity and online behavior, we were able to create more accurate risk profiles.
This led to a 25% increase in loan approvals for creditworthy applicants who might have been overlooked by traditional scoring methods.
One best practice we’ve identified is the importance of explainable AI in the financial sector. Regulators and customers alike demand transparency in decision-making processes.
We’ve focused on developing AI models that can provide clear reasoning for their decisions, which has been crucial in gaining trust and regulatory approval.
Another innovative application we’ve pioneered is using AI for predictive compliance. By analyzing regulatory changes and internal processes, our system can forecast potential compliance issues before they occur.
This proactive approach has helped our clients reduce regulatory fines by 35% on average.
The future of AI in financial fraud detection lies in its ability to process and analyze unstructured data.
We’re currently working on systems that can detect fraud attempts through voice analysis in call center interactions and anomalies in video banking sessions.
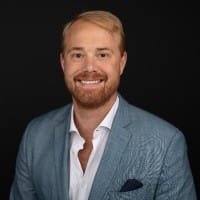
Fraud has always been a major problem in financial services. It’s a never-ending game of cat and mouse between banks and fraudsters, with both sides always trying to outdo the other.
But recently, AI has been shaking things up. Not only does it make things faster, but it makes everything much smarter. And really, it’s about time someone figured that out.
At Bloomfilter, I had the opportunity to see how data can truly change businesses, and AI is no exception. Especially when it comes to fraud detection and risk management.
What was once a purely reactive approach has become much more proactive. It was exciting to watch it unfold, to be honest with you. The new way to combat fraud with AI.
AI’s New Way of Tackling Fraud
In the past, fraud detection was based on established rules. If a transaction did not fall into the “normal” category, it was flagged. That’s all. The problem was that fraudsters eventually figured out these rules and found ways around them. But AI completely reverses the situation.
Instead of relying on rigid and outdated rules, AI learns as it goes and adapts in real time. These systems can process millions of transactions in the blink of an eye and detect subtle patterns that no one else could.
For example, let’s say you buy something in New York today, and tomorrow you are in Paris to make another purchase. An old system might flag this right away, but AI looks at your overall behavior, not just a single transaction.
What’s really cool about AI is that it’s always learning. The more it is used, the more powerful it is in identifying fraud. It’s like training a puppy, except this “puppy” learns from a mountain of data and gets smarter every time.
Cutting Down on Those Annoying False Positives
Reduces annoying false positives. We’ve all been there, haven’t we? Try to buy a coffee or something and bam! Your card is flagged as fraudulent for no reason. Yeah, it’s super frustrating. Banks do this because they want to be safe, but these false positives are a real headache. This is another area where AI helps.
Instead of flagging every little discrepancy, the AI looks at the bigger picture. It not only detects location changes but also takes into account your spending habits, time of day and other factors. It knows the difference between suspicious activity and the simple fact that you are on vacation.
A friend who works at a large bank told me how their AI system once blocked a ton of legitimate transactions, and when they switched to AI, the false positives dropped dramatically. Customers were much happier, and it made the work of their anti-fraud team easier.
Risk Management
Much more than just fighting fraud. Fraud detection is only part of AI’s job. AI also prevents risk management altogether.
Financial institutions have always tried to predict risks using data, but AI is also a game changer in this area. Now, AI can analyze what is happening in real time and even predict future risks.
Artificial intelligence is especially good at analyzing unstructured data, such as news articles, social media posts, or even random reports. AI brings all these factors together to get a more comprehensive picture of potential risks that may exist.
Banks no longer simply react to issues once they have occurred. They can actually see it coming, which is a big step forward in making things work.
Portfolio Risk Management
Managing portfolio risks involves assessing the potential impact of various market conditions on investment portfolios. AI-powered predictive analytics can simulate different market scenarios and their effects on portfolios.
This enables asset managers to identify potential risks and adjust their investment strategies accordingly. By leveraging AI, financial institutions can optimize their portfolios and achieve better risk-adjusted returns.
Predictive Diagnostics
AI-powered predictive analytics can analyze patient data, such as medical history, genetic information, and lifestyle factors, to predict the likelihood of developing certain diseases.
For example, AI can identify patients at high risk of diabetes or cardiovascular diseases and recommend preventive measures. By catching potential health issues early, healthcare providers can offer timely interventions and improve patient outcomes.
Operational Efficiency
AI can also optimize hospital operations by predicting patient admission rates, optimizing staff schedules, and managing inventory levels.
For instance, predictive analytics can forecast the number of patients expected in the emergency department on a given day, allowing hospitals to allocate resources effectively. This ensures that hospitals are adequately staffed and equipped to handle patient demand, enhancing overall operational efficiency.
Risk Management in Healthcare
In healthcare, risk management involves ensuring patient safety, maintaining compliance with regulations, and minimizing operational risks.
AI-powered predictive analytics can help healthcare providers identify potential risks, such as patient falls or medication errors, and implement preventive measures. Additionally, AI can ensure that healthcare providers comply with regulations by monitoring patient data and identifying any deviations from established protocols.
Predictive Maintenance
Predictive maintenance involves using AI to predict equipment failures before they occur. By analyzing data from sensors and historical maintenance records, AI can identify patterns that indicate potential equipment failures.
This enables manufacturers to schedule maintenance activities proactively, reducing downtime and maintenance costs. Predictive maintenance also extends the lifespan of equipment and improves overall operational efficiency.
Quality Control
AI-powered predictive analytics can enhance quality control by identifying defects and variations in the manufacturing process.
For example, AI can analyze data from production lines to detect anomalies that may indicate defects in the final product. By addressing these issues early, manufacturers can ensure that their products meet quality standards and reduce the risk of recalls.
Supply Chain Optimization
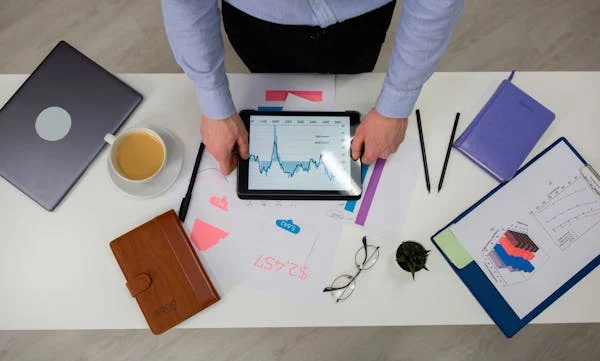
Supply chain optimization involves ensuring that products are delivered to customers efficiently and cost-effectively. AI-powered predictive analytics can analyze data from various sources, such as supplier performance, transportation routes, and market demand, to optimize supply chain operations.
For example, AI can predict potential disruptions in the supply chain and recommend alternative routes or suppliers to mitigate risks. This proactive approach ensures that products are delivered on time and at the lowest possible cost.
Customer Experience
AI can analyze customer data, such as purchase history and browsing behavior, to predict their preferences and recommend personalized products. This enhances the customer experience and increases the likelihood of repeat purchases.
For example, e-commerce platforms use AI to recommend products based on a customer’s previous purchases and browsing history. This personalized approach boosts customer satisfaction and drives sales.
Inventory Management
Effective inventory management is crucial for retail businesses to ensure that products are available when customers need them. AI-powered predictive analytics can analyze sales data and market trends to forecast demand accurately.
This enables retailers to optimize their inventory levels, reducing the risk of stockouts and overstocking. For example, AI can predict seasonal demand for certain products and recommend appropriate inventory levels to meet customer needs.
Sales Forecasting
Accurate sales forecasting is essential for making informed business decisions. AI-powered predictive analytics can analyze historical sales data, market trends, and other relevant factors to forecast future sales.
This enables retailers to plan their marketing strategies, manage inventory levels, and allocate resources effectively. For instance, AI can predict the impact of a promotional campaign on sales and recommend the optimal timing and budget for the campaign.
Route Optimization
AI can analyze traffic data, weather conditions, and other relevant factors to recommend the most efficient routes for deliveries. This reduces transportation costs, minimizes fuel consumption, and ensures timely deliveries.
For example, logistics companies use AI to predict traffic patterns and recommend alternative routes to avoid congestion. This proactive approach enhances the efficiency of transportation operations.
Fleet Management
Effective fleet management involves monitoring the performance of vehicles and ensuring that they are maintained properly. AI-powered predictive analytics can analyze data from vehicle sensors to predict potential issues and recommend maintenance activities.
This reduces the risk of breakdowns and extends the lifespan of vehicles. Additionally, AI can optimize fleet utilization by recommending the best routes and schedules for deliveries.
Timely Deliveries
Ensuring timely deliveries is crucial for customer satisfaction. AI-powered predictive analytics can analyze data from various sources, such as traffic patterns, weather conditions, and order volumes, to predict potential delays and recommend alternative strategies. For example, AI can recommend adjusting delivery schedules or using alternative transportation modes to ensure that products are delivered on time.
Conlusion
AI-powered predictive analytics offers transformative potential for risk management and strategic decision-making. For startup founders, understanding and implementing these advanced tools can provide a significant competitive advantage. By leveraging AI to analyze data, predict risks, and generate actionable insights, startups can make proactive decisions that drive success.
Read Next:
- How AI Helps in Content Personalization
- Best AI Writing Tools for Bloggers
- AI in Content Editing: Quick Tips
- AI-Powered Content Creation for Marketers
- How AI Transforms Data Analytics: A Deep Dive
Comments are closed.