Imagine a workplace where you can predict employee performance, identify potential leaders, and proactively address issues before they escalate. With AI in predictive HR analytics, this vision is becoming a reality. By leveraging artificial intelligence, companies can gain deep insights into their workforce, optimize performance, and foster a productive, engaging work environment. In this article, we’ll explore how AI-driven HR analytics can transform your business, providing actionable strategies to enhance employee performance.
Understanding Predictive HR Analytics
What is Predictive HR Analytics?
Predictive HR analytics uses data, statistical algorithms, and machine learning techniques to analyze current and historical employee data to predict future trends and outcomes. This approach allows HR teams to make proactive decisions that can improve employee performance, engagement, and retention. For startup founders, understanding and implementing predictive HR analytics can be a game-changer, enabling more effective management of human resources and driving business success.
Predictive HR analytics involves several key components: data collection, data analysis, and actionable insights. By integrating these components, startups can transform raw data into valuable insights that inform HR strategies and practices.
How AI Enhances HR Analytics
AI significantly enhances HR analytics by automating the data processing and analysis phases, leading to more accurate and comprehensive insights. Here’s a deeper look at how AI adds value to HR analytics:
Data Processing Efficiency: AI can process vast amounts of data quickly and accurately. For startups, this means being able to analyze everything from performance reviews and attendance records to engagement surveys and social media activity without manual intervention. This efficiency not only saves time but also ensures that decisions are based on the most up-to-date information.
Pattern Recognition: Machine learning algorithms excel at identifying patterns and correlations in data that may not be immediately obvious to human analysts. For instance, AI can identify that employees who participate in certain training programs tend to have higher performance ratings or that a specific demographic is more likely to leave the company. These insights enable startups to develop targeted strategies that address specific issues or capitalize on opportunities.
Predictive Capabilities: AI doesn’t just analyze past and present data; it predicts future outcomes. For example, predictive models can forecast which employees are likely to excel in leadership roles, who might be at risk of leaving, and what factors are most likely to influence job satisfaction and performance. These predictions allow startups to take proactive measures, such as offering tailored development programs or intervening early to prevent turnover.
Strategic Implementation for Startup Founders
Implementing predictive HR analytics in a startup environment involves several strategic steps:
Start with Clear Objectives: Define what you aim to achieve with predictive HR analytics. Are you looking to reduce turnover, identify potential leaders, improve overall employee performance, or enhance employee engagement? Clear objectives will guide your data collection and analysis efforts.
Invest in Data Infrastructure: Robust data infrastructure is crucial for successful predictive analytics. This includes data management systems that can handle large datasets, ensure data accuracy, and integrate data from various sources. For startups, investing in cloud-based solutions can provide the scalability and flexibility needed to manage growing data needs.
Leverage Cross-Functional Expertise: Predictive HR analytics requires collaboration between HR professionals, data scientists, and IT experts. HR professionals provide context and understanding of human factors, data scientists bring expertise in data analysis and machine learning, and IT ensures the technological infrastructure supports the analytics process. Building a cross-functional team ensures that all aspects of predictive analytics are addressed effectively.
Prioritize Data Quality: The accuracy of predictive models heavily depends on the quality of the data. Ensure that your data is clean, accurate, and comprehensive. Implement data governance practices to maintain high standards of data quality. This includes regular audits, data cleaning processes, and strict data management protocols.
Focus on Actionable Insights: Predictive analytics is valuable only if it leads to actionable insights. Ensure that the output from your predictive models is presented in a way that is easy to understand and act upon. Develop dashboards and reporting tools that translate complex data into clear, actionable recommendations for HR professionals and managers.
Real-World Applications and Examples
To understand the practical applications of predictive HR analytics, consider how leading companies leverage AI to transform their HR practices:
Google: Google uses predictive analytics to enhance employee retention by identifying factors that contribute to job satisfaction and performance. By analyzing data from employee surveys, performance reviews, and other sources, Google can predict which employees might be at risk of leaving and take proactive measures to improve their satisfaction and retention.
IBM: IBM employs AI to streamline its talent management processes. Predictive models help IBM identify high-potential employees who are likely to succeed in leadership roles. By focusing on these individuals, IBM can develop tailored career development plans and ensure a strong leadership pipeline.
Deloitte: Deloitte uses predictive analytics to optimize workforce planning. By forecasting future staffing needs based on historical data and market trends, Deloitte can ensure that it has the right talent in place to meet future business demands. This proactive approach allows Deloitte to stay ahead of market changes and maintain a competitive edge.
Actionable Advice for Startups
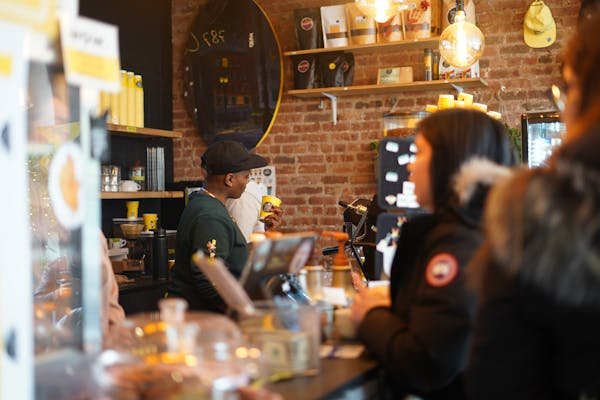
For startup founders looking to implement predictive HR analytics, here are some actionable steps to get started:
Identify Key Metrics: Determine the key performance indicators (KPIs) that are most critical to your HR objectives. This could include metrics related to employee performance, engagement, retention, and development. Focusing on these metrics will help you gather relevant data and build effective predictive models.
Develop a Data-Driven Culture: Encourage a culture where decisions are based on data and insights. Provide training and resources to help your team understand the value of predictive analytics and how to interpret and use the insights generated by AI. This cultural shift will ensure that predictive HR analytics is embraced and utilized effectively across your organization.
Start Small and Scale Gradually: Begin with a pilot project to test the effectiveness of predictive HR analytics in a specific area, such as employee retention or performance management. Use the insights gained from this pilot to refine your approach and gradually scale up to other areas of HR. This incremental approach allows you to manage risks and learn from initial implementations.
Partner with AI Experts: If your startup lacks in-house expertise in AI and data science, consider partnering with external experts or consulting firms. These partners can provide the necessary technical skills and guidance to help you implement predictive HR analytics effectively.
Monitor and Iterate: Continuous monitoring and iteration are crucial for the success of predictive HR analytics. Regularly review the performance of your predictive models and make necessary adjustments based on new data and feedback. This ongoing process ensures that your analytics remain accurate and relevant.
Benefits of AI in Predictive HR Analytics
Improved Employee Performance
AI can significantly enhance employee performance by identifying strengths and areas for improvement. By analyzing performance data, AI can provide personalized development plans tailored to each employee’s needs. This targeted approach ensures that employees receive the support and resources they need to succeed, leading to higher productivity and job satisfaction.
For example, if AI identifies that an employee struggles with time management, HR can provide specific training to help them improve. Conversely, if an employee excels in a particular area, they can be considered for roles that leverage their strengths, ensuring they remain engaged and motivated.
Enhanced Talent Management
AI-driven HR analytics can revolutionize talent management by identifying potential leaders and high performers early. By analyzing data such as past achievements, skill sets, and behavioral traits, AI can predict which employees have the potential to take on more significant roles within the organization.
This foresight allows HR teams to create tailored development programs and career paths for these individuals, ensuring they are ready to step into leadership positions when needed. This proactive approach not only helps retain top talent but also ensures the company has a strong leadership pipeline.
Reduced Turnover
Employee turnover is a significant challenge for many organizations, leading to high recruitment and training costs. Predictive HR analytics can help reduce turnover by identifying employees at risk of leaving and addressing their concerns proactively.
For instance, AI can analyze factors such as job satisfaction surveys, engagement scores, and social media activity to identify employees who may be disengaged or unhappy. HR can then intervene with strategies such as personalized career development plans, improved communication, or adjustments to their work environment to increase satisfaction and retention.
Data-Driven Decision Making
One of the most significant advantages of AI in HR analytics is the ability to make data-driven decisions. By providing accurate and actionable insights, AI enables HR professionals to make informed choices that enhance employee performance and overall business outcomes.
For example, AI can help HR determine the most effective training programs by analyzing their impact on employee performance. This ensures that resources are allocated to initiatives that provide the most value, leading to better outcomes for both employees and the organization.
Enhanced Employee Engagement
Engaged employees are more productive, motivated, and loyal to their organization. AI-driven HR analytics can help enhance employee engagement by identifying factors that contribute to satisfaction and addressing potential issues proactively.
For example, AI can analyze engagement surveys and feedback to identify common themes and areas for improvement. HR can then implement targeted initiatives, such as flexible working arrangements or wellness programs, to address these issues and improve overall engagement.
Predictive Analytics for Workforce Planning
Workforce planning is crucial for ensuring that an organization has the right people with the right skills at the right time. Predictive HR analytics can enhance workforce planning by forecasting future staffing needs based on historical data and trends.
For instance, AI can predict seasonal fluctuations in workforce requirements, allowing HR to plan for temporary hires or adjust schedules accordingly. This proactive approach ensures that the organization is always adequately staffed, reducing the risk of overstaffing or understaffing.
Implementing AI in Predictive HR Analytics
Assessing Your Data Needs
Before implementing AI in predictive HR analytics, it’s crucial to assess your data needs. Start by identifying the types of data that are most relevant to your HR goals. This could include performance reviews, attendance records, engagement surveys, employee demographics, and even social media activity.
Conduct a thorough data audit to determine what data you currently have and identify any gaps. Ensure that your data is clean, accurate, and well-organized. This may involve cleaning up outdated information, standardizing data formats, and integrating data from various sources into a centralized system.
Choosing the Right AI Tools
Selecting the right AI tools is essential for successfully implementing predictive HR analytics. There are numerous AI platforms and tools available, each offering different features and capabilities. Look for tools that can handle large datasets, support various data types, and provide robust analytical capabilities.
Consider cloud-based AI solutions like Google Cloud AI, IBM Watson, and Microsoft Azure AI. These platforms offer scalability and flexibility, making them ideal for handling the vast amounts of data required for predictive HR analytics. Evaluate each platform based on factors such as ease of integration with your existing systems, user-friendly interfaces, and support for compliance with HR regulations.
Building and Training AI Models
Once your data is prepared and you’ve chosen the right tools, the next step is to build and train your AI models. This involves selecting appropriate machine learning algorithms and feeding them with historical data to identify patterns and make predictions.
Define clear objectives for your AI models. For example, you might want to predict employee performance, identify high-potential employees, or forecast turnover risks. Use a portion of your data to train the model and another portion to test its accuracy. Continuously refine and improve the model to ensure it remains effective and relevant.
Collaboration with data scientists and HR professionals is crucial during this phase. Data scientists can provide expertise in selecting and tuning machine learning algorithms, while HR professionals can offer insights into the practical applications and relevance of the predictions.
Integrating AI into HR Processes
After building and training your AI models, the next step is to integrate them into your HR processes. This involves embedding AI insights into your day-to-day HR operations, making them easily accessible to HR professionals and managers.
Develop user-friendly dashboards and reporting tools that present AI-generated insights in a clear and actionable manner. Ensure that HR professionals and managers are trained to interpret and use these insights effectively. This might involve regular workshops, training sessions, and ongoing support to help them adapt to new tools and processes.
Monitoring and Continuous Improvement
The implementation of AI in predictive HR analytics is an ongoing process. Continuous monitoring and improvement are essential to ensure that your AI models remain accurate and relevant.
Establish key performance indicators (KPIs) to track the success of your AI initiatives. Common KPIs might include employee performance metrics, turnover rates, and engagement scores. Regularly review these metrics to identify areas for improvement and make necessary adjustments to your models and processes.
Create a feedback loop where insights from AI are continuously fed back into the system to refine and enhance the models. This iterative process helps your AI capabilities evolve with changing workforce dynamics and business needs.
Case Studies of AI in Predictive HR Analytics
IBM: Enhancing Employee Retention
IBM uses AI to enhance employee retention by analyzing factors such as job satisfaction, performance reviews, and engagement scores. By identifying employees at risk of leaving, IBM can proactively address their concerns and implement personalized retention strategies.
For example, IBM’s AI system might identify that an employee is disengaged due to a lack of career development opportunities. HR can then intervene with targeted training programs and career advancement plans to improve the employee’s satisfaction and reduce turnover risk.
Unilever: Streamlining Recruitment
Unilever leverages AI to streamline its recruitment process, ensuring that it hires the best candidates efficiently. By analyzing resumes, social media profiles, and interview performance, Unilever’s AI system predicts which candidates are most likely to succeed in various roles.
For example, AI can analyze a candidate’s resume and online presence to predict their fit for a specific job based on skills, experience, and cultural fit. This not only speeds up the recruitment process but also improves the quality of hires, ensuring that Unilever attracts top talent.
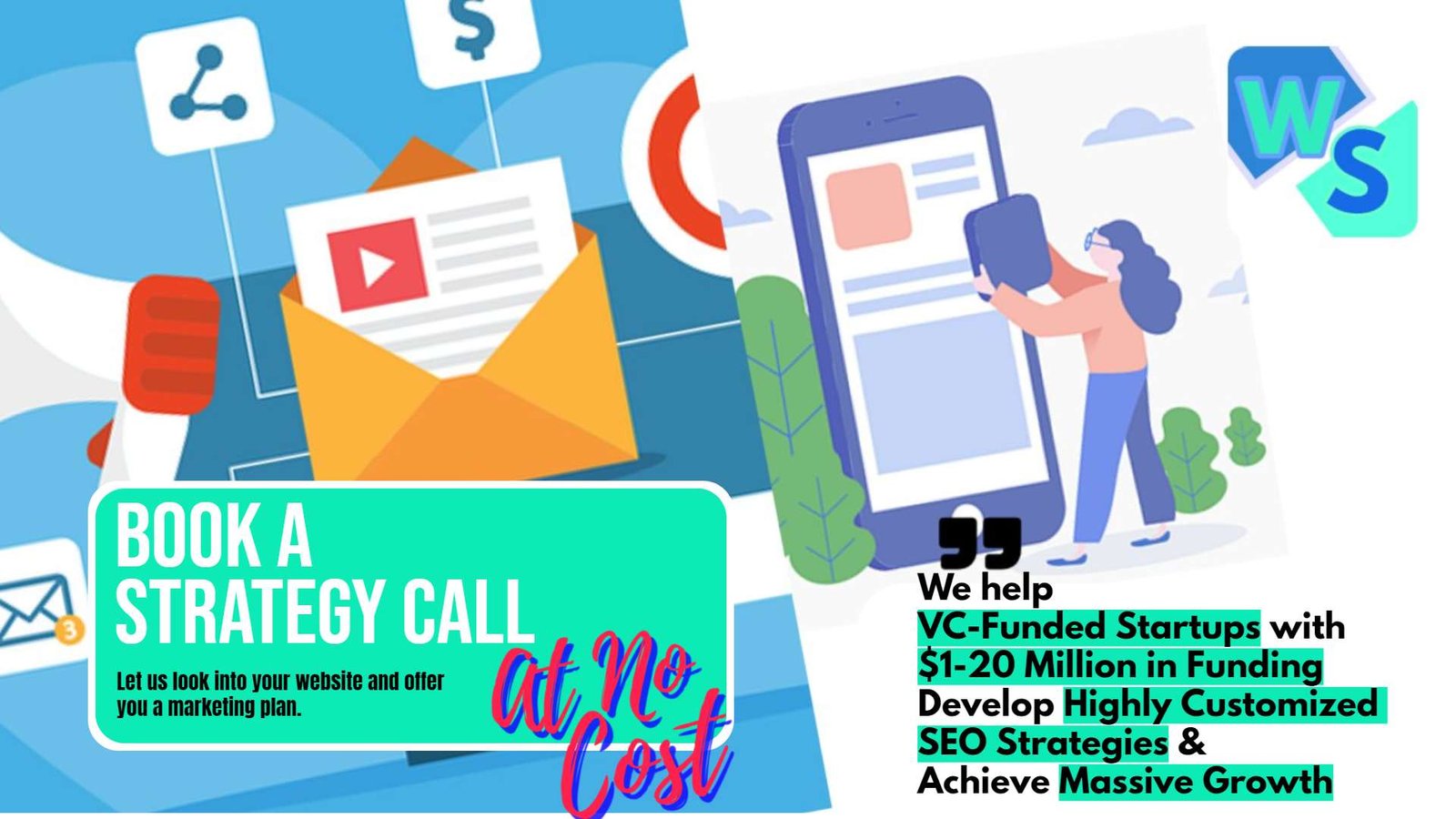
Related: Check out our free tools:
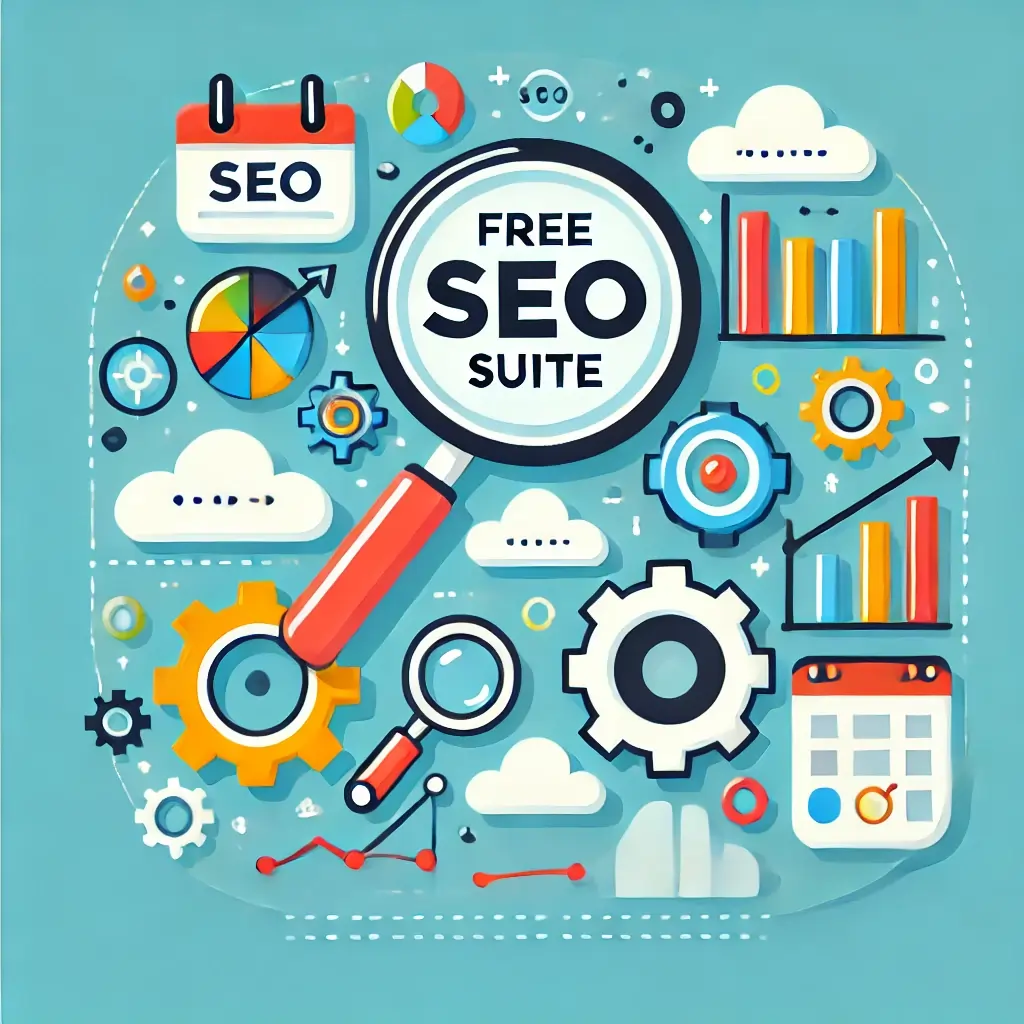
Google: Improving Employee Engagement
Google uses AI to improve employee engagement by analyzing feedback from surveys, performance data, and other sources. This enables Google to identify trends and issues that affect engagement and implement targeted initiatives to address them.
For example, if AI analysis reveals that employees in a particular department are consistently reporting high stress levels, HR can investigate the root causes and implement measures such as flexible working hours, wellness programs, or team-building activities to improve morale and engagement.
Microsoft: Optimizing Workforce Planning
Microsoft leverages AI to optimize workforce planning by predicting future staffing needs and identifying skill gaps. By analyzing historical data and market trends, Microsoft’s AI system forecasts demand for specific roles and skills, allowing HR to plan accordingly.
For example, AI might predict an increased demand for software engineers with expertise in a particular programming language. HR can then focus recruitment efforts on these skills, ensuring that Microsoft is prepared to meet future business needs and maintain a competitive edge.
Future Trends in Predictive HR Analytics
Advanced Personalization
As AI technology continues to evolve, advanced personalization techniques will become more prevalent in HR analytics. AI will enable companies to deliver highly personalized experiences for employees, from tailored training programs to customized career development plans.
For example, AI can analyze an employee’s performance data, feedback, and career aspirations to create a personalized development plan that aligns with their goals and the company’s needs. This level of personalization enhances employee satisfaction and retention by ensuring that each employee feels valued and supported in their career journey.
Real-Time Analytics
Real-time analytics will become increasingly important as businesses seek to respond to workforce trends instantly. AI-driven tools will enable HR professionals to analyze data in real-time, providing immediate insights that can inform decision-making.
For instance, real-time analytics can help HR monitor employee engagement levels continuously, allowing them to address issues as they arise rather than waiting for periodic surveys. This proactive approach ensures that potential problems are addressed quickly, maintaining a positive work environment.
Ethical AI and Transparency
As AI becomes more integrated into HR processes, ethical considerations and transparency will become crucial. Ensuring that AI models are fair, unbiased, and transparent is essential for building trust with employees and stakeholders.
Companies must prioritize ethical AI practices by implementing measures to detect and mitigate bias in their models. Being transparent with employees about how AI is used and the benefits it provides can also help build trust and acceptance. By adopting ethical AI practices, companies can ensure that their AI initiatives are both effective and responsible.
Integration with Employee Wellness Programs
The future of predictive HR analytics will see greater integration with employee wellness programs. AI can analyze data from wellness initiatives, such as fitness trackers and mental health surveys, to identify trends and tailor wellness programs to individual needs.
For example, AI can predict which employees might benefit from stress management programs based on their activity levels and survey responses. This targeted approach ensures that wellness programs are effective and address the specific needs of the workforce, promoting overall health and well-being.
AI and Workforce Diversity
AI can play a significant role in promoting workforce diversity by identifying and mitigating biases in recruitment, performance evaluations, and career development processes. By analyzing data objectively, AI can help ensure that all employees are evaluated fairly based on their skills and performance.
For example, AI can analyze recruitment data to identify any patterns of bias and suggest adjustments to job descriptions or interview processes to attract a more diverse pool of candidates. This proactive approach promotes diversity and inclusion, which can enhance creativity, innovation, and overall business performance.
AI for Continuous Learning and Development
Continuous learning and development are essential for keeping employees engaged and ensuring they have the skills needed to meet evolving business demands. AI can significantly enhance these efforts by identifying individual learning needs and providing personalized training recommendations.
For example, AI can analyze an employee’s current skill set, performance data, and career aspirations to suggest relevant training programs or courses. This not only helps employees develop skills that are aligned with their career goals but also ensures that the organization benefits from a highly skilled and adaptable workforce. For startup founders, investing in AI-driven learning and development tools can lead to a more competent and motivated team.
Predictive Analytics for Succession Planning
Succession planning is crucial for maintaining business continuity and ensuring that key roles are filled with capable leaders. AI can enhance succession planning by predicting which employees are most likely to succeed in leadership roles based on their performance, experience, and potential.
For instance, AI can analyze data from performance reviews, peer feedback, and career progression to identify high-potential employees who are ready for leadership development programs. This proactive approach allows HR to develop tailored succession plans, ensuring that the organization is prepared for future leadership transitions. Startup founders can use AI to create a strong leadership pipeline, reducing the risks associated with leadership gaps.
Integration with Remote Work Analytics
With the rise of remote work, AI-driven predictive HR analytics can help organizations manage and optimize remote teams more effectively. AI can analyze data from various remote work tools, such as collaboration platforms and time tracking software, to provide insights into productivity, engagement, and work patterns.
For example, AI can identify trends in remote work habits, such as peak productivity times or common challenges faced by remote employees. HR can use these insights to develop strategies for improving remote work experiences, such as offering flexible work hours, providing additional support resources, or implementing new collaboration tools. For startup founders, leveraging AI to optimize remote work can lead to higher productivity and employee satisfaction.
AI in Performance Management
AI can revolutionize performance management by providing real-time feedback and continuous performance evaluation. Traditional performance reviews are often infrequent and can be influenced by recency bias. AI can provide ongoing insights into employee performance, ensuring that feedback is timely and objective.
For example, AI can analyze an employee’s work output, collaboration metrics, and engagement levels to provide real-time performance feedback. This continuous feedback loop helps employees make improvements promptly and keeps them aligned with organizational goals. For startup founders, implementing AI-driven performance management can lead to a more agile and high-performing workforce.
Predictive Analytics for Employee Well-Being
Employee well-being is a critical component of overall productivity and job satisfaction. AI can enhance employee well-being initiatives by predicting potential issues and offering personalized recommendations for improvement.
For instance, AI can analyze data from employee wellness surveys, health records, and workplace behavior to identify signs of burnout or stress. HR can then develop targeted well-being programs, such as stress management workshops, mental health resources, or wellness challenges, to address these issues. For startup founders, prioritizing employee well-being through predictive analytics can lead to a healthier, happier, and more productive team.
AI-Driven Diversity and Inclusion Initiatives
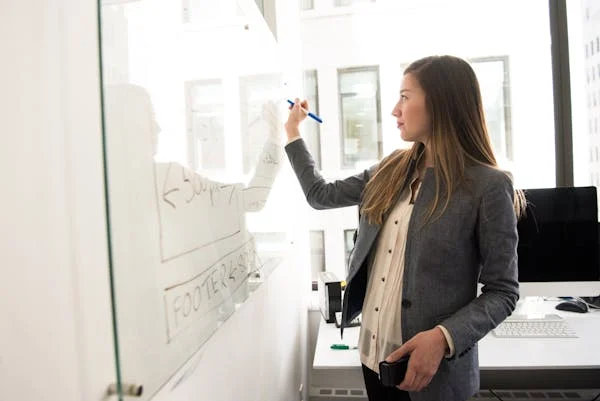
Diversity and inclusion are essential for fostering innovation and creating a positive work environment. AI can support diversity and inclusion initiatives by analyzing data to identify areas for improvement and tracking the effectiveness of these initiatives.
For example, AI can analyze hiring data to ensure that recruitment processes are free from bias and that diverse candidates are being considered for roles. Additionally, AI can monitor employee engagement and satisfaction across different demographic groups to identify any disparities and develop targeted inclusion programs. For startup founders, leveraging AI to promote diversity and inclusion can enhance organizational culture and drive better business outcomes.
Future-Proofing Your Workforce with AI
The rapid pace of technological change means that businesses must continuously adapt to stay competitive. AI can help future-proof your workforce by identifying emerging skills and predicting future workforce needs.
For instance, AI can analyze industry trends, job market data, and internal workforce analytics to predict which skills will be in demand in the coming years. HR can then develop training and development programs to upskill employees in these areas, ensuring that the organization remains agile and competitive. For startup founders, investing in AI-driven workforce planning can provide a strategic advantage in navigating future challenges.
Conclusion
AI in predictive HR analytics offers a transformative approach to managing and optimizing workforce performance.
For startup founders, harnessing this technology can lead to significant improvements in employee engagement, performance, and retention, all of which are crucial for long-term success. By leveraging AI, businesses can make data-driven decisions that enhance their human resources strategies and foster a productive and positive work environment.
Read Next:
- How Dentists Can Leverage Social Media Marketing
- Smart and Clever Marketing Ideas to Try
- Marketing Strategies to Grow Your Cleaning Business
- How to Create Engaging Email Marketing Content
- How New Realtors Can Market Themselves
Comments are closed.