In the competitive world of business, understanding your customers is key to success. One of the most effective ways to gain this understanding is through market segmentation. By dividing your market into distinct groups based on various characteristics, you can tailor your marketing strategies to meet the specific needs of each segment. This approach leads to more effective marketing campaigns, higher customer satisfaction, and increased sales. With the advent of artificial intelligence (AI), market segmentation has become more precise and actionable than ever before.
Understanding Market Segmentation
The Foundation of Market Segmentation
Market segmentation is a fundamental marketing strategy that involves dividing a broad target market into smaller, more manageable groups of consumers who share similar characteristics. This allows businesses to tailor their marketing efforts to meet the specific needs and preferences of each segment, leading to more effective and efficient marketing strategies.
For startup founders, understanding the foundation of market segmentation is crucial for creating targeted marketing campaigns that resonate with their audience and drive growth.
Market segmentation can be based on various criteria, including demographics, psychographics, behavior, and geography. By analyzing these different dimensions, businesses can gain a deeper understanding of their customers and create more personalized marketing messages. This approach not only improves customer satisfaction but also increases the likelihood of conversion and retention.
Demographic Segmentation
Demographic segmentation divides the market based on demographic factors such as age, gender, income, education, occupation, and family size.
This type of segmentation is often the starting point for many businesses because demographic data is relatively easy to obtain and analyze. For startup founders, demographic segmentation provides a straightforward way to identify and understand different customer groups.
For example, a startup selling educational toys might segment its market based on age groups of children, such as toddlers, preschoolers, and elementary school-aged kids.
Each segment would have different needs and preferences, allowing the startup to tailor its product offerings and marketing messages accordingly. By understanding the demographics of their target market, businesses can create more relevant and appealing marketing campaigns.
My work in developing platforms for data-driven insights has given me a deep understanding of how AI can elevate demographic and psychographic segmentation in marketing.
AI-driven analytics transforms traditional segmentation by diving deeper into not only who customers are but what motivates them. For example, AI can analyze vast datasets, combining demographic information—such as age, income, and education—with psychographic data like interests, lifestyle preferences, and values.
Through machine learning algorithms, these insights can predict what types of messages resonate most effectively with each segment. In the vacation rental market, this approach allows companies to identify high-value traveler personas: AI may reveal that one group prioritizes eco-friendly accommodations and immersive local experiences, while another seeks luxury amenities and seamless booking processes. By segmenting these audiences with precision, brands can create targeted campaigns that speak directly to each group’s unique desires.
Tools like IBM Watson and Google Cloud’s AI tools are commonly used to analyze these complex datasets, providing marketers with the ability to run highly personalized, scalable campaigns. With AI, companies are no longer limited to broad demographic groups but can instead refine their messaging to resonate on a personal level, fostering stronger customer loyalty and higher conversion rates.
With over 21 years of distinguished experience in marketing, operations, and IT, I have been involved in driving strategic initiatives and leading innovative marketing campaigns for different brands.
AI-driven analytics has transformed demographic and psychographic segmentation by enabling marketers to go beyond traditional data points such as age or income to understand the underlying motivations, interests, and values that drive customer behavior. AI tools analyze large amounts of data from multiple sources—social media, purchase histories, browsing patterns—to identify nuanced demographic traits and psychographic profiles. For example, at Max Cash, we use AI-driven platforms to segment audiences by income and financial behaviors, such as risk tolerance and savings habits.
Integrating AI with customer relationship management (CRM) and marketing automation tools helps us to create hyper-targeted campaigns that resonate on a deeper level.
For instance, if a segment shows high interest in sustainable products, we can tailor campaigns to emphasize eco-friendly offers, reinforcing alignment with their values. This level of segmentation increases engagement and loyalty, as customers feel seen and understood.
As AI tools such as Google Analytics 4 continue advancing, they provide real-time insights that enable us to adjust and optimize campaigns dynamically, making each touchpoint more relevant and impactful.
Psychographic Segmentation
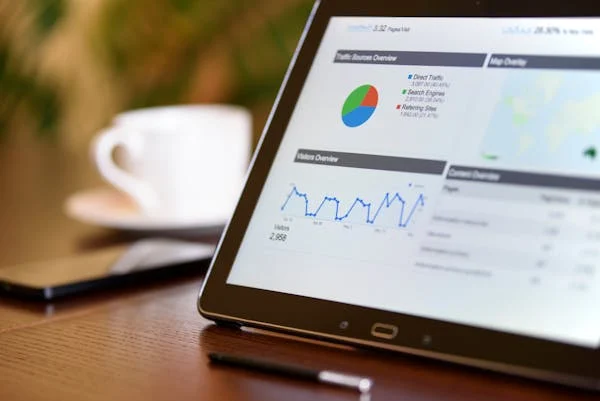
Psychographic segmentation goes beyond demographics to consider the psychological aspects of consumer behavior, such as values, attitudes, interests, and lifestyles.
This type of segmentation provides deeper insights into why consumers make certain purchasing decisions and how they perceive different brands. For startup founders, psychographic segmentation can reveal opportunities for differentiation and positioning.
For instance, a fitness apparel startup might segment its market based on customers’ fitness goals and attitudes towards health and wellness. One segment could consist of fitness enthusiasts who prioritize performance and technical features, while another segment might include casual exercisers who value comfort and style.
By tailoring their product development and marketing strategies to these different psychographic segments, the startup can create a stronger connection with its audience and build brand loyalty.
Behavioral Segmentation
Behavioral segmentation focuses on dividing the market based on consumers’ behavior and interactions with a brand. This can include factors such as purchasing habits, product usage, brand loyalty, and engagement with marketing campaigns. For startup founders, behavioral segmentation provides actionable insights into how customers interact with their brand and what drives their purchasing decisions.
For example, an e-commerce startup might segment its market based on customers’ purchasing frequency, such as one-time buyers, occasional shoppers, and loyal customers.
Each segment would require different marketing strategies to encourage repeat purchases and increase customer lifetime value. By analyzing customer behavior, businesses can identify opportunities to optimize their marketing efforts and improve customer retention.
Geographic Segmentation
Geographic segmentation involves dividing the market based on geographic factors such as location, climate, and population density. This type of segmentation is particularly useful for businesses with a broad geographic reach or those that offer location-specific products or services. For startup founders, geographic segmentation can help identify regional preferences and tailor their marketing strategies to different markets.
For instance, a food delivery startup might segment its market based on urban, suburban, and rural areas. Urban customers might prioritize quick delivery and a wide variety of restaurant options, while suburban customers might value family-sized meal options and affordable pricing. By understanding the geographic characteristics of their target market, businesses can create more effective marketing campaigns that resonate with local customers.
I’ve seen firsthand how AI-driven analytics transforms geographic segmentation, especially for businesses catering to varied regional needs. AI tools can process huge amounts of location-specific data, revealing customer behavior patterns, preferences, and even regional trends.
For example, AI algorithms can analyze local engagement data from social media or e-commerce platforms to find out which products resonate in different areas. I think this is a huge leap from traditional methods, allowing businesses to be much more precise in their targeting.
I’ve noticed that companies often use AI to tailor their marketing messages based on real-time, location-based insights. A great example is Starbucks, which uses AI-powered geo-analytics to adjust promotions based on weather, local events, and buying patterns in specific regions.
By analyzing such data, Starbucks personalizes its offers—like promoting cold beverages in warmer areas and seasonal flavors elsewhere—making its marketing much more relevant and engaging.
AI also helps with product availability strategies. Retailers like Walmart leverage AI-driven analytics to optimize inventory and stock particular items in specific locations based on local demand. This approach not only improves customer satisfaction but also maximizes efficiency.
My area of expertise is improving geographic segmentation for companies with a variety of regional audiences with AI-driven data. I assist businesses in forecasting regional trends, optimizing product offerings, and customizing marketing campaigns by evaluating location-specific data. Through data-driven decision-making, this enables companies to enhance consumer interaction, better target regional preferences, and boost sales growth.
AI tools help businesses analyze location-specific data by leveraging machine learning algorithms, data mining, and predictive analytics to understand regional behaviors, preferences, and trends. By processing vast amounts of geographic data, AI tools can segment audiences based on key location factors, including demographics, purchasing patterns, and regional market conditions. This allows companies to tailor marketing messages, product offerings, and service availability to meet the unique needs of customers in different areas.
For example, Coca-Cola uses AI to optimize regional marketing strategies. By analyzing purchasing trends and local preferences, they can adjust product offerings, promotions, and messaging in specific regions. Similarly, Amazon utilizes AI-driven analytics to adjust pricing, promotions, and stock availability based on regional demand, ensuring a more personalized customer experience in different geographic locations.
In the hospitality industry, Marriott uses AI to segment its customer base geographically, offering tailored experiences, packages, and discounts for specific regions, thus optimizing bookings and enhancing customer satisfaction.
By leveraging AI to analyze location-specific data, businesses can develop more effective, regionally relevant strategies that boost engagement, improve customer satisfaction, and increase overall sales.
I’m reaching out to share some insights on how AI-driven analytics is revolutionizing geographic segmentation, enabling businesses to tailor strategies for diverse regional audiences. With AI tools, companies can now leverage location-specific data to shape marketing messages, customize product offerings, and fine-tune service availability.
Data Precision: AI systems analyze vast sets of data to detect location-specific trends and preferences, providing actionable insights for targeting unique audience segments across regions.
Personalized Marketing: By tracking user behavior within geographic areas, AI can recommend content, products, and offers that resonate locally. For instance, [Example Company] uses AI to segment their audience based on regional weather trends, tailoring promotions to each region’s needs.
Dynamic Product Offerings: AI-driven analytics help identify popular products or services in specific regions. We have successfully adjusted one of our client’s product availability based on regional demand, improving customer satisfaction and retention.
Combining Segmentation Criteria
While each segmentation criterion provides valuable insights, the most effective market segmentation strategies often involve combining multiple criteria to create more detailed and accurate customer profiles.
For startup founders, using a combination of demographic, psychographic, behavioral, and geographic segmentation can lead to a more comprehensive understanding of their target market.
For example, a skincare startup might use demographic segmentation to identify different age groups, psychographic segmentation to understand attitudes towards skincare, behavioral segmentation to analyze purchasing habits, and geographic segmentation to identify regional preferences.
By combining these criteria, the startup can create highly targeted marketing campaigns that address the specific needs and preferences of each segment.
The Benefits of Market Segmentation
Market segmentation offers numerous benefits for businesses, particularly for startups looking to establish a strong market presence and drive growth. Understanding these benefits can help startup founders prioritize segmentation in their marketing strategy.
One of the primary benefits of market segmentation is improved marketing efficiency. By targeting specific segments with tailored marketing messages, businesses can allocate their marketing resources more effectively and achieve better results. This targeted approach reduces wasted efforts and increases the return on investment (ROI) for marketing campaigns.
Market segmentation also enhances customer satisfaction and loyalty. When businesses understand and address the unique needs and preferences of their customers, they can create more relevant and personalized experiences. This leads to higher levels of customer engagement, satisfaction, and loyalty, which are critical for long-term success.
Additionally, market segmentation can provide a competitive advantage by identifying underserved or niche markets. For startup founders, identifying and targeting these segments can create opportunities for differentiation and market leadership. By addressing the specific needs of niche segments, businesses can build a loyal customer base and establish a strong market position.
One standout example is our work with a digital health platform aiming to expand beyond general wellness into preventive care for chronic conditions, a challenging but underserved market segment.
Using AI models, we analyzed demographic, behavioral, and psychographic data to identify a subset of users likely to benefit from proactive health interventions. Our machine-learning algorithms uncovered trends in user behavior and health data that were otherwise obscured in broader datasets.
The results? A highly targeted outreach campaign that increased user engagement by over 40% within six months and a 30% uptick in long-term user retention. By leveraging AI, we could precisely tailor our messaging and channel strategies for this underserved group, showing a real-life example of how effective AI-driven segmentation can be.
AI allows us to go beyond surface-level metrics and dig deep into user patterns and preferences, making it possible to uncover and connect with niche audiences that would have otherwise remained hidden.
I’ve successfully used AI-driven market segmentation to uncover niche markets by leveraging machine learning algorithms that analyze customer behavior and preferences. This approach helps identify underserved segments that traditional methods might miss.
For example, in a recent project, we used predictive analytics to target a specific subgroup within the health and wellness industry, leading to a 30% increase in engagement within that segment.
One challenge was ensuring data accuracy, which we addressed by integrating multiple data sources. Overall, AI allowed us to personalize outreach effectively, delivering clear results in customer engagement and conversion.
AI lets us quickly analyze large amounts of data. By looking at customer demographics, service patterns, and locations, we can find groups that other companies might miss. For example, we noticed many rural areas needed plumbing and septic services, so we tailored our marketing to reach those communities.
Effective Ways to Reach These Audiences
- After identifying these segments, we used AI to create targeted ads. With specific keywords and messages for each group, we saw higher engagement.
- AI helps us know what services each segment needs most. In areas with older homes, we focused on heating and cooling services, which boosted conversions.
- By analyzing social media, we see what local communities talk about. This helps us connect with them and build trust as a go-to home services provider.
In one case, AI showed us a market for energy-efficient solutions among environmentally conscious customers. By promoting our sustainable heating and cooling options, we boosted sales in that category by 25% within a year.
I trust that I have adequately addressed your inquiry. If there are any other ways that I may be of assistance, please don’t hesitate to reach out to me.
At IBC24, AI-powered segmentation has completely redefined our strategy for connecting with niche audiences. Here’s one of our most impactful case studies:
In digital news, reaching specific segments is key, but traditional demographics often miss the mark, especially in diverse regions. We wanted to connect with underserved audiences in rural areas, yet typical demographic markers—like age or income—didn’t capture their interests.
We used AI-powered analytics tools to dig deeper, examining patterns in language, content preferences, and even viewing times. This revealed unique content clusters among audiences interested in hyper-local news and regional cultural stories. Using machine learning algorithms, we then segmented these clusters based on behavioral insights rather than standard demographics.
With these insights, we customized our content and delivery times. For instance, we produced short, culturally relevant video segments in regional dialects and released them at times that AI predicted as “high-engagement” windows.
The impact was huge! Engagement metrics soared, with a 62% increase in viewership among our targeted rural audience. Not only did our click-through rates improve by 45%, but our audience retention jumped by 50%, a key indicator of sustained interest.
Such AI-driven segmentation has really been quite effective and useful in identifying niche or underserved market segments, analyzing vast datasets to identify unique customer characteristics and behaviors. Machine learning can detect patterns that may be missed with traditional techniques, for example, regional preferences or even spending habits.
It also helps to break down an audience into more niche segments using demographics, buying history, and even psychographics. Businesses can thus target niche small markets based on tailored approaches to their needs. For example, AI can classify customers who demonstrate similarity in the buying patterns of your top performers but possibly were not targeted previously.
A case study of note is by Netflix, who through AI segmented the viewers and thus provides them with personalized content recommendations. In fact, knowing what each viewer prefers in advance allows targeting niche genres and shows to those viewers, which leads to greater engagement and retention.
I am a seasoned technologist, product leader in Healthcare with over 18 years leading strategic transformation initiatives at Fortune 500 companies.
It is during this time that I experienced first hand how complex, manual and painful regulatory processes are.
I founded Essenvia to solve this problem. We’re able to use AI tools to identify the especially niche search terms in our field and serve customers information-rich content that focuses on that specific niche.
These are terms that are essentially only searched by people in our industry, so this is an especially valuable angle for us.
My recent case study shows how insights into leveraging AI were used and resulted in a 28.47% increase in engagement, within an otherwise challenging niche audience.
AI-Driven Segmentation Unlocks New Niche Markets
AI can sift through behavioral data from purchase history, search patterns, and social media activity to uncover emerging needs within small audiences. We applied AI to find a segment interested in hypoallergenic, eco-friendly products not marketed by mainstream competitors.
Some Effective AI Methods for Reaching Niche Markets
Targeting niche segments, predictive analytics, and NLP in analyzing social media and customer reviews have been effective. NLP showed us not only recurring keywords and sentiments but those targeting health-conscious customers willing to pay for premium ingredients.
Overcoming Challenges and Achieving Results
The most critical challenge regarding AI-driven segmentation has been ensuring, so far, that the sources of data are of high quality and aligned. I designed a custom data cleaning protocol to ensure the reliability of insights. High accuracy enabled us to pin-point consumers who weigh sustainability practices over cost. Sustainability positioning messages increased loyalty and repeat purchases within this segment by 42.14%.
Implementing Market Segmentation in Your Business
For startup founders, implementing market segmentation requires a strategic approach and a commitment to continuous learning and improvement. Start by conducting thorough market research to gather data on your target market. This can include surveys, interviews, focus groups, and analysis of existing data sources.
Next, use this data to identify and define your market segments based on the criteria that are most relevant to your business. Develop detailed customer profiles for each segment, including demographic, psychographic, behavioral, and geographic characteristics. These profiles will serve as the foundation for your marketing strategy.
Create tailored marketing campaigns for each segment, using the insights gained from your market research. Develop personalized messages and offers that resonate with the specific needs and preferences of each segment. Continuously monitor and analyze the performance of your campaigns to identify opportunities for optimization and improvement.
How AI Transforms Market Segmentation
Processing Large Volumes of Data
One of the biggest challenges in market segmentation is processing and analyzing large volumes of data. Traditional methods can be time-consuming and limited in scope. AI-driven analytics can handle vast amounts of data quickly and accurately, identifying patterns and trends that might be missed by human analysts.
AI algorithms can analyze data from various sources, such as customer transactions, social media interactions, and website behavior. By processing this data, AI can uncover insights into customer preferences, behaviors, and needs. This comprehensive analysis enables businesses to create more precise and actionable segments.
Identifying Hidden Patterns
AI can identify hidden patterns in data that traditional methods might overlook. For example, AI algorithms can analyze purchase history to identify products that are frequently bought together. This information can be used to create segments based on buying behavior, such as customers who prefer premium products versus those who are price-sensitive.
Additionally, AI can identify patterns in customer interactions with marketing campaigns. By analyzing data on how customers respond to different types of content, AI can segment customers based on their engagement levels. This enables businesses to tailor their marketing strategies to different engagement segments, such as highly engaged customers who respond well to email campaigns versus less engaged customers who prefer social media interactions.
Enhancing Predictive Segmentation
Predictive segmentation involves using data to predict future behaviors and preferences of customers. AI-driven analytics can enhance predictive segmentation by analyzing historical data and identifying trends that indicate future behavior.
For example, AI can analyze past purchase data to predict which customers are likely to make repeat purchases. This information can be used to create segments of high-value customers who are likely to provide long-term value. Businesses can then create targeted retention strategies for these segments, such as loyalty programs and personalized offers.
Predictive segmentation also helps in identifying potential customers who are at risk of churning. By analyzing data on customer interactions, AI can identify patterns that indicate declining engagement. Businesses can then take proactive measures to re-engage these customers, such as offering special promotions or improving customer service.
Implementing AI-Driven Market Segmentation
Setting Clear Objectives
Before diving into AI-driven market segmentation, it’s crucial to set clear and measurable objectives. For startup founders, this means defining what you aim to achieve with your segmentation strategy.
These objectives could range from improving customer acquisition and retention rates to optimizing marketing spend and increasing sales. Having specific goals helps guide your AI implementation and ensures that your efforts are aligned with your broader business strategy.
To set effective objectives, start by analyzing your current market position and identifying key challenges and opportunities. For example, if your startup struggles with customer retention, your objective might be to use AI-driven segmentation to identify high-risk customers and develop targeted retention strategies. Clear objectives provide a roadmap for your AI initiatives and help measure their success.
Collecting and Preparing Data
High-quality data is the cornerstone of successful AI-driven market segmentation. Begin by identifying all potential data sources, including customer transactions, website interactions, social media activity, and CRM systems. The more comprehensive and diverse your data, the more accurate and actionable your AI-driven insights will be.
Once you’ve collected the data, the next step is data preparation. This involves cleaning and organizing the data to ensure it’s suitable for analysis. Data cleaning includes removing duplicates, correcting errors, and handling missing values.
Organizing data might involve structuring it into a consistent format and integrating it from various sources into a single database. Investing time and resources in data preparation is crucial for the accuracy and reliability of your AI models.
Choosing the Right AI Tools
Selecting the right AI tools and platforms is critical for the success of your market segmentation efforts. With numerous options available, it’s essential to choose tools that align with your specific needs and objectives. Consider factors such as ease of use, scalability, integration capabilities, and cost.
For instance, if your startup requires advanced natural language processing (NLP) capabilities to analyze customer feedback and social media interactions, tools like IBM Watson or Google Cloud NLP might be suitable.
If you need robust machine learning capabilities to handle large datasets and complex algorithms, platforms like Microsoft Azure Machine Learning or Amazon SageMaker can be valuable.
Conduct thorough research and, if possible, run pilot tests with a few selected tools to evaluate their performance and compatibility with your existing systems. Choosing the right tools ensures that you can efficiently implement AI-driven segmentation and derive meaningful insights.
Building and Training AI Models
Building and training AI models is a core component of AI-driven market segmentation. This process involves selecting appropriate machine learning algorithms and training them on your prepared data. The goal is to create models that can accurately identify and segment different customer groups based on the defined criteria.
Start by selecting algorithms that best suit your data and objectives. Commonly used algorithms for market segmentation include k-means clustering, hierarchical clustering, and decision trees. Each algorithm has its strengths and is suited for different types of segmentation tasks.
Once you’ve selected the algorithms, train your models using your dataset. This involves feeding the data into the algorithms and allowing them to learn patterns and relationships within the data. It’s important to continuously validate and refine your models to ensure their accuracy. This can be done by testing the models on a separate validation dataset and comparing their predictions to actual outcomes.
Integrating AI Insights into Marketing Strategies
The true value of AI-driven market segmentation lies in its application. Once you have your segments, the next step is integrating these insights into your marketing strategies. This involves creating personalized marketing campaigns tailored to the specific needs and preferences of each segment.
For example, if your AI-driven segmentation identifies a group of price-sensitive customers, you can design targeted promotions and discounts to attract this segment. Similarly, if another segment consists of high-value customers who prioritize premium features, you can focus on highlighting the unique benefits and exclusivity of your products.
In addition to campaign design, AI insights can also inform decisions about marketing channels, content, and timing. Use the insights to determine which channels are most effective for reaching each segment, what types of content resonate best, and when to engage your audience for maximum impact.
Continuously Monitoring and Improving
AI-driven market segmentation is not a one-time task but an ongoing process. Continuously monitor the performance of your segmentation efforts and make adjustments based on new data and insights. Regularly updating your segments ensures that they remain relevant and effective as market conditions and customer behaviors change.
Set up a feedback loop to gather data on the performance of your marketing campaigns and the response from different segments. Use this feedback to refine your AI models and improve the accuracy of your segmentation. For example, if a particular segment is not responding as expected to your campaigns, analyze the data to understand why and adjust your strategy accordingly.
Additionally, stay updated with advancements in AI technology and best practices in market segmentation. As new tools and techniques emerge, be open to experimenting and integrating them into your processes. Continuous learning and adaptation are key to maintaining the effectiveness of your AI-driven segmentation efforts.
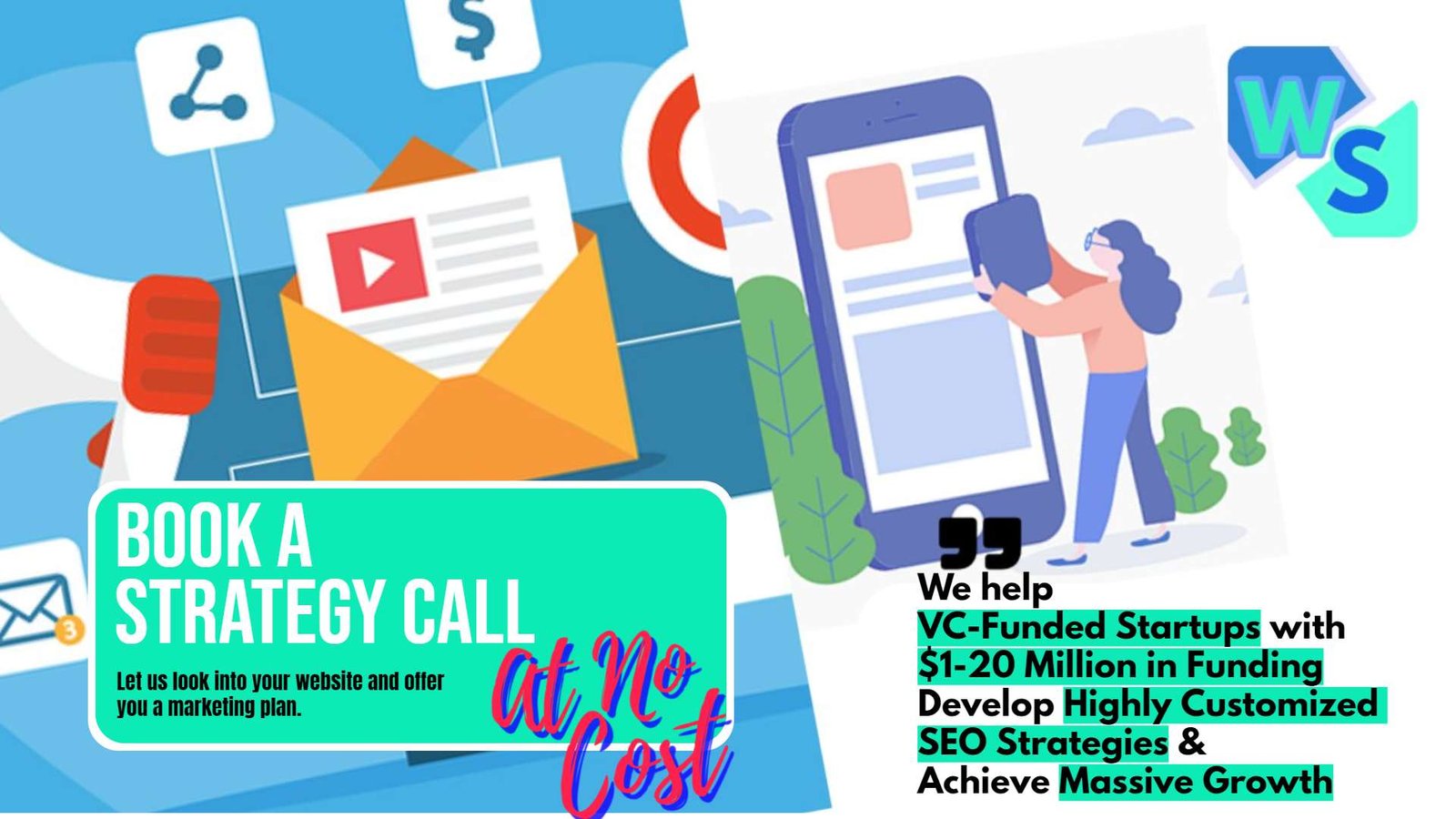
Related: Check out our free tools:
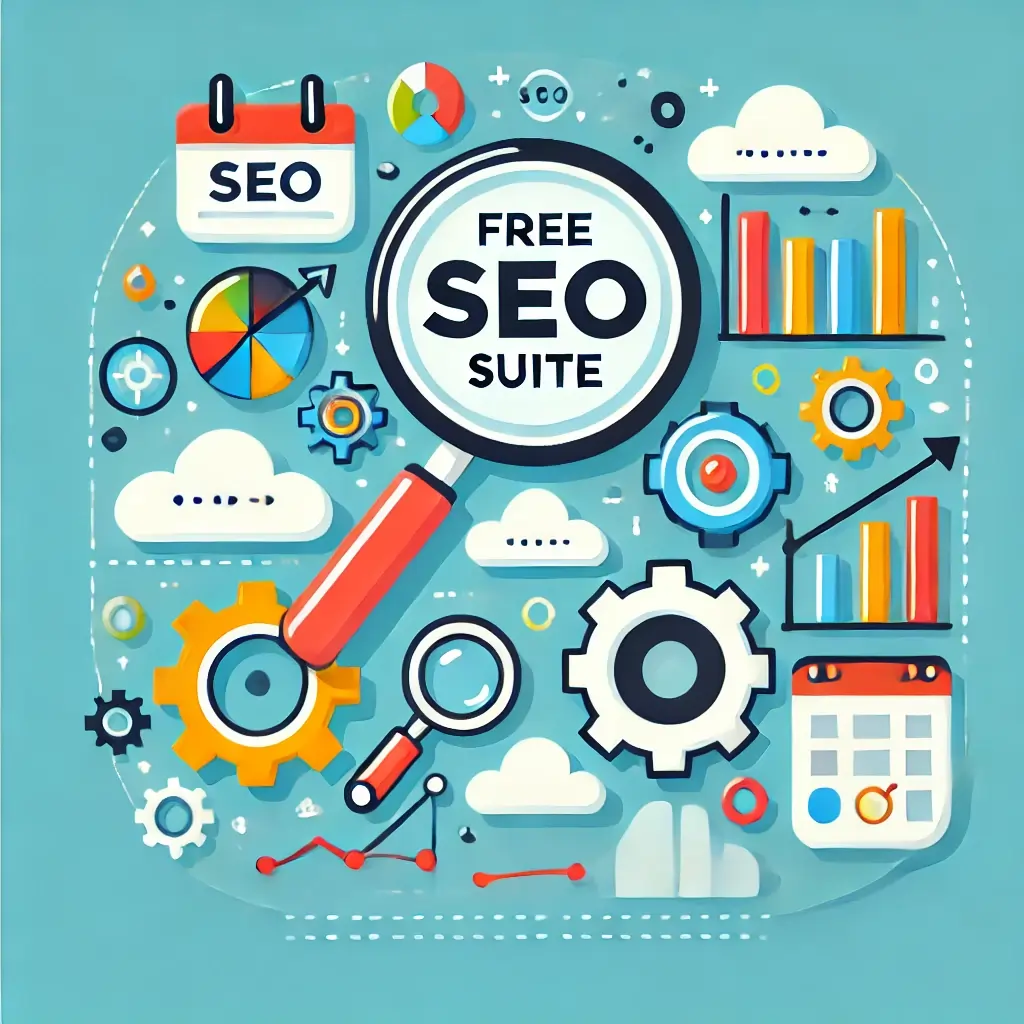
Collaboration and Cross-Functional Integration
Effective implementation of AI-driven market segmentation requires collaboration across different functions within your organization. For startup founders, fostering a culture of collaboration and cross-functional integration is crucial for success.
Engage teams from marketing, sales, customer service, and IT in the segmentation process. Each team can provide valuable insights and expertise that enhance the quality and application of your AI-driven insights. For instance, the marketing team can help interpret the segments and develop targeted campaigns, while the sales team can provide feedback on customer interactions and preferences.
Regular cross-functional meetings and workshops can facilitate knowledge sharing and ensure that everyone is aligned with the segmentation strategy. By promoting collaboration, you can maximize the impact of your AI-driven segmentation efforts and create a more cohesive and effective marketing approach.
Ethical Considerations and Transparency
As you implement AI-driven market segmentation, it’s important to consider the ethical implications and ensure transparency in your practices. For startup founders, building trust with customers and stakeholders is essential for long-term success.
Be transparent about how you collect and use customer data for segmentation. Clearly communicate your data practices and obtain explicit consent where necessary. Ensure that your AI models are designed and used in a way that respects customer privacy and avoids bias.
Advanced Strategies for Leveraging AI in Market Segmentation
Dynamic Segmentation
Dynamic segmentation is a powerful strategy enabled by AI that allows businesses to continuously update and refine their market segments in real-time. Unlike static segmentation, which relies on fixed data points, dynamic segmentation adapts to new data as it becomes available. This flexibility ensures that your segments remain relevant and actionable.
For example, an e-commerce platform can use dynamic segmentation to adjust its marketing strategies based on real-time customer behavior. If a customer who typically buys fitness products starts browsing home office equipment, AI can automatically reassign the customer to a different segment and trigger relevant marketing messages. This real-time adaptability ensures that your marketing efforts are always aligned with current customer interests and behaviors.
Through my work developing data-driven platforms, I’ve gained a keen understanding of how AI-based dynamic segmentation can transform real-time marketing.
AI-driven dynamic segmentation enables companies to refine marketing messages based on the latest customer behaviors, allowing for timely, contextually relevant interactions. By continuously analyzing recent interactions, purchases, and engagement metrics, AI can swiftly categorize customers into dynamic segments, which helps marketers respond with precision.
For example, if a customer shows high interest in a particular product category but hasn’t completed a purchase, AI can trigger an immediate tailored offer or recommendation, keeping the conversation relevant and nudging them toward conversion.
This method proves especially powerful in e-commerce, where companies can adapt ad campaigns based on browsing behavior or adjust promotions in real-time to retain potential buyers.
In leveraging these real-time insights, businesses create a fluid customer journey that adapts in response to every interaction, significantly enhancing both engagement and conversion rates. Dynamic segmentation makes it possible to deliver a personalized experience at scale—fostering a customer relationship that feels less like a one-way broadcast and more like an ongoing dialogue.
I’ve seen how AI helps us adjust our marketing based on what customers need right now. With AI, we can respond to customer actions in real-time and make our messages more relevant.
AI lets us quickly group customers based on their latest actions. For example, if a customer books a plumbing service, AI can add them to a group that might be interested in follow-up services, like drain cleaning. This way, we send messages that match what they’re currently looking for, instead of generic promotions.
We use this AI insight to tailor our email marketing. If a customer shows interest in heating services in winter, the AI adjusts our emails to feature heating maintenance offers. This approach helps us connect better with customers and boosts our conversion rates since our messages fit their current needs.
Recently, after heavy rainfall, we saw more requests for septic services. AI quickly spotted this trend, so we targeted customers who had shown past interest in septic maintenance. We sent them offers for inspections, and the response led to a jump in bookings.
AI-driven dynamic segmentation keeps us responsive to customer needs, helping us create more personalized experiences. It’s changed the way we reach customers, making our marketing more effective and driving growth.
AI enables businesses to use dynamic segmentation to personalize marketing in real-time. By analyzing recent customer behaviors, such as product views or purchases, AI algorithms can instantly adjust segmentation.
For example, if a customer has repeatedly viewed a product without purchasing, the system might move them to a “high-interest” segment, triggering targeted messaging like a limited-time discount or reminder.
This real-time segmentation improves engagement by delivering relevant, timely offers, boosting conversion rates.
Behavioral Segmentation
Behavioral segmentation focuses on grouping customers based on their interactions with your business. AI-driven analytics can analyze patterns in customer behavior, such as purchase history, browsing habits, and engagement with marketing campaigns. This type of segmentation provides deeper insights into customer preferences and motivations.
For instance, AI can identify customers who frequently purchase during sales events, suggesting a segment of price-sensitive shoppers. Similarly, it can highlight customers who engage with email newsletters but rarely make purchases, indicating a need for different engagement strategies. By understanding the nuances of customer behavior, businesses can create tailored experiences that resonate more deeply with each segment.
The Real-Time Challenge:
Today, businesses need to pivot their marketing strategies on a dime, but with fragmented data streams and static segmentation models, many fall behind in delivering relevant messaging. Studies show that 74% of customers expect personalized experiences in real-time, yet only a fraction of companies have the infrastructure to deliver.
The cxgenies Solution:
At cxgenies, we use AI to decode customer interactions across all channels—calls, emails, social media, and more. Our platform dynamically segments and adjusts marketing messages based on the latest engagement patterns, helping businesses stay relevant with minimal effort.
Instant Behavioral Insights: cxgenies captures real-time shifts in customer intent, allowing brands to adjust campaigns on the fly.
Actionable Segmentation: Our AI identifies emerging customer needs, empowering businesses to tailor their messaging with precision.
Improved Campaign ROI: By adapting in real-time, clients see higher engagement, lower churn, and increased customer loyalty.
Impact in Action:
One of our clients, a major retailer, achieved a 15% increase in customer retention within six months by leveraging real-time segmentation to adapt marketing campaigns based on live data from customer interactions.
With advances in AI and predictive analytics, now is the perfect time for brands to turn customer insights into immediate action. I’d be delighted to share more on how cxgenies is setting a new standard in dynamic segmentation and CX management.
My experience building platforms like SummerOS has given me an in-depth understanding of how AI-driven predictive models can profoundly enhance customer engagement and retention in a tech-forward environment.
Predictive behavioral segmentation harnesses AI to analyze patterns in customer data, enabling companies to anticipate customer needs and adapt their strategies effectively.
For example, by monitoring user engagement metrics, businesses can predict which customers might benefit from specific product features or who may be at risk of churning. When at-risk customers are identified, companies can initiate targeted interventions, such as personalized tutorials or exclusive offers, to re-engage and retain them.
This proactive approach enhances the user experience and boosts lifetime value by addressing potential dissatisfaction before it escalates to cancellation. Across industries, businesses employ similar segmentation techniques to refine marketing efforts, dynamically tailoring offerings to individual customer behaviors.
These AI-driven insights support hyper-personalized interactions that foster increased customer satisfaction and brand loyalty.
With over 21 years of distinguished experience in marketing, operations, and IT, I have been involved in driving strategic initiatives and leading innovative marketing campaigns for different brands.
AI-driven predictive segmentation is transforming how companies anticipate customer behaviors by using historical data to identify trends and forecast future actions. For example, predictive models can analyze purchase patterns, browsing habits, and even support interactions to assess the likelihood of churn or repeat purchases.
In the financial services sector, for instance, AI-driven analytics help identify clients who need loan refinancing or new financial products based on transaction trends. Companies enhance engagement and retention by proactively reaching out to these segments with tailored offers.
Similarly, e-commerce brands like Max Cash use predictive models to segment high-potential customers for exclusive rewards programs, increasing customer loyalty and lifetime value.
These predictive insights empower businesses to personalize interactions, reduce churn, and drive repeat purchases, ultimately building a more engaged and loyal customer base.
Predictive behavioral segmentation powered by AI is transforming customer engagement strategies by forecasting needs, predicting churn, and boosting lifetime value. By analyzing data patterns and customer interactions, AI helps companies precisely anticipate actions and tailor responses.
For example, in e-commerce, predictive AI models track browsing and purchase history to deliver personalized recommendations and promotions, which significantly improve both conversion rates and customer loyalty. One notable outcome is a 30% increase in repeat purchases when personalized offers align with predicted customer needs.
In the B2B manufacturing sector, AI-driven segmentation forecasts demand, allowing inventory adjustments that avoid overstock or stockouts. This proactive strategy enhances customer satisfaction and retention by meeting expectations consistently.
Because we’re in such a niche market, we work hard to identify the behavior of any users who do visit our sites or search for essential terms in our industry.
Especially when we get multiple visits from the same IP address or region, we can be sure that a manufacturer is exploring their options with us.
We can use this information to target them with ads or ideally identify the company and send direct emails.
Recognized by Forbes 30 Under 30 and MIT Innovators Under 35 for my work in AI applications, I believe I can offer valuable insights on how predictive segmentation can forecast customer behaviors.
In today’s data-rich environment, companies still struggle to anticipate customer needs, potential churn, and purchasing likelihood. According to Gartner, over 60% of marketers feel they lack effective tools for predictive analytics, leading to missed opportunities in customer retention and lifetime value enhancement.
Traditional segmentation methods often fall short due to:
Data Silos: Fragmented customer data across multiple channels hampers holistic analysis.
Limited Processing of Unstructured Data: Valuable insights from calls, emails, and social media are frequently overlooked because they’re harder to quantify.
At cxgenies, we’ve developed AI-driven predictive models that analyze omnichannel customer interactions—including calls, emails, reviews, and social media engagements—to forecast customer behaviors with high accuracy. Our platform not only predicts potential churn but also identifies opportunities for upselling and personalized engagement.
Case in point:
A leading retail client implemented our predictive segmentation tools and achieved:
25% increase in customer retention within six months.
15% boost in customer lifetime value by tailoring offerings based on predicted needs.
Reduction in customer complaints by 37%, enhancing overall brand reputation.
With advancements in AI and natural language processing, now is the perfect time for businesses to leverage predictive segmentation to stay ahead of customer expectations.
AI-driven models are at the forefront of this evolution, with AI enabling predictive segmentation that is revolutionizing customer behavior understanding and forecasting. With historical data available, companies can now create segments based on how likely a customer is to churn, buy something, or even interact with certain content.
This facilitates more personalized marketing strategies for brands all when businesses and brand owners can align their message to the right audience at exactly the right time.
At Digital Trawler, we have experience using predictive segmentation to help our clients increase their customer retention and customer lifetime value. As an example, we partnered with a SaaS client that used to offer subscriptions and was experiencing high churn rates. Predictive segmentation helped us find those customers who were about to churn—based on behavioral patterns, like fading engagement and a decrease in logins.
We were then able to send targeted retention campaigns to get these users re-engaged — special offers, check-in emails, and content that educated them on features they hadn’t yet used. This led to a 20% increase in retention rates over the next quarter.
Moreover, predictive segmentation allows brands to predict customer behavior and feature there in context products or content before the purchase is undertaken. And by tailoring offers to expected behaviors, brands are unlocking not just new conversion opportunities, but also richer customer relationships and greater loyalty.
Companies with a robust database of customer insights are able to leverage AI algorithms that can analyse the data, such as demographics, purchase history, and online behaviours, to detect emerging patterns.
This allows businesses to offer tailored solutions before a particular trend emerges. Having a human-in-the-loop ensures that potential churn can be accounted for. AI predictions need to accompanied by human insights, in order to make an informed decision that encompasses past experiences and actual knowledge of the customers.
One example is music platform, Spotify, that leverages AI to analyse listening habits and preferences, providing users with customised playlists and recommendations. Their use of AI predictions to offer users with a personalised experience of the platform has proven to boost user engagement and retention rates.
Psychographic Segmentation
Psychographic segmentation goes beyond demographics and behavior to consider the psychological aspects of customer decision-making. This includes values, attitudes, interests, and lifestyles. AI can enhance psychographic segmentation by analyzing unstructured data from social media, reviews, and other sources to uncover these deeper insights.
For example, AI can analyze social media posts to identify customers who are environmentally conscious and prefer sustainable products. These insights can inform marketing strategies that highlight eco-friendly features and appeal to the values of this segment. Psychographic segmentation helps businesses connect with customers on a more emotional and personal level, fostering stronger brand loyalty.
Geographical Segmentation
Geographical segmentation involves dividing the market based on location. AI can enhance this by analyzing location-specific data to identify regional trends and preferences. This is particularly useful for businesses with a broad geographic reach, such as national retailers or global brands.
For instance, an AI-driven analysis might reveal that certain products are more popular in specific regions due to cultural preferences or climate conditions. Businesses can use this information to tailor their product offerings and marketing messages for different locations. Geographical segmentation ensures that your strategies are relevant and effective across diverse markets.
Case Studies: Success Stories with AI-Driven Market Segmentation
Spotify: Personalized Playlists
Spotify is a great example of a company using AI-driven market segmentation to enhance user experience. By analyzing listening habits, song preferences, and engagement patterns, Spotify’s AI creates personalized playlists for each user. These playlists, such as “Discover Weekly” and “Release Radar,” are tailored to individual tastes, increasing user satisfaction and engagement.
Spotify’s AI analyzes billions of data points to identify patterns and predict what users will enjoy. This dynamic and behavioral segmentation approach ensures that each user’s experience is unique and highly relevant, leading to increased loyalty and reduced churn.
Amazon: Targeted Recommendations
Amazon leverages AI-driven market segmentation to provide targeted product recommendations. By analyzing purchase history, browsing behavior, and customer reviews, Amazon’s AI identifies segments of customers with similar interests and preferences. This allows Amazon to deliver personalized recommendations that drive sales and enhance the shopping experience.
For example, if a customer frequently purchases books in a specific genre, Amazon’s AI will recommend new releases and bestsellers in that genre. This behavioral and psychographic segmentation ensures that recommendations are highly relevant, increasing the likelihood of conversion.
Netflix: Customized Viewing Experiences
Netflix uses AI-driven market segmentation to customize viewing experiences for its users. By analyzing viewing habits, ratings, and interaction patterns, Netflix’s AI creates personalized content recommendations that cater to individual preferences. This segmentation strategy has been instrumental in keeping users engaged and satisfied.
Netflix’s AI also employs dynamic segmentation, continuously updating recommendations based on real-time data. If a user starts watching a new genre or type of content, Netflix quickly adapts its recommendations to reflect these new interests. This approach ensures that users always find content that matches their evolving tastes.
Overcoming Challenges in AI-Driven Market Segmentation
Ensuring Data Privacy and Security
One of the primary challenges in AI-driven market segmentation is ensuring data privacy and security. With increasing regulations such as GDPR and CCPA, businesses must handle customer data responsibly and transparently. Ensuring compliance with these regulations is crucial for maintaining customer trust and avoiding legal repercussions.
Implement strong data security measures, including encryption, access controls, and regular security audits. Be transparent with customers about how their data is used and obtain explicit consent where necessary. By prioritizing data privacy and security, businesses can build trust with customers and ensure the ethical use of AI.
Managing Data Quality
High-quality data is essential for accurate AI-driven market segmentation. Inaccurate or incomplete data can lead to incorrect insights and ineffective marketing strategies. Ensuring data quality involves implementing robust data management practices and continuously monitoring and improving data integrity.
Start by establishing data governance policies that define standards for data collection, storage, and usage. Use data cleaning tools to identify and correct errors, remove duplicates, and fill in missing values. Regularly audit your data to ensure it remains accurate and up-to-date. High-quality data ensures that your AI models provide reliable and actionable insights.
Addressing Algorithm Bias
Algorithm bias is a significant concern in AI-driven analytics. If the data used to train AI models is biased, the resulting insights and segmentation can also be biased. This can lead to unfair treatment of certain customer segments and negatively impact your business.
To address algorithm bias, ensure that your data is representative of your entire customer base. Use diverse data sources and regularly review your AI models for potential biases. Implement fairness-aware machine learning techniques that mitigate biases in your algorithms. By proactively addressing algorithm bias, businesses can ensure fair and equitable treatment of all customer segments.
The Future of AI-Driven Market Segmentation
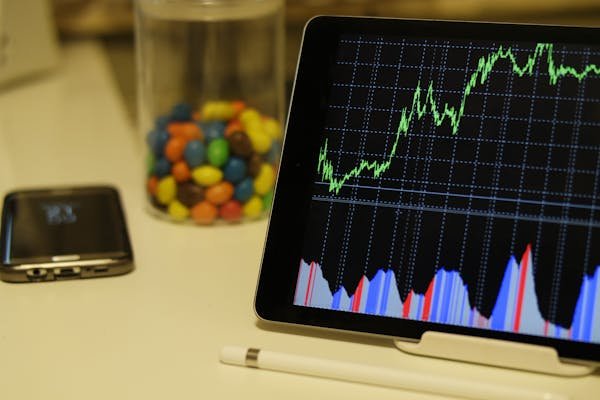
Continuous Advancements in AI Technology
The field of AI is continuously evolving, with new advancements enhancing its capabilities and applications. Future developments in AI, such as improved natural language processing and advanced machine learning algorithms, will further transform market segmentation efforts.
For example, advancements in natural language processing will enable more sophisticated analysis of unstructured data, such as customer reviews and social media posts. This will provide deeper insights into customer sentiments and preferences, enhancing psychographic segmentation. Improved machine learning algorithms will offer more accurate predictions and insights, driving better decision-making across the business.
Increasing Accessibility for Businesses of All Sizes
As AI technology becomes more advanced and affordable, it will become increasingly accessible to businesses of all sizes. Small and medium-sized enterprises (SMEs) will have greater opportunities to leverage AI-driven market segmentation, driving competitive advantage and growth.
Affordable AI solutions, user-friendly interfaces, and robust support will make it easier for SMEs to adopt and implement AI technologies. This democratization of AI will level the playing field, allowing businesses of all sizes to benefit from data-driven insights and innovation.
Expanding Applications Across Industries
The applications of AI-driven market segmentation are expanding beyond traditional industries such as retail and entertainment. From healthcare and finance to education and manufacturing, AI-driven segmentation will drive innovation and efficiency across various sectors.
In healthcare, AI can segment patients based on health conditions and treatment responses, enabling personalized care plans and improving patient outcomes. In finance, AI can identify segments of customers with similar risk profiles, guiding investment strategies and risk management. In education, AI can segment students based on learning styles and performance, enabling personalized learning experiences.
Conclusion
AI-driven market segmentation offers a powerful way for businesses to understand and engage their customers more effectively. By leveraging advanced analytics and machine learning, startups can identify precise customer segments, tailor their marketing strategies, and achieve better outcomes.
Implementing AI-driven market segmentation involves setting clear objectives, collecting and preparing high-quality data, choosing the right AI tools, and continuously refining your approach.
Read Next:
- How to Create Content with AI Tools
- How Much Time Do We Spend on Social Media? Key Statistics
- Budgeting for Small Business Marketing: Essential Statistics
- Conversational Marketing: Key Statistics and Strategies
- Flyer Marketing in 2024: Key Statistics and Effectiveness
Comments are closed.