In today’s data-driven world, businesses are constantly seeking ways to leverage their data for better decision-making and competitive advantage. Artificial Intelligence (AI) has emerged as a powerful tool in data analytics, transforming how organizations interpret and utilize their data. By effectively integrating AI into data analytics, businesses can uncover hidden patterns, predict future trends, and make informed decisions. This article will guide you through using AI in data analytics effectively, providing actionable strategies to harness its full potential.
Understanding AI in Data Analytics
The Evolution of AI in Data Analytics
AI in data analytics has evolved significantly over the past decade. Initially, AI was used primarily for basic data processing tasks. Today, AI technologies are capable of performing complex analyses, making accurate predictions, and providing actionable insights that drive business decisions. For startup founders, understanding this evolution is crucial for leveraging AI effectively.
Early AI applications focused on automating simple tasks such as data entry and basic analysis. These systems used rule-based algorithms that required explicit instructions for every task.
As machine learning and deep learning techniques developed, AI systems became more sophisticated, capable of learning from data and improving over time without explicit programming. This shift has enabled AI to handle more complex tasks, such as predictive modeling and natural language processing.
Startup founders should take advantage of these advancements by implementing AI technologies that can grow and adapt with their businesses. By staying informed about the latest AI developments and continuously integrating new capabilities, startups can maintain a competitive edge and drive innovation.
Differentiating AI from Traditional Analytics
Traditional data analytics relies heavily on human intervention to analyze data and extract insights. Analysts use statistical methods and predefined rules to interpret data, which can be time-consuming and prone to human error. In contrast, AI-driven analytics automates much of this process, using algorithms to analyze data quickly and accurately.
AI can process vast amounts of data at high speed, uncovering patterns and correlations that might be missed by human analysts. This capability allows businesses to make data-driven decisions more efficiently. For startup founders, this means being able to scale their operations without the need for a large team of data analysts.
Furthermore, AI-driven analytics can handle unstructured data, such as text, images, and videos, which traditional analytics tools struggle to process. This ability opens up new avenues for gaining insights from diverse data sources, such as social media posts, customer reviews, and video footage.
Strategic Advantages of AI in Data Analytics
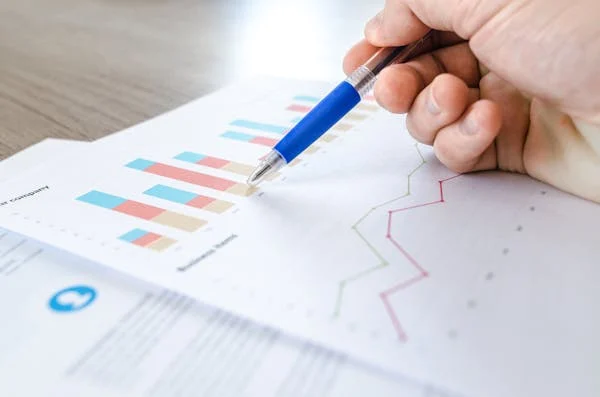
AI offers several strategic advantages for startups, enabling them to compete effectively with larger, more established companies. One of the primary benefits is the ability to gain deeper insights from data. AI algorithms can analyze complex datasets to reveal hidden patterns, trends, and relationships that inform strategic decision-making.
For example, AI can help identify emerging market trends before they become apparent to competitors. By analyzing social media activity, search trends, and consumer behavior, AI can predict shifts in customer preferences and market demand. This foresight allows startups to adapt their products and services proactively, staying ahead of the competition.
Another strategic advantage is improved customer segmentation. AI can analyze customer data to identify distinct segments based on behavior, preferences, and demographics. This granular understanding of the customer base enables startups to tailor their marketing efforts and product offerings to specific segments, increasing engagement and conversion rates.
Overcoming Challenges with AI in Data Analytics
Implementing AI in data analytics is not without its challenges. Startups must navigate issues such as data quality, integration, and the need for specialized skills. However, with the right approach, these challenges can be effectively managed.
Data quality is paramount for accurate AI analysis. Poor-quality data can lead to incorrect insights and flawed decision-making. Startups should invest in robust data collection and cleaning processes to ensure their data is reliable and accurate. This includes regular audits of data sources, validation checks, and the use of data preprocessing techniques to handle missing or inconsistent data.
Integration is another critical challenge. AI systems must be integrated seamlessly with existing data infrastructure to function effectively. This requires a clear understanding of the data ecosystem and careful planning to ensure compatibility and scalability. Startups should work closely with IT and data teams to develop an integration strategy that aligns with their business goals.
Building a skilled team is essential for leveraging AI effectively. Startups may face a shortage of in-house expertise in AI and data science. To address this, founders can invest in training programs, partner with academic institutions, or hire external consultants. Building a culture that values continuous learning and innovation will also attract and retain top talent in the field.
The Role of AI in Data-Driven Cultures
For AI to be truly effective, startups must foster a data-driven culture. This means promoting the use of data in decision-making at all levels of the organization. Leaders should set an example by using data to inform their strategies and encouraging teams to do the same.
Implementing AI tools that are user-friendly and accessible can help democratize data analytics within the organization. When employees across departments can easily access and interpret data, they are more likely to use it in their daily work. Training programs and workshops can also help build data literacy, ensuring that all team members understand the basics of data analysis and how to leverage AI tools effectively.
Creating a feedback loop where insights from AI analytics are regularly reviewed and acted upon is crucial. This iterative process allows startups to continuously refine their AI models and data strategies, ensuring they remain relevant and effective.
Getting Started with AI in Data Analytics
Conducting a Needs Assessment
For startup founders, the first strategic step in integrating AI into data analytics is conducting a thorough needs assessment. This involves evaluating your current data capabilities, understanding your business goals, and identifying specific areas where AI can add value. Start by asking critical questions: What are your key business objectives? Where are the current gaps in your data analytics processes? How can AI help bridge these gaps?
A detailed needs assessment will help you pinpoint the most impactful use cases for AI in your organization. For example, if customer churn is a significant issue, predictive analytics could help identify at-risk customers and suggest retention strategies. If operational efficiency is your goal, AI-driven process automation might be the solution. By clearly defining your needs, you can ensure that your AI initiatives are focused and aligned with your strategic objectives.
Prioritizing Use Cases
Once you’ve identified potential areas where AI can make a difference, the next step is to prioritize these use cases based on their potential impact and feasibility. Startups often have limited resources, so it’s crucial to focus on projects that offer the highest return on investment (ROI).
Consider factors such as the complexity of implementation, the availability of data, and the expected benefits. For instance, a project that requires minimal changes to existing infrastructure but promises significant cost savings should be prioritized. Conversely, highly complex projects with uncertain outcomes might be better suited for later stages of your AI journey.
By prioritizing use cases, you can allocate resources effectively and achieve quick wins that demonstrate the value of AI to stakeholders and build momentum for further initiatives.
Setting Measurable Goals
For AI in data analytics to be effective, it’s essential to set clear, measurable goals. These goals should be specific, achievable, relevant, and time-bound (SMART). Define what success looks like for each AI project and establish key performance indicators (KPIs) to track progress.
For example, if your goal is to reduce customer churn, a measurable objective could be to decrease churn rates by 10% within six months. KPIs might include the number of at-risk customers identified, the effectiveness of retention strategies implemented, and overall changes in churn rates.
Having measurable goals helps ensure that your AI initiatives stay on track and deliver tangible results. It also provides a basis for evaluating the success of your projects and making data-driven adjustments as needed.
Building the Right Infrastructure
Effective AI implementation requires a robust data infrastructure. This includes scalable storage solutions, powerful computing resources, and reliable data pipelines. For startups, investing in cloud-based infrastructure can be a cost-effective way to access the necessary resources without significant upfront investment.
Cloud platforms like AWS, Google Cloud, and Microsoft Azure offer a range of AI and machine learning services that can be easily integrated into your existing systems. These platforms provide scalable computing power, storage, and advanced analytics tools, enabling you to build and deploy AI models efficiently.
Additionally, ensure that your data infrastructure supports seamless data integration. This involves setting up data pipelines that can collect, process, and store data from various sources, such as CRM systems, social media, and transactional databases. A well-integrated data infrastructure ensures that your AI models have access to high-quality, real-time data.
Data Preparation and Management
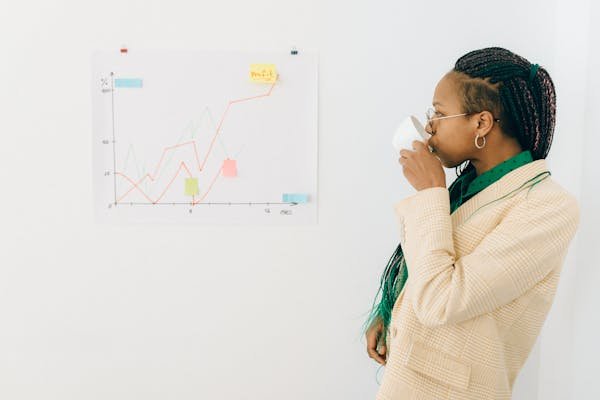
Data preparation is a critical step in any AI project. Clean, accurate, and well-organized data is essential for building reliable AI models. Start by collecting relevant data from all available sources and ensure that it is properly formatted and labeled.
Data cleaning involves identifying and correcting errors, handling missing values, and removing duplicates. This process can be time-consuming but is crucial for ensuring the accuracy of your AI models. Use data preprocessing techniques to transform and normalize data, making it suitable for analysis.
Implement data management practices that ensure ongoing data quality. This includes regular audits, validation checks, and data governance policies. By maintaining high data standards, you can ensure that your AI models continue to deliver accurate and reliable insights.
Collaborating with Experts
Building a successful AI-driven data analytics capability often requires collaboration with experts in the field. If your startup lacks in-house AI expertise, consider partnering with external consultants or hiring AI specialists.
Collaboration with academic institutions, research organizations, and technology partners can provide access to cutting-edge AI technologies and methodologies. These partnerships can also offer valuable insights and guidance on best practices for AI implementation.
When collaborating with experts, clearly define the scope of work, deliverables, and expected outcomes. Regularly review progress and ensure that the collaboration aligns with your strategic goals. By leveraging external expertise, you can accelerate your AI initiatives and achieve better results.
Iterative Development and Continuous Improvement
AI implementation should be viewed as an iterative process. Start with small, manageable projects and gradually scale up as you gain experience and confidence. Use an agile development approach that allows for continuous testing, feedback, and refinement.
Regularly monitor the performance of your AI models and make data-driven adjustments as needed. This might involve retraining models with new data, fine-tuning algorithms, or exploring alternative approaches. By continuously improving your AI capabilities, you can ensure that they remain effective and aligned with your business objectives.
Encourage a culture of experimentation and innovation within your organization. Provide your team with the freedom to explore new ideas and approaches, and support them with the necessary resources and training. This mindset fosters continuous improvement and helps your startup stay at the forefront of AI-driven data analytics.
Measuring and Communicating Success
Measuring the success of your AI initiatives is crucial for demonstrating their value and securing ongoing support from stakeholders. Use the KPIs defined earlier to track progress and evaluate outcomes.
Communicate the results of your AI projects to stakeholders clearly and effectively. Highlight the tangible benefits achieved, such as cost savings, revenue growth, or improved customer satisfaction. Use data visualizations and real-world examples to illustrate the impact of AI on your business.
Regularly report on the progress of your AI initiatives and share insights gained from the process. This transparency builds trust and ensures that stakeholders remain engaged and supportive of your AI journey.
Implementing AI in Data Analytics
Data Collection and Preparation
The first step in implementing AI in data analytics is data collection and preparation. Gather relevant data from various sources, such as customer transactions, social media interactions, and operational logs.
Ensure that your data is clean, accurate, and up-to-date. Data preparation involves cleaning, transforming, and organizing your data to make it suitable for analysis. High-quality data is crucial for accurate AI predictions and insights.
Selecting and Training AI Models
Once your data is prepared, the next step is selecting and training AI models. Choose models that are best suited to your data and objectives. This might involve supervised learning, unsupervised learning, or reinforcement learning techniques.
Train your models using historical data, and validate their performance to ensure accuracy. Continuously monitor and refine your models to improve their predictive capabilities.
Integrating AI into Business Processes
Integrating AI into your business processes involves embedding AI-powered analytics into your day-to-day operations. This might include automating routine tasks, enhancing decision-making processes, and providing real-time insights to support strategic initiatives.
Ensure that your team understands how to use AI tools and interprets the insights they generate. Effective integration will maximize the impact of AI on your business performance.
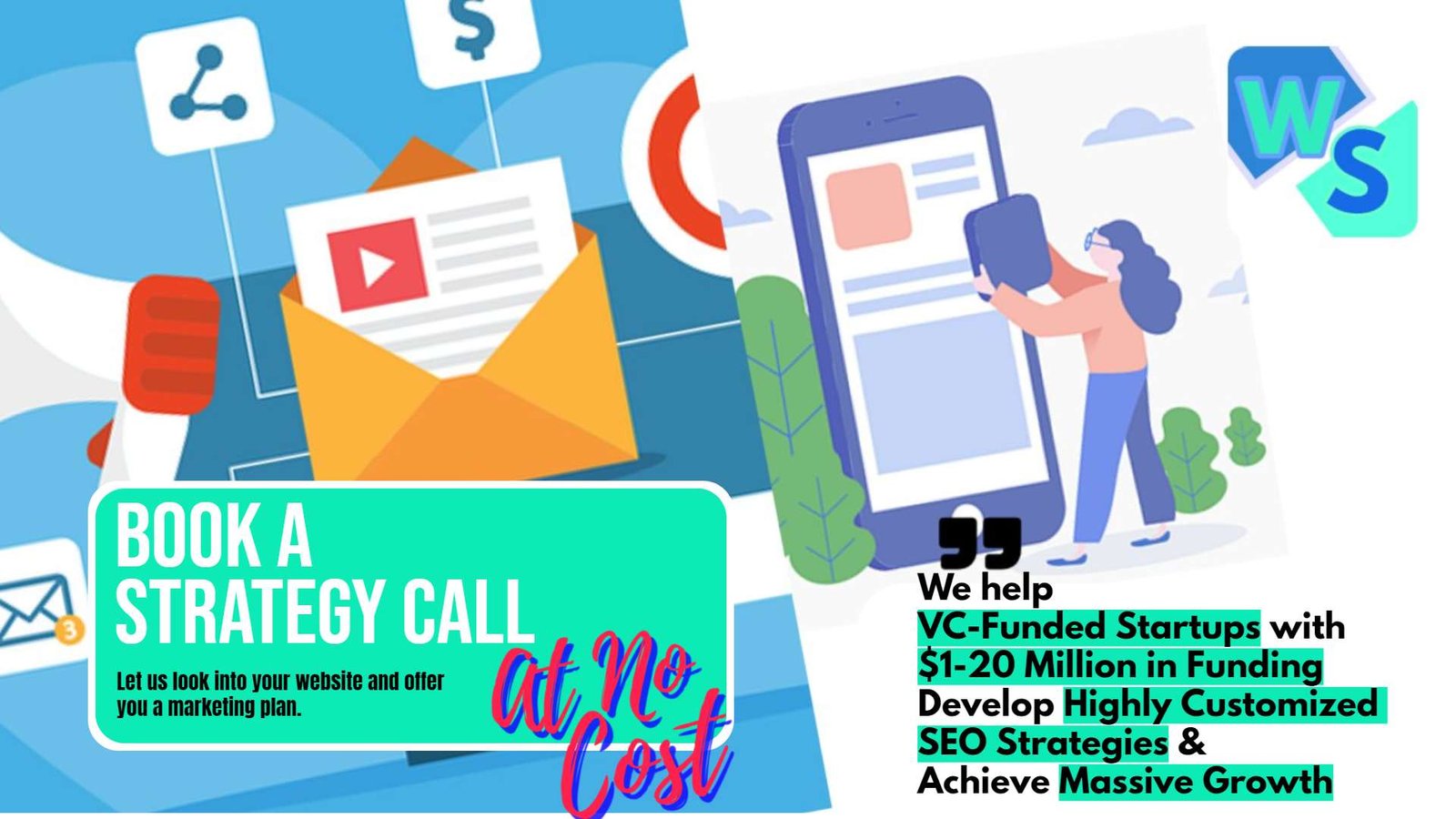
Related: Check out our free tools:
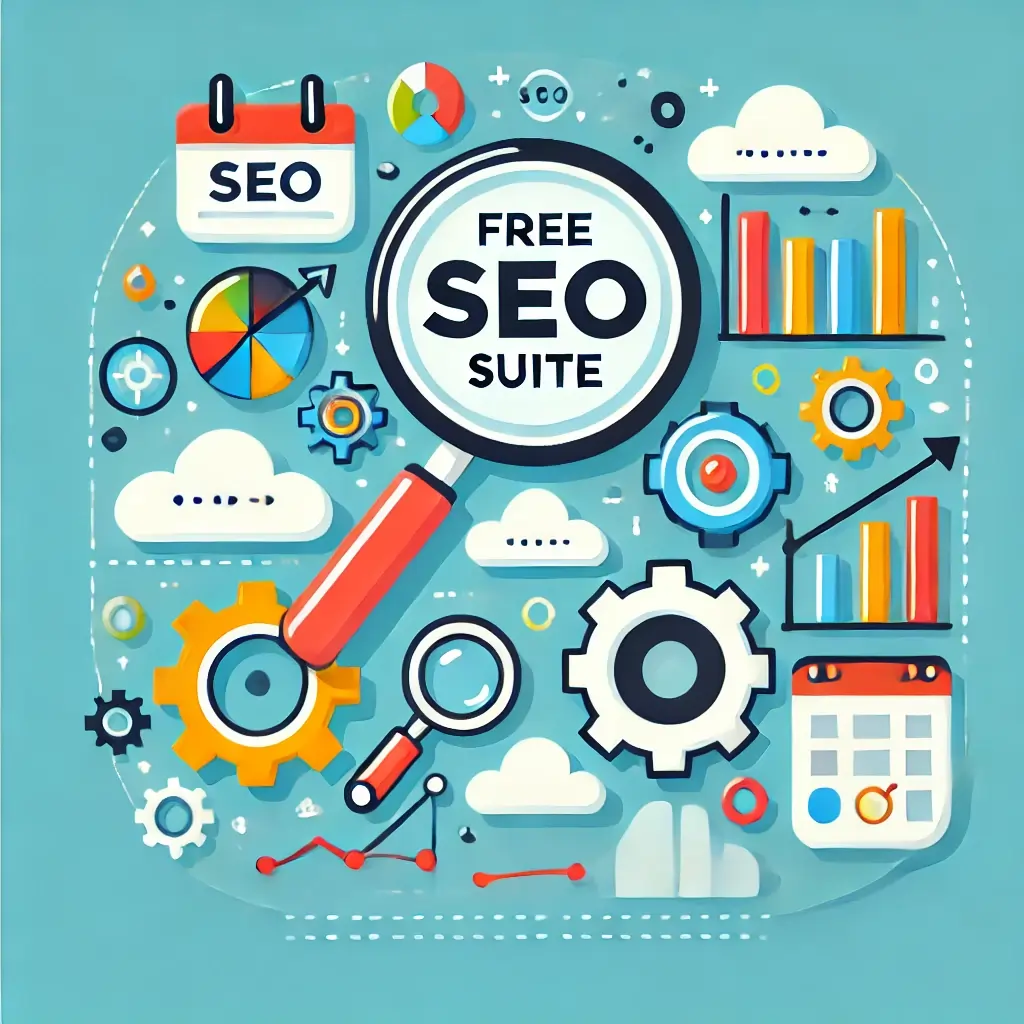
Enhancing Decision-Making with AI
Real-Time Analytics
One of the most powerful applications of AI in data analytics is real-time analytics. AI can process data as it is generated, providing immediate insights and enabling quick decision-making. For businesses, this means being able to respond to market changes, customer behaviors, and operational issues as they happen.
To leverage real-time analytics effectively, ensure that your AI systems are integrated with data sources that provide up-to-date information. Use AI to monitor key performance indicators (KPIs) and alert decision-makers to any significant changes or trends. For example, in retail, AI can analyze sales data in real-time to optimize inventory levels and adjust pricing strategies on the fly.
Predictive Analytics
Predictive analytics involves using AI to forecast future events based on historical data. This capability can help businesses anticipate customer needs, market trends, and potential risks. By making accurate predictions, companies can make proactive decisions that improve outcomes and reduce uncertainty.
Implement predictive analytics by training AI models on historical data relevant to your business goals. For example, a financial services company might use predictive analytics to forecast stock prices or credit risk.
Regularly update your models with new data to maintain their accuracy. Predictive analytics can also enhance customer relationship management (CRM) by identifying which customers are most likely to churn or make a purchase.
Prescriptive Analytics
While predictive analytics tells you what might happen, prescriptive analytics goes a step further by suggesting actions to achieve desired outcomes. AI can analyze data, predict future scenarios, and recommend the best course of action. This is particularly useful for complex decision-making processes where multiple factors must be considered.
To implement prescriptive analytics, combine predictive models with optimization algorithms. For instance, a logistics company could use prescriptive analytics to optimize delivery routes, considering factors like traffic, weather, and fuel costs. By recommending the most efficient routes, AI can reduce operational costs and improve service delivery.
Improving Customer Experiences with AI
Personalized Marketing
AI can significantly enhance marketing efforts by delivering personalized experiences to customers. By analyzing customer data, AI can identify individual preferences, behaviors, and purchase histories, allowing businesses to tailor their marketing messages and offers.
To use AI for personalized marketing, segment your customer base using AI-driven analytics. Create detailed customer profiles and use these insights to design targeted marketing campaigns.
For example, an e-commerce company can use AI to recommend products based on a customer’s browsing history and previous purchases. Personalized marketing not only improves customer satisfaction but also increases conversion rates and sales.
Customer Support Automation
AI-powered chatbots and virtual assistants are transforming customer support by providing instant, 24/7 assistance. These AI systems can handle a wide range of customer inquiries, from answering frequently asked questions to processing orders and troubleshooting issues. By automating routine support tasks, businesses can reduce response times and improve customer satisfaction.
To implement AI in customer support, integrate chatbots and virtual assistants into your website, mobile apps, and social media channels.
Ensure that these AI systems are trained with comprehensive knowledge bases to handle various customer queries effectively. Continuously update and refine your AI systems based on customer feedback and new information to maintain high service quality.
Sentiment Analysis
Sentiment analysis uses AI to interpret and analyze customer emotions expressed in text data, such as social media posts, reviews, and feedback. This helps businesses understand how customers feel about their products, services, and brand, enabling them to make data-driven improvements.
Implement sentiment analysis by integrating AI tools that can process and analyze large volumes of text data. Use these insights to gauge customer satisfaction, identify common pain points, and respond to negative feedback proactively. For example, a company can use sentiment analysis to monitor social media mentions and quickly address any emerging issues, maintaining a positive brand image.
Streamlining Operations with AI
Process Automation
AI can automate repetitive and time-consuming tasks, improving efficiency and reducing operational costs. By automating processes such as data entry, invoice processing, and inventory management, businesses can free up employees to focus on more strategic activities.
To implement process automation, identify routine tasks that can be automated and deploy AI tools to handle these processes. For example, a manufacturing company can use AI to automate quality control inspections, ensuring consistent product standards while reducing manual labor. Regularly review and optimize your automated processes to ensure they remain effective and aligned with business goals.
Supply Chain Optimization
AI can enhance supply chain management by providing real-time visibility, predictive analytics, and optimization capabilities. By analyzing data from various points in the supply chain, AI can identify inefficiencies, predict demand, and optimize inventory levels.
To use AI for supply chain optimization, integrate AI systems with your supply chain data sources. Use predictive analytics to forecast demand and plan inventory accordingly.
For instance, AI can help a retailer anticipate seasonal demand fluctuations and adjust stock levels to avoid overstocking or stockouts. Additionally, AI can optimize logistics and delivery routes, reducing costs and improving service delivery.
Workforce Management
AI can also improve workforce management by analyzing employee data and predicting staffing needs. This helps businesses optimize their workforce, ensuring they have the right number of employees with the right skills at the right time.
Implement AI in workforce management by analyzing historical data on employee performance, attendance, and workload. Use these insights to predict future staffing requirements and plan accordingly.
For example, a call center can use AI to forecast call volumes and schedule staff shifts to ensure adequate coverage during peak times. AI can also assist in talent acquisition by analyzing resumes and predicting candidate suitability for specific roles.
Ensuring Data Security and Privacy
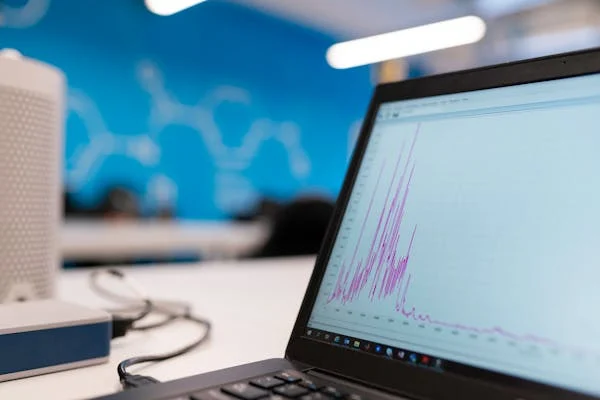
Implementing Robust Security Measures
With the increasing use of AI in data analytics, ensuring data security and privacy is more important than ever. Businesses must implement robust security measures to protect sensitive data from breaches and unauthorized access.
To ensure data security, use encryption to protect data at rest and in transit. Implement access controls to restrict data access to authorized personnel only. Regularly audit your security practices and update them as needed to address emerging threats. Additionally, ensure that your AI systems comply with relevant data protection regulations, such as GDPR or CCPA.
Ethical AI Practices
As AI becomes more integrated into business operations, ethical considerations must be addressed. This includes ensuring that AI systems are transparent, unbiased, and used responsibly. Ethical AI practices build trust with customers and stakeholders, fostering long-term business success.
To implement ethical AI practices, establish guidelines and frameworks for AI development and deployment. Ensure that your AI models are trained on diverse datasets to avoid bias.
Regularly review and audit your AI systems to ensure they adhere to ethical standards. Communicate transparently with customers about how their data is used and provide them with control over their information.
Data Privacy Compliance
Compliance with data privacy regulations is crucial for maintaining customer trust and avoiding legal penalties. Businesses must ensure that their AI systems comply with all relevant data privacy laws and regulations.
To ensure data privacy compliance, stay informed about the latest data protection regulations that apply to your business. Implement processes to manage and protect personal data, including obtaining consent from customers for data collection and processing. Regularly review and update your data privacy practices to ensure ongoing compliance.
Conclusion
Integrating AI into data analytics offers startups a powerful way to gain deeper insights, make data-driven decisions, and maintain a competitive edge in an increasingly data-centric world. By strategically approaching AI implementation, startup founders can unlock the full potential of their data and drive significant business growth.
Starting with a thorough needs assessment helps identify the most impactful use cases for AI within your organization. Prioritizing these use cases based on their potential ROI ensures that resources are allocated effectively. Setting measurable goals provides a clear framework for success and enables continuous monitoring and optimization of AI initiatives.
Read Next:
- Healthcare Marketing Statistics: Data-Driven Insights for 2024
- Experiential Marketing: Creating Impact with Key Statistics
- Text Marketing: Essential Statistics to Leverage in 2024
- Event Marketing in the Digital Age: Key Statistics for 2024
- The Future of Location-Based Marketing: Vital Statistics and Trends