Predictive analytics is reshaping the way businesses make decisions. By analyzing data and predicting future trends, companies can stay ahead of the competition and make smarter choices. At the heart of this revolution is artificial intelligence (AI). AI-driven predictive analytics tools are now essential for any business looking to thrive in today’s data-driven world. In this article, we’ll explore the best tools available and how they can help you harness the power of AI for predictive analytics.
Understanding Predictive Analytics
The Strategic Value of Predictive Analytics for Startups
For startup founders, predictive analytics can be a game-changer. It goes beyond just analyzing past performance to anticipate future trends and behaviors.
This foresight is crucial for startups that need to be agile and responsive to market dynamics. Predictive analytics helps in identifying opportunities, mitigating risks, and optimizing resources, which are all critical for the survival and growth of a new business.
A robust predictive analytics team should include data scientists, data engineers, and AI/machine learning engineers. Data scientists focus on building models and interpreting data to provide actionable insights, while data engineers handle the architecture, ensuring data is accessible, clean, and ready for analysis.
AI/machine learning engineers develop and fine-tune the algorithms that power predictive models. A business analyst with solid domain knowledge is essential to bridge the gap between tech and strategic business needs, ensuring outputs align with business goals.
Each member should be proficient in coding languages like Python or R and have a solid understanding of machine learning frameworks (e.g., TensorFlow, PyTorch). Strong problem-solving skills and familiarity with data visualization tools like Tableau or Power BI are essential for conveying insights. For startups, adaptability is vital—team members should be comfortable wearing multiple hats and pivoting as projects evolve.
Fostering continuous learning is vital in a startup environment like ours. This means investing in training resources and encouraging team members to stay updated on AI advancements. Collaboration is also critical; cross-functional work involving product teams and marketers ensures that predictive models address real business problems and drive user-focused outcomes.
Look beyond traditional resumes and seek candidates who demonstrate curiosity and a proven ability to learn quickly. Startups thrive on agility, so hiring individuals who have shown they can adapt and contribute across different stages of product development is a major plus.
Emphasize a culture where technical skills and strategic thinking go hand-in-hand, enabling your team to leverage AI tools to create insights and drive impactful, data-informed decisions.
As Founder and CEO of Zapiy.com, I’ve seen how essential the right team is for effective AI-driven predictive analytics. Here’s our approach to assembling and nurturing such a team in a startup environment.
Key Roles for Predictive Analytics
Data Scientist: Develops models and algorithms; expertise in machine learning, stats, and programming (Python, R) is crucial.
Data Engineer: Builds and maintains data infrastructure, optimizing ETL and databases; skills in SQL, Apache Spark, and cloud platforms (AWS, Google Cloud) are essential.
Machine Learning Engineer: Deploys models in production, focusing on scalability and reliability; strong in MLOps and model deployment tools.
Data Analyst: Interprets trends and validates model outputs, providing insights for business decisions.
Core Skills Needed
Programming and Data Skills: Proficiency in Python, SQL, and cloud platforms.
Statistics and Modeling: A solid grasp of statistical methods underpins accurate predictions.
Data Storytelling: Clear communication of insights to stakeholders is vital for actionable results.
Training and Upskilling
Continuous Learning: We invest in online courses and conferences to keep our team up-to-date.
Cross-Training: Data scientists and engineers learn each other’s basics, promoting flexibility.
Knowledge-Sharing: Weekly sessions encourage collaboration and best practices.
Collaboration in a Startup
Cross-Departmental Communication: Our team works closely with other departments to align predictive insights with business goals.
Agile Workflow: We use short sprints to iterate on models quickly, ensuring progress without stalling for perfection.
Hiring for AI Roles
Prioritize Versatility: Candidates with flexible skills and an ability to adapt quickly are ideal.
Cultural Fit: Passion for the mission is essential in a fast-paced startup.
Problem-Solving Mindset: We look for curiosity and innovative thinking beyond just technical skills.
By combining data science, engineering, and analytics roles with a collaborative culture and ongoing learning, we’ve built a predictive analytics team that thrives in a startup environment and drives impactful insights for our business.
Last quarter, we implemented basic AI tools to analyze our waste collection patterns. Instead of complex systems, we started with simple pattern recognition. The tool predicted collection needs with 85% accuracy just by analyzing three months of route data. This saved us from purchasing an expensive enterprise solution.
Real Implementation:
We use Python-based Prophet for waste volume forecasting. Despite having no data scientists, our operations team learned to use it effectively in two weeks. The results were immediate – we reduced unnecessary collections by 40% by better predicting which bins needed servicing.
The same tool helped us spot seasonal patterns we’d missed. For example, we discovered that office waste increases 25% two weeks before Christmas, not during the holiday week as we’d assumed. This insight helped us adjust staffing patterns.
Cost-Effective Approach:
Instead of full-scale analytics platforms, we use Google Colab with basic visualization tools. Total monthly cost: under £100. This setup has improved our route efficiency by 35% while requiring minimal technical expertise.
Professional Truth:
Start with analyzing one specific problem. We began with just route optimization and expanded from there.
For startups stepping into predictive analytics, MonkeyLearn is a tool worth mentioning. It’s designed for simplicity, helping smaller teams dive into analytics without needing deep technical knowledge. MonkeyLearn’s no-code setup allows startups to quickly analyze customer data in real time and spot trends that might otherwise be missed.
One example comes to mind: a startup used MonkeyLearn to sift through customer feedback and social media comments, creating models that flagged potential buying patterns. This insight let them fine-tune their marketing instantly, based on what their audience cared about most.
Tools like MonkeyLearn give startups the chance to embrace data-driven decisions right from the start, no data experts required. It’s really about making analytics something anyone on the team can dive into and use effectively, without the usual hurdles.
“Our start-up digital marketing agency has used a variety of AI tools with predictive analytics to help us scale our company and provide value to our clients.
The AI tools we’ve used include Seamless.ai for lead generation and outreach, Claude.ai for help with generating content marketing assets, CGDream.ai to help with editing multimedia content to be used in content marketing assets, and ChatGPT to help conduct research for the content marketing assets we create.
For example, we’ve prospected and sourced new leads using Seamless.ai, built out search engine optimized (SEO) marketing content using Claude.ai and SurferSEO, and sourced material for our content marketing assets using ChatGPT.
As a result, we’ve been able to onboard clients with consistency month over month for the last one and a half years.
Thanks to the above-mentioned AI tools, we’ve been more efficient at and productive in running our digital marketing start-up.“
Building a Predictive Analytics Framework
To leverage predictive analytics effectively, startup founders must establish a robust framework. This involves understanding the key components of predictive analytics: data collection, data preparation, model building, model validation, and deployment. Each component must be carefully managed to ensure that the predictive models deliver accurate and actionable insights.
Data collection is the foundation of predictive analytics. Startups should identify all potential data sources, including sales data, customer interactions, social media, market trends, and even third-party data. The more comprehensive the data, the better the predictive model will perform.
Data preparation involves cleaning and transforming the data to make it suitable for analysis. This step is crucial as poor-quality data can lead to inaccurate predictions. Startups should invest in tools and processes that automate data cleaning and preparation, ensuring that the data is accurate, complete, and up-to-date.
Model building is where the actual predictive analytics takes place. Using historical data, startups can create models that predict future outcomes. This requires selecting the right algorithms and techniques, which can be complex. Tools like RapidMiner and DataRobot can simplify this process by automating many of the technical tasks involved in model building.
Model validation ensures that the predictive models are accurate and reliable. This involves testing the models against new data to see how well they predict future outcomes. Startups should continuously validate and refine their models to maintain their accuracy over time.
Deployment is the final step, where the predictive models are integrated into business processes. This allows startups to use the predictions in real-time to inform decision-making. Continuous monitoring is essential to ensure that the models remain relevant and accurate as business conditions change.
Aligning Predictive Analytics with Business Goals
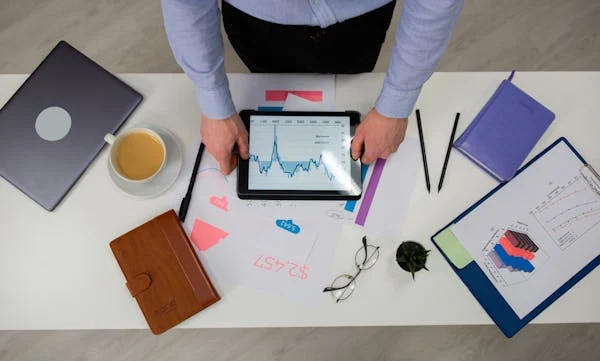
For predictive analytics to be truly effective, it must be aligned with the startup’s business goals. Founders should start by identifying the key performance indicators (KPIs) that matter most to their business.
These could be metrics related to sales growth, customer acquisition, churn rate, or operational efficiency. By focusing on these KPIs, startups can ensure that their predictive analytics efforts are directly contributing to their strategic objectives.
For example, if a startup’s goal is to increase customer retention, predictive analytics can help by identifying the factors that lead to churn. By analyzing historical data, the startup can predict which customers are most likely to leave and take proactive steps to retain them. This could involve personalized marketing campaigns, targeted promotions, or improved customer support.
Data-driven business landscape, integrating AI-driven predictive analytics into core business processes has become a crucial strategy for organizations seeking to gain a competitive edge. This powerful combination of artificial intelligence and data analysis allows companies to anticipate future trends, make informed decisions, and optimize their operations across various departments.
Predictive analytics harnesses the power of historical data, statistical algorithms, and machine learning techniques to forecast future outcomes. When integrated into core business processes, these insights can transform how companies approach marketing, operations, product development, and customer service, among other areas.
The result is a more agile, efficient, and forward-thinking organization capable of adapting to market changes and customer needs with unprecedented speed and accuracy.
Integrating AI-driven predictive analytics into core business processes can significantly enhance decision-making and drive operational efficiency.
Leveraging Predictive Analytics in business operations can help owners in multiple ways. In the healthcare IT industry, using AI-driven predictive analytics can boost product development, marketing campaigns, and other operations. In marketing, AI-based predictive analytics can help leaders segment customers, make predictions about market demands and trends, and even optimize ad campaigns.
I feel the best way to use AI to boost marketing is to integrate it into the company’s CRM software. This integration will help business owners or marketing leaders craft dynamic and targeted campaigns using customer behavioral data and other trends. Not just marketing, AI algorithms in predictive models can also effectively manage inventory and employees.
Integrating AI into ERP systems can prove to be quite effective in streamlining workflows, allocating resources, and reducing staff downtime. AI-driven predictive analytics can also facilitate better product development. In recent years, the product development team at my company has been leveraging AI tools and analytics to get actionable insights on market trends, designs, usability, feature optimization and feedback. Doing so has helped us in building a customer-oriented product.
While using AI-driven predictive analytics for marketing, product development, and operations can prove a great way to achieve business goals, it’s also essential to select the right set of tools and adopt best practices.
Opting for predictive analytics platforms that can easily integrate with CRM, SCM, and ERP systems should be a priority. Besides that, you should start small and then scale up. Integrating AI insights into dashboards and applications can be also helpful. Lastly, it’s crucial to establish a continuous improvement model through feedback loops from customers to ensure everything is running smoothly.
Leveraging Predictive Analytics for Competitive Advantage
In a competitive market, predictive analytics can provide a significant advantage. By anticipating market trends and customer behavior, startups can stay ahead of their competitors. This could involve identifying emerging market opportunities, optimizing pricing strategies, or improving product development processes.
For instance, a startup in the retail sector can use predictive analytics to forecast demand for different products. By understanding which products are likely to be popular in the coming months, the startup can optimize its inventory levels, reduce stockouts, and improve customer satisfaction.
Similarly, a tech startup can use predictive analytics to identify the features that customers are most likely to want in the next version of their software, allowing them to prioritize development efforts accordingly.
Integrating Predictive Analytics into Decision-Making
To fully realize the benefits of predictive analytics, startups must integrate it into their decision-making processes. This involves creating a data-driven culture where decisions are based on data and insights rather than intuition. Founders should lead by example, using predictive analytics to inform their strategic decisions and encouraging their teams to do the same.
One practical way to achieve this is by incorporating predictive analytics into regular business reviews and planning sessions. For example, during a quarterly business review, the startup could use predictive models to forecast sales for the next quarter and identify any potential risks or opportunities. This allows the team to make more informed decisions and adjust their strategies as needed.
Investing in the Right Skills and Tools
Successful implementation of predictive analytics requires the right skills and tools. Startups should invest in training their teams on the basics of data analysis and predictive modeling. This could involve hiring data scientists or providing existing employees with the necessary training and resources.
In terms of tools, startups should choose solutions that are scalable and easy to use. Platforms like DataRobot and RapidMiner are designed to be user-friendly, making it easier for startups to get started with predictive analytics without needing extensive technical expertise. Additionally, these tools often come with built-in automation features that can save time and reduce the complexity of building and deploying predictive models.
Measuring the Impact of Predictive Analytics
Finally, it’s important for startups to measure the impact of their predictive analytics efforts. This involves tracking the performance of predictive models and assessing how they contribute to business outcomes.
By continuously monitoring and evaluating their predictive analytics initiatives, startups can identify areas for improvement and ensure that they are getting the maximum value from their investment.
For example, a startup could measure the accuracy of its sales forecasts by comparing predicted sales to actual sales. If there are significant discrepancies, the startup can investigate the reasons and refine its models accordingly. By regularly reviewing the performance of their predictive analytics efforts, startups can ensure that they remain on track to achieve their business goals.
Key Benefits of AI-Driven Predictive Analytics
Tailoring Marketing Strategies
For startup founders, one of the most impactful applications of AI-driven predictive analytics is in tailoring marketing strategies. By analyzing customer data, AI can identify patterns and trends that indicate which marketing campaigns are most likely to succeed.
This allows startups to target their marketing efforts more effectively, reaching the right audience with the right message at the right time. For instance, predictive analytics can help determine the best time to launch a new product or the most effective channels for advertising, ensuring maximum ROI on marketing spend.
Optimizing Resource Allocation
Startups often operate with limited resources, making efficient resource allocation critical. AI-driven predictive analytics can provide insights into which areas of the business are likely to yield the highest returns. By forecasting future demand and identifying growth opportunities, startups can allocate their resources more strategically.
This might involve investing more in high-potential product lines, expanding into new markets, or scaling operations to meet anticipated demand. Optimizing resource allocation not only maximizes returns but also ensures that the startup remains agile and responsive to market changes.
Enhancing Product Development
Predictive analytics can significantly enhance product development processes. By analyzing customer feedback, market trends, and usage patterns, AI can identify unmet needs and suggest features that customers are likely to value. This enables startups to prioritize their development efforts and bring products to market that are more closely aligned with customer demands.
Additionally, predictive analytics can help in forecasting the success of new products, allowing startups to make data-driven decisions about product launches and iterations. This proactive approach to product development can reduce the risk of costly mistakes and accelerate time-to-market.
Streamlining Financial Forecasting
Accurate financial forecasting is crucial for startups to manage cash flow, secure funding, and plan for growth. AI-driven predictive analytics can provide more precise financial forecasts by analyzing historical data and identifying trends. This enables startups to anticipate revenue fluctuations, manage expenses, and make informed financial decisions.
For example, AI can predict seasonal variations in sales, helping startups prepare for periods of high or low demand. By improving the accuracy of financial forecasts, startups can enhance their financial stability and make more strategic investment decisions.
Improving Customer Retention
Retaining customers is often more cost-effective than acquiring new ones, making customer retention a key focus for startups. Predictive analytics can help by identifying customers who are at risk of churning and suggesting targeted retention strategies. By analyzing customer behavior, AI can detect early warning signs of dissatisfaction and recommend proactive measures, such as personalized offers or enhanced customer support.
This allows startups to address issues before they lead to churn, improving customer satisfaction and loyalty. Enhanced customer retention not only boosts revenue but also builds a loyal customer base that can provide valuable referrals and feedback.
As the Founder and CEO of Zapiy.com, I’ve seen firsthand how AI-driven predictive analytics can dramatically improve customer retention. Let me share some insights and examples of how we and other startups have leveraged this technology to better understand our customers, predict churn, and drive personalized retention efforts.
1. Using Predictive Analytics to Identify At-Risk Customers
One of the most effective applications of predictive analytics for retention is identifying customers who may be on the verge of leaving. At Zapiy.com, we use predictive models that analyze behavioral data to detect signs of disengagement. For example, we track metrics such as login frequency, time spent on the platform, interaction patterns, and support tickets raised.
Tools and Techniques: We employ machine learning models built with tools like Python’s Scikit-Learn and TensorFlow to analyze these behavioral indicators. These models help us create a “churn risk score” for each user. We also leverage platforms like Tableau for data visualization, which helps us monitor these metrics in real time and allows non-technical team members to interact with the data.
Metrics Tracked: To spot potential churn, we focus on KPIs such as reduced product usage, declining engagement over a certain period, and negative feedback scores. We set thresholds that, when crossed, automatically alert our customer success team. These alerts allow us to initiate personalized retention efforts promptly.
2. Personalized Retention Strategies Through Segmentation
Another way predictive analytics has transformed our retention approach is by helping us segment customers more precisely. Using clustering techniques, such as k-means clustering, we can divide our user base into segments based on usage patterns, engagement levels, and preferences.
Practical Example: Let’s say one segment we identify consists of users who sign up but drop off quickly due to a lack of engagement with advanced features. For this group, we use automated campaigns to guide them through key features, like product tours, and even offer personalized onboarding sessions. Another segment may be high-value users who aren’t fully exploring new features—so for them, we highlight advanced functionalities that might enhance their experience.
Tools and Techniques: Beyond clustering, we use A/B testing to validate the effectiveness of these targeted campaigns. Tools like HubSpot and Intercom enable us to customize messages based on the segment, making our outreach more relevant.
3. Predicting Retention-Boosting Features and Content
Predictive analytics also informs us which features and content drive the most engagement, helping us allocate resources effectively to boost retention.
Case Example: When analyzing customer behavior, we noticed that users who engaged with our educational content—like webinars and tutorials—showed a higher retention rate. By deploying a model that predicted content interaction likelihood, we could nudge new users towards these resources early in their journey, making it more likely that they’d find value in our product and stick around.
Outcome: After implementing these content-focused recommendations, we observed a 20% increase in retention within the targeted group. This insight guided our strategy to produce even more relevant content and make it easily accessible for new users.
4. Measuring the Impact and Outcomes
It’s essential to quantify the impact of these retention efforts. We track customer retention rate, lifetime value (LTV), and net promoter score (NPS) as our key metrics for measuring success.
Results: By continuously fine-tuning our predictive models, we’ve been able to reduce our churn rate by approximately 25% over the last year. More importantly, the average lifetime value of our customers has grown, as we’re now able to engage and retain users much more effectively.
5. Real-Time Feedback Loops for Continuous Improvement
Finally, predictive analytics has enabled us to establish real-time feedback loops. Each retention campaign’s performance feeds back into our models, helping them become more accurate over time.
Continuous Improvement: Tools like Amazon SageMaker make this iterative model refinement more manageable, allowing us to retrain models as we gather more data on user interactions. By updating our algorithms regularly, we ensure they stay relevant and accurate as user behaviors shift.
In summary, predictive analytics has been a game-changer in our approach to customer retention at Zapiy.com. It enables us to pinpoint at-risk customers, tailor our outreach, and continually improve retention strategies through data-driven insights.
This approach doesn’t just help us retain more customers—it allows us to build stronger relationships with them, creating a loyal and engaged user base that drives long-term success.
I’ve seen how AI-driven predictive analytics can help keep customers. One example is when we used a predictive tool to analyze customer behavior and spot clients at risk of leaving.
We used machine learning algorithms and data analytics platforms. The tool gathered data from customer interactions, service usage, and feedback. By analyzing this, we could predict which customers might cancel.
In Key Metrics Tracked we focused on:
- How often customers used our services.
- How regularly customers used their security systems.
- Ratings and comments from surveys.
- A risk score for each customer’s likelihood of leaving.
Using predictive analytics, we found customers who seemed disengaged. For example, clients who hadn’t opened their security app in over a month were more likely to leave. With this information, we created personalized retention strategies, like outreach campaigns with discounts or extra services. We also reached out to these clients to address any concerns.
As a result, we improved customer retention by 15% in six months. This boosted revenue and customer satisfaction, as clients appreciated our proactive approach.
Predictive analytics has been a powerful tool, helping us identify at-risk customers and adapt our strategies to keep them. This experience has underscored the value of data-driven decisions in our business.
Accelerating Data-Driven Culture
Implementing AI-driven predictive analytics can accelerate the adoption of a data-driven culture within a startup. When founders and teams see the tangible benefits of data-driven decision-making, they are more likely to embrace data and analytics in other areas of the business.
This cultural shift can lead to more informed decisions across the organization, from marketing and sales to operations and finance. A data-driven culture fosters continuous improvement, innovation, and a focus on measurable results, all of which are essential for long-term success in a competitive market.
Facilitating Investor Relations
For startups seeking investment, demonstrating the use of advanced technologies like AI-driven predictive analytics can be a significant advantage. Investors are more likely to be impressed by startups that leverage data to drive decisions and optimize performance.
By showcasing predictive analytics capabilities, startups can provide investors with more accurate financial projections, detailed market insights, and evidence of a proactive approach to risk management. This transparency and foresight can build investor confidence and improve the likelihood of securing funding.
Supporting Sustainable Growth
Sustainable growth is a key objective for any startup, and predictive analytics plays a crucial role in achieving it. By providing insights into market trends, customer preferences, and operational efficiencies, AI-driven predictive analytics helps startups grow in a controlled and manageable way.
This ensures that growth is not only rapid but also sustainable, with a strong foundation built on data-driven decisions. Startups can avoid the pitfalls of overexpansion or underinvestment by continuously monitoring key metrics and adjusting their strategies based on predictive insights.
Enhancing Competitive Advantage
In today’s fast-paced business environment, maintaining a competitive edge is essential for startups. AI-driven predictive analytics can provide that edge by enabling faster and more informed decision-making.
Startups can anticipate market shifts, respond to customer needs more effectively, and optimize their operations to stay ahead of competitors. The ability to act on insights before competitors do can be a significant differentiator, allowing startups to capture market share and establish themselves as leaders in their industry.
Fostering Innovation
Innovation is at the heart of every successful startup. Predictive analytics fosters innovation by identifying emerging trends and opportunities that may not be immediately apparent. By continuously analyzing data and generating insights, AI encourages startups to experiment with new ideas, products, and strategies.
This culture of innovation can lead to the development of unique solutions that set the startup apart from competitors. Moreover, predictive analytics can help validate innovative ideas by forecasting their potential impact, reducing the risk associated with experimentation.
Essential Tools for AI-Driven Predictive Analytics
Google Cloud AI Platform
Google Cloud AI Platform is a comprehensive suite of machine learning tools designed to help businesses build, deploy, and manage predictive models. For startup founders, leveraging this platform can provide a significant advantage.
The AI Platform offers seamless integration with other Google Cloud services, making it easier to manage data and scale operations as the business grows. Its pre-built algorithms and flexible infrastructure allow startups to quickly develop and deploy models, saving time and resources.
Amazon SageMaker
Amazon SageMaker is another powerful tool for AI-driven predictive analytics. It simplifies the process of building, training, and deploying machine learning models. SageMaker’s integrated Jupyter notebooks offer a collaborative environment for data scientists and developers, making it ideal for startups that require team-based model development.
Its managed infrastructure handles the heavy lifting of machine learning, allowing startups to focus on refining their models and interpreting insights. SageMaker’s scalability ensures that as your startup grows, your predictive analytics capabilities can expand seamlessly.
BigML
BigML provides a user-friendly interface for creating and deploying machine learning models, making it accessible for startups with limited technical expertise. Its platform supports a wide range of predictive analytics tasks, including classification, regression, time-series forecasting, and anomaly detection.
For startup founders, BigML’s ease of use and rapid deployment capabilities mean quicker insights and faster decision-making. The platform’s ability to handle complex datasets and provide clear visualizations of results makes it a valuable tool for driving strategic business decisions.
TIBCO Data Science
TIBCO Data Science offers a robust platform for predictive analytics that combines data preparation, machine learning, and model management. For startups, TIBCO’s platform provides the flexibility to integrate with various data sources and the power to process large volumes of data efficiently.
Its visual analytics and intuitive interface make it easier for non-technical users to understand and leverage predictive insights. By using TIBCO Data Science, startups can accelerate their data-to-insight journey, enabling more agile and informed decision-making.
Qlik Sense
Qlik Sense is a self-service analytics tool that enables startups to explore and analyze data interactively. Its associative engine allows users to explore data without predefined queries, uncovering hidden insights and relationships.
For startup founders, Qlik Sense offers the ability to quickly generate dashboards and reports that can inform strategic decisions. Its real-time data processing capabilities ensure that insights are always up-to-date, helping startups stay agile and responsive to market changes.
Tableau
Tableau is renowned for its powerful data visualization capabilities, making it an essential tool for startups looking to harness predictive analytics. By integrating with various data sources, Tableau allows startups to create interactive and shareable dashboards.
These visualizations make it easier to interpret complex data and communicate insights across the organization. For founders, Tableau’s ability to quickly turn data into actionable insights supports more effective strategic planning and operational execution.
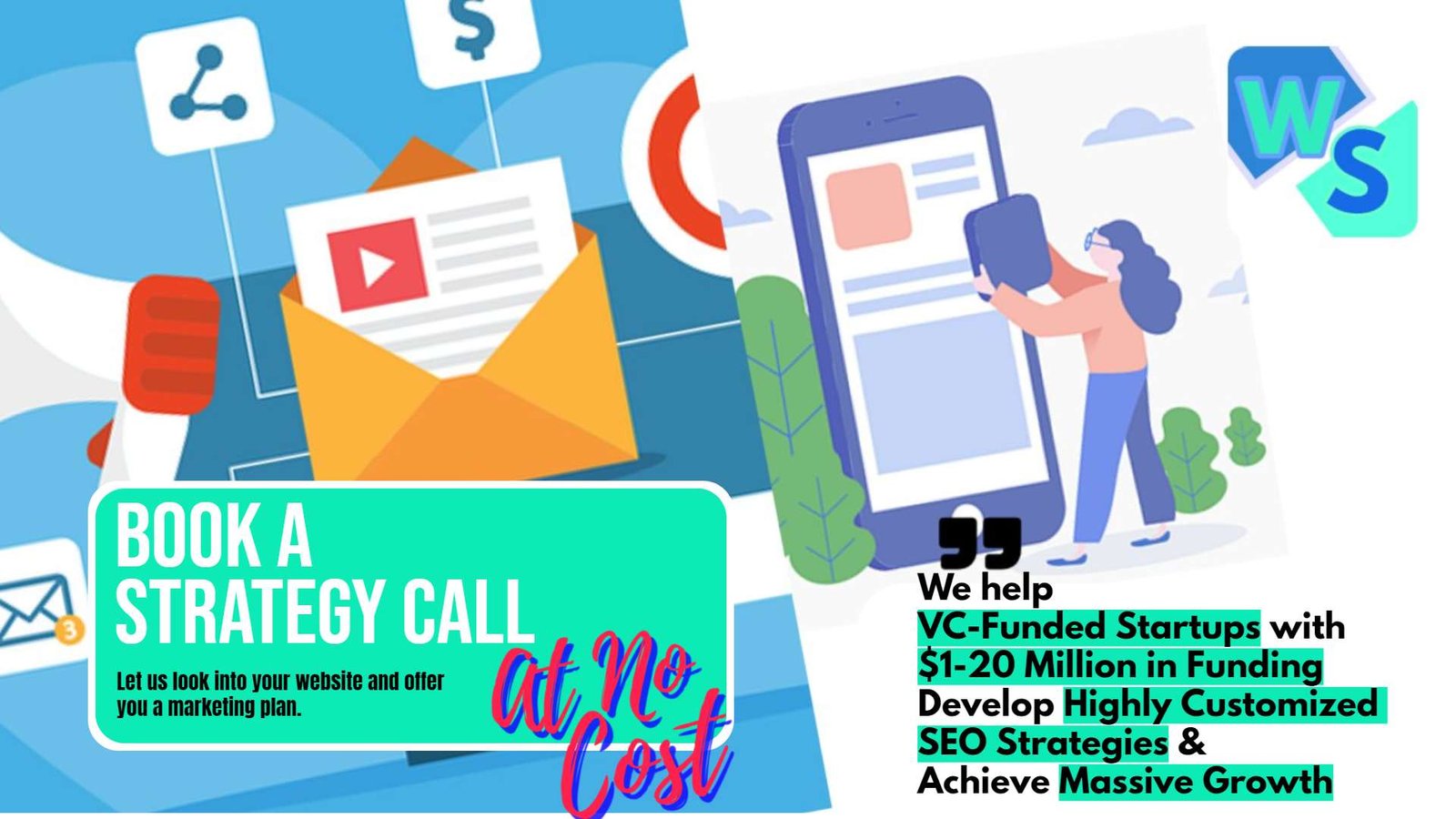
Related: Check out our free tools:
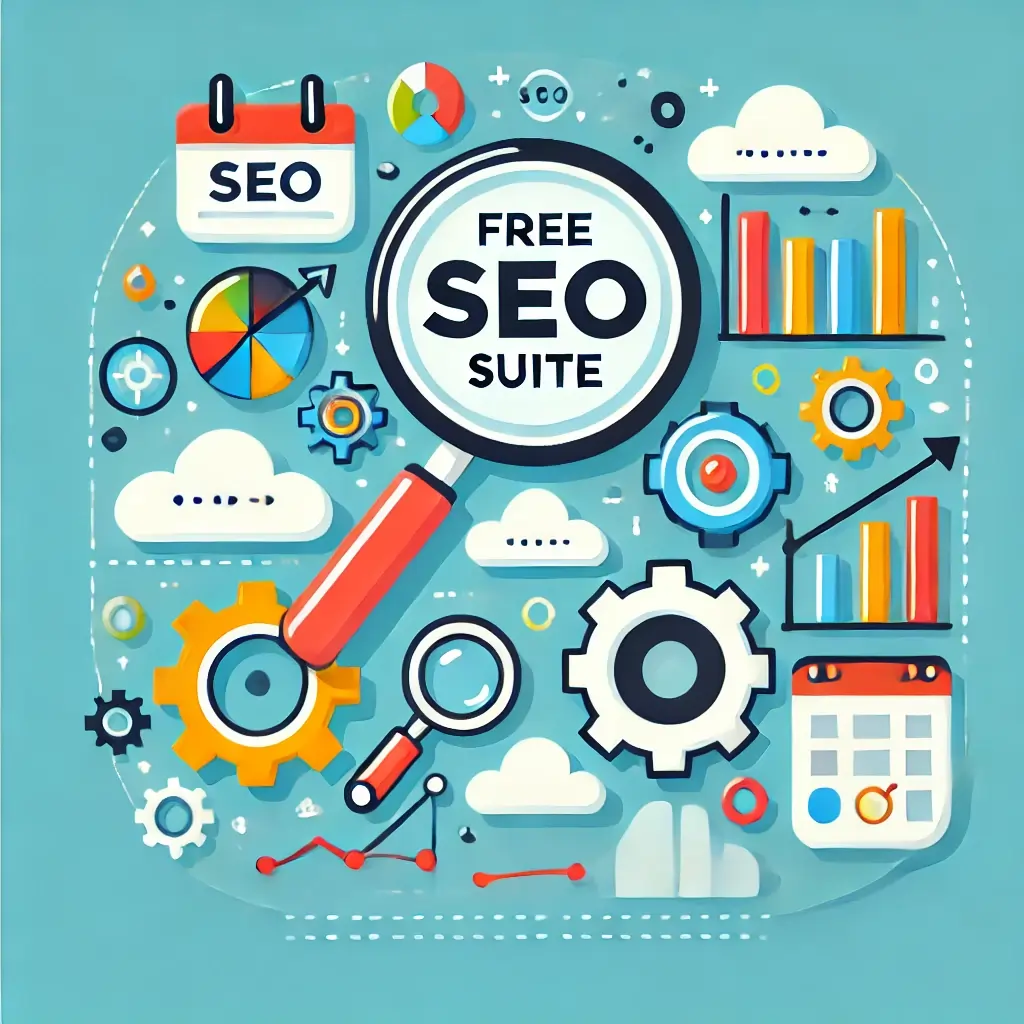
Zoho Analytics
Zoho Analytics is a business intelligence and analytics platform that offers robust predictive analytics features. It supports data integration from multiple sources, allowing startups to create a unified view of their data.
Zoho’s AI-driven analytics engine can perform predictive modeling, helping startups forecast trends and make data-driven decisions. Its collaborative features enable teams to share insights and work together on data analysis, fostering a data-centric culture within the startup.
Domino Data Lab
Domino Data Lab is an enterprise-grade platform designed for collaborative data science. It supports the entire predictive analytics lifecycle, from data preparation and model development to deployment and monitoring. For startups, Domino’s collaborative environment can enhance team productivity and innovation.
Its integration with popular data science tools and cloud services ensures flexibility and scalability. By using Domino, startups can streamline their predictive analytics workflows and accelerate their time-to-value.
Looker
Looker is a data analytics platform that offers powerful tools for predictive analytics. Its LookML language allows startups to create custom data models tailored to their specific needs.
Looker’s integration with cloud databases and its ability to handle large datasets make it a robust solution for startups aiming to scale their predictive analytics efforts. For founders, Looker provides the ability to build and share insightful dashboards that drive data-driven decision-making across the organization.
Snowflake
Snowflake’s cloud data platform is designed for high-performance data analytics, including predictive modeling. Its architecture allows for seamless integration with machine learning tools and supports real-time data processing.
For startups, Snowflake offers the scalability and flexibility needed to handle growing data volumes and complex analytics tasks. By leveraging Snowflake, startups can ensure that their predictive analytics infrastructure is robust and future-proof, supporting long-term growth and innovation.
Integrating Tools for Maximum Impact
To maximize the impact of these tools, startup founders should consider integrating them into a cohesive predictive analytics ecosystem.
This involves selecting tools that complement each other and provide end-to-end capabilities, from data collection and preparation to model deployment and monitoring. By creating a seamless workflow, startups can ensure that their predictive analytics processes are efficient and effective.
For example, a startup could use Google Cloud AI Platform for model development, Snowflake for data storage, and Tableau for visualization. This combination allows for robust data management, advanced analytics, and clear communication of insights. The key is to ensure that the chosen tools integrate well with each other and align with the startup’s specific needs and objectives.
Building a Predictive Analytics Team
Successful implementation of AI-driven predictive analytics requires a skilled team. Startup founders should focus on building a team that includes data scientists, data engineers, and business analysts. Each role brings a unique set of skills essential for the different stages of predictive analytics.
Data scientists develop and refine models, data engineers ensure the infrastructure and data pipelines are robust, and business analysts interpret insights and drive strategic decisions.
Investing in ongoing training and development for the team is crucial. The field of AI and predictive analytics is rapidly evolving, and staying updated with the latest techniques and tools will ensure that the startup remains competitive. Encouraging a culture of continuous learning and experimentation can lead to innovative solutions and improved business outcomes.
Fostering Collaboration and Communication
Predictive analytics is most effective when insights are shared and acted upon across the organization. Startup founders should foster a collaborative environment where data and insights are accessible to all relevant stakeholders. Using tools that support collaboration, such as Domino Data Lab and Qlik Sense, can facilitate this process.
Regular meetings and workshops focused on data insights can help ensure that predictive analytics is integrated into the startup’s strategic planning and daily operations. By creating a feedback loop where insights are continuously refined and acted upon, startups can maximize the value of their predictive analytics efforts.
Ensuring Ethical Use of AI
As startups harness the power of AI-driven predictive analytics, it’s essential to consider the ethical implications. This includes ensuring data privacy, avoiding biases in models, and maintaining transparency in how AI is used. Founders should establish clear guidelines and practices for ethical AI use, including regular audits and reviews of predictive models.
By prioritizing ethical considerations, startups can build trust with their customers, investors, and other stakeholders. This trust is crucial for long-term success and sustainability in the increasingly data-driven business environment.
Getting Started with AI-Driven Predictive Analytics
Establish a Clear Vision and Goals
The first step for startup founders in getting started with AI-driven predictive analytics is to establish a clear vision and set specific, measurable goals. Understand what you aim to achieve with predictive analytics and how it aligns with your overall business strategy.
Whether it’s improving customer retention, optimizing marketing efforts, or enhancing operational efficiency, having a well-defined objective will guide your efforts and ensure that your initiatives are focused and impactful.
Invest in Data Quality and Management
High-quality data is the backbone of effective predictive analytics. Startups must prioritize data management practices that ensure the accuracy, completeness, and timeliness of their data.
This involves implementing robust data governance frameworks and investing in data cleaning and validation processes. Tools like Alteryx and TIBCO Data Science can help automate these tasks, reducing the burden on your team and ensuring that your predictive models are built on reliable data.
Start with a Pilot Project
To mitigate risks and demonstrate the value of predictive analytics, start with a pilot project. Choose a specific area of your business where predictive analytics can make a significant impact and where you have sufficient data.
This could be a marketing campaign, sales forecasting, or inventory management. By starting small, you can refine your approach, address any challenges, and build a solid foundation before scaling your efforts.
Assemble a Cross-Functional Team
Building a successful predictive analytics initiative requires a cross-functional team that includes data scientists, data engineers, business analysts, and domain experts. Each team member brings unique skills and perspectives that are essential for developing and implementing predictive models.
Encourage collaboration and ensure that everyone understands the project’s objectives and their role in achieving them. Regular communication and coordination will help align efforts and foster a sense of shared ownership.
Leverage Open Source and Cloud-Based Tools
For startups, leveraging open-source and cloud-based tools can provide cost-effective solutions for predictive analytics. Platforms like H2O.ai, KNIME, and Google Cloud AI Platform offer powerful capabilities without the need for significant upfront investment.
These tools are often scalable and can grow with your business, providing flexibility as your predictive analytics needs evolve. Additionally, cloud-based solutions reduce the need for extensive on-premises infrastructure, making it easier to get started quickly.
Develop and Iterate Models
Developing predictive models is an iterative process. Start by selecting the right algorithms and building initial models using historical data. Validate these models to ensure they provide accurate predictions. Use tools like DataRobot and RapidMiner to automate and streamline this process.
Once your models are validated, deploy them in a controlled environment and monitor their performance. Continuously refine and update your models based on new data and feedback to improve their accuracy and relevance.
Integrate Predictive Analytics into Business Processes
For predictive analytics to deliver real value, it must be integrated into your core business processes. This means embedding predictive models into decision-making workflows and ensuring that insights are easily accessible to those who need them.
Use visualization tools like Tableau and Qlik Sense to create intuitive dashboards that present predictive insights in a clear and actionable format. Ensure that your team is trained to interpret and act on these insights, fostering a data-driven decision-making culture across the organization.
Monitor Performance and Measure Impact
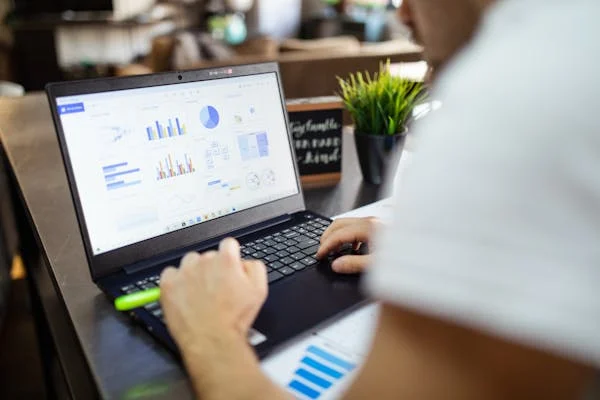
Monitoring the performance of your predictive models is crucial for ensuring their ongoing effectiveness. Establish key performance indicators (KPIs) that align with your business goals and regularly track these metrics. Use tools that provide real-time monitoring and alerting to identify any deviations or issues promptly.
Additionally, measure the impact of your predictive analytics initiatives on business outcomes. This could include improvements in sales, cost savings, enhanced customer satisfaction, or other relevant metrics. By demonstrating tangible results, you can build support for expanding your predictive analytics efforts.
Foster a Culture of Continuous Improvement
The field of AI and predictive analytics is constantly evolving. To stay competitive, startups must foster a culture of continuous improvement. Encourage your team to stay updated with the latest developments, attend industry conferences, and participate in training programs.
Experiment with new techniques and tools to keep your predictive models at the cutting edge. By maintaining a learning mindset and continuously seeking ways to enhance your predictive analytics capabilities, you can drive ongoing innovation and growth.
Secure Executive Buy-In and Support
For predictive analytics initiatives to succeed, it’s essential to have strong executive buy-in and support. Founders and senior leaders must champion the use of predictive analytics and provide the necessary resources and support. Communicate the strategic value of predictive analytics and its potential impact on the business.
Regularly update executives on progress and successes, using data and tangible results to demonstrate the benefits. This top-down support will help ensure that predictive analytics is prioritized and integrated into the startup’s strategic initiatives.
Prioritize Ethical Considerations and Compliance
As you implement AI-driven predictive analytics, it’s crucial to prioritize ethical considerations and ensure compliance with relevant regulations. This includes protecting customer data privacy, avoiding bias in predictive models, and maintaining transparency in how AI is used.
Establish clear policies and guidelines for ethical AI use and ensure that your team adheres to them. Regular audits and reviews can help identify and address any ethical or compliance issues, building trust with customers and stakeholders.
In predictive analytics, data privacy compliance is foundational. Startups must prioritize data anonymization and minimize data collection to what is strictly necessary, thus reducing exposure risk. Compliance frameworks like GDPR and CCPA serve as strong guides even for companies operating outside their primary jurisdictions.
Regular data audits, conducted quarterly or semi-annually, help ensure ongoing adherence to these standards. In our platform, we also employ encryption across data touchpoints, a simple yet powerful measure that safeguards sensitive information.
Avoiding Bias in Predictive Models
Bias in predictive models often arises from unbalanced data or unchecked assumptions within algorithms. Startups can mitigate this by adopting a rigorous approach to data selection, ensuring that training datasets are representative of the broader population and free of overrepresentation in any particular category.
Establishing Ethical Guidelines for Predictive Analytics
Ethical guidelines for predictive analytics should address data handling practices, transparency, and accountability. Startups are well-advised to form a small ethics committee or advisory group to oversee AI use and help set guidelines tailored to their specific industry. Clear documentation of model decisions and data sources adds a layer of transparency that can be shared with stakeholders and used for internal reviews.
Our approach has been to treat transparency as a cornerstone; by making our model decision pathways visible to clients, we foster trust and demonstrate a commitment to fairness and ethical rigor.
Conducting Regular Audits for Ethical Compliance
Regular audits are essential to monitor compliance and adapt to new regulatory standards or ethical challenges. An audit should assess the entire lifecycle of data, from collection to application, identifying any potential for bias or privacy risks.
For startups with limited resources, starting with smaller, monthly audits focused on high-impact areas, followed by more comprehensive quarterly reviews, is a manageable yet effective approach. Incorporating automated audit tools can also streamline this process and ensure consistent oversight without requiring large staff resources.
As the Founder and CEO of Zapiy.com, I am acutely aware of the ethical considerations and potential pitfalls when using AI-driven predictive analytics. Predictive analytics can be an incredible tool for growth, but without careful planning, it can also bring significant risks, especially around privacy and bias. Let me walk you through how we approach these issues at Zapiy.com.
1. Data Privacy and Compliance
Data privacy is foundational to ethical AI. One of our first priorities is ensuring that our data handling practices align with regulations like GDPR, CCPA, and others that aim to protect individual privacy. Here’s a practical rundown of what we do to stay compliant:
Data Minimization: We limit data collection to what’s absolutely necessary. We ensure our models work effectively without hoarding unnecessary personal data, reducing exposure and risk.
Informed Consent: We’re transparent with users about what data we collect and why. By providing clear opt-in and opt-out options, we give users control over their personal data and build trust.
Anonymization: Wherever possible, we anonymize data. Even if data is breached, anonymized information mitigates the risk of personally identifiable information being exposed.
Regular Compliance Checks: Compliance isn’t a one-time effort, especially as regulations evolve. We conduct frequent checks and audits to ensure our practices align with current data protection standards, and we also consult legal experts periodically.
2. Avoiding Bias in Predictive Models
Bias is another significant ethical concern, particularly when it comes to model training and outcomes. Bias can often be hidden within historical data or inadvertently introduced during the training process. Here’s how we address this:
Diverse Data Sources: We make sure our data represents a diverse set of perspectives. This isn’t just about broadening demographic categories; it’s about ensuring that each group is adequately represented.
Bias Testing and Mitigation: Regularly testing our models for bias is essential. We do this by examining outcomes across different demographics to ensure fair and accurate predictions. If we detect biases, we take corrective actions, such as re-training models or adjusting feature weights.
Transparency in Algorithms: Where feasible, we lean toward models that can provide explainability, allowing us to understand how specific features impact predictions. This transparency helps us identify any underlying bias or misrepresentation.
3. Establishing Ethical Guidelines
We’ve found that establishing an ethical framework is crucial for guiding decisions throughout the lifecycle of an AI project. Our ethical guidelines include:
Clear Documentation: We document the purpose, methods, and limitations of our models. This documentation isn’t only for the development team but for everyone in the organization, helping us stay aligned on ethical practices.
Human Oversight: AI shouldn’t operate in a vacuum. We have human oversight at critical checkpoints, and our team frequently reviews predictions and decisions to ensure they align with our ethical standards.
Transparent Communication with Users: Whenever we use predictive analytics to make user-facing decisions, we make sure users understand how these decisions are made. This transparency builds accountability and helps us course-correct if there are any misunderstandings.
4. Conducting Regular Audits
Predictive models aren’t static, so regular audits are a non-negotiable part of our process. Here’s how we manage it:
Routine Performance Checks: We conduct regular audits of our predictive models, focusing on accuracy, fairness, and compliance. This helps us ensure that model predictions continue to be accurate and fair over time.
Third-Party Reviews: Bringing in external experts provides an objective view of our models and processes. This extra layer of scrutiny helps us identify and address potential ethical issues that may not be apparent internally.
Updating Models and Training Data: Data shifts over time, and so do societal standards. We revisit our training data and retrain our models regularly, ensuring they remain relevant and unbiased.
At Zapiy.com, we view ethical AI as a commitment rather than a constraint. As our models evolve, so do our strategies for ensuring they remain fair, transparent, and aligned with both regulatory and ethical standards. With a thoughtful approach, startups can harness the power of predictive analytics responsibly, turning ethics into a core strength, not just an obligation.
1. Prioritize Data Privacy and Compliance from the Outset
Startups must ensure that data collection and processing meet privacy laws, like GDPR and CCPA. To achieve this, consider the following steps:
– Data Minimization and Anonymization: Collect only what’s necessary for the model. Anonymize data wherever possible. Anonymization reduces the risks of exposing sensitive information and can simplify compliance.
– Consent Management: Get users’ consent before using their data. They must understand how it will be used in predictive models. Transparent data practices foster trust and help align with regulatory requirements.
– Regular Privacy Audits: Conduct audits to check data handling, storage security, and model outputs. Audits should confirm regulation adherence and reveal any gaps in data privacy management.
2. Addressing Bias and Fairness in Predictive Models
AI models are only as unbiased as the data on which they are trained. Biased data can lead to skewed predictions, impacting users or stakeholders unfairly. To mitigate bias, startups should:
– Diverse Data Sourcing: Use diverse datasets to train models and reduce the risk of inherent biases. Including data from varied demographics and perspectives makes models more representative and fair.
– Algorithmic Audits and Bias Testing: Regularly test models for potential output bias. Audit algorithms. Check predictions for bias or unfairness. Then, adjust them to improve fairness.
– Transparency in Model Design: Clear docs on the model’s design, data, and training allow for better scrutiny and accountability. Transparent models also make it easier to communicate ethical considerations with stakeholders.
3. Establishing Ethical Guidelines for Predictive Analytics
Developing ethical guidelines that govern predictive analytics is vital for maintaining responsible practices. These guidelines should include:
– Ethical Use Policies: Outline policies on acceptable and unacceptable predictive analytics uses. Define use cases that prioritize user welfare and mitigate potential harms.
– Stakeholder Involvement: Engage stakeholders in creating and reviewing ethical guidelines, including data privacy experts and affected community members. This collaborative approach can help reveal blind spots and increase accountability.
– Ongoing Training and Awareness: Train teams on data ethics and best practices for AI model development. Regular workshops and training sessions reinforce the importance of ethical considerations.
4. Continuous Monitoring and Audits for Ethical Compliance
Ethics in AI is an evolving field requiring continuous evaluation and updates. Conducting frequent audits helps ensure models remain aligned with ethical standards. Also, set up a way for users to report biases or issues. This will let you address concerns as they arise.
Ethical issues in AI-driven predictive analytics require:
- Careful data handling.
- Strong bias-mitigation practices.
- Regular audits.
Startups can create responsible and effective predictive models by:
- Adhering to data privacy regulations.
- Fostering model transparency.
- Implementing ethical solid guidelines.
The ethical implementation of predictive analytics requires a comprehensive framework that addresses privacy, bias, and transparency throughout the analytics lifecycle. Organizations must establish robust data governance protocols that ensure compliance with evolving privacy regulations while maintaining the utility of their predictive models.
This includes implementing strong data anonymization techniques, establishing clear data retention policies, and maintaining transparent communication with stakeholders about how their data is used.
Addressing bias in predictive models requires continuous vigilance and systematic testing approaches. Successful organizations implement regular bias audits that examine model outputs across different demographic groups and business scenarios.
These audits should be complemented by diverse development teams who can identify potential bias issues early in the model development process and implement appropriate mitigation strategies.
The establishment of ethical guidelines for predictive analytics should be a collaborative process involving stakeholders from across the organization. These guidelines should address not only technical aspects of model development but also the broader societal implications of predictive analytics applications. Regular reviews and updates of these guidelines ensure they remain relevant as technology evolves and new ethical considerations emerge.
Harmeet is an IT and Audiovisual leader at the forefront of workplace transformation. Harmeet drives operational excellence and innovation in end-user support at a global level. With a diverse background spanning corporate IT, hybrid broadcast/event production and entertainment management, Harmeet brings a unique perspective to the evolving landscape of hybrid work environments.
His expertise in strategic IT leadership, audiovisual engineering, and virtual event production positions him as a key voice in shaping collaborative and productive workspaces. Keen to learn and stay abreast of the latest developments in IT and audiovisual technologies, Harmeet offers valuable insights into cutting-edge solutions for creating engaging, flexible, and purpose-built workplaces that meet the demands of today’s dynamic business world.
Transparency, transparency, and more transparency is the way to ensure that data privacy regulations are being upheld. If all collection, security, and predictive analytics are transparent, compliance is guaranteed.
In addition, users must have control over their data, give informed consent, and minimal data must be collected. Strictly the data that serves the stated purpose.
To prevent bias in predictive models, we have to start with diverse datasets. In addition, we have to monitor biases in datasets, and correct them as necessary. Cross validation and human oversight are key to identifying bias and correction.
Data mapping is our foundation. We conduct detailed audits on a regular basis to trace the lifecycle of user data. This includes identifying how we gather data via surveys, analyze user interactions on our platform, and capture dietary habits.
Once we understand this data journey, we can see where our responsibilities under regulation such as GDPR or CCPA are. For instance, this mapping exercise prompted us to add two-factor authentication to user accounts, offering another layer of protection for valuable dietary data.
And more importantly, we believe in “Data Protection by Design and Default”. Privacy is built into the core of our platform. All user data is encrypted both while in transit and at rest to avoid potential exposure. And we collect as little data as we need to be able to deliver our services.
A symptom-tracking app, for example, may want information on stomach problems, but we wouldn’t ask for something trivial such as a user’s address. This method keeps the volume of personal data we hold at a minimum and eliminates misuse.
The process of gaining user trust is never done. We also regularly audit our predictive models. This entails scrutinizing the algorithms behind things such as personalized meal plan recommendations to ensure they’re not biased.
1. Prioritizing Data Privacy and Regulatory Compliance
Startups need to be proactive about data privacy, especially with regulations like GDPR and CCPA in place. Here’s how businesses can ensure they’re compliant:
Data Anonymization: Always anonymize personal data wherever possible. This means stripping out any personally identifiable information so that data can’t be traced back to individuals, which helps mitigate privacy concerns.
Transparent Consent Processes: Make sure users fully understand how their data will be used and that they’ve consented to it. Using user-friendly consent forms and allowing users to opt-out at any time is key.
Ongoing Privacy Audits: Regularly audit your data practices to identify potential vulnerabilities. This ensures you’re staying compliant with evolving privacy laws and industry standards.
2. Addressing Bias in AI Models
Predictive models can inadvertently reflect biases in the training data, leading to skewed outcomes. To avoid this:
Diverse Data Sets: Train models on diverse data to minimize the risk of biased predictions. This means including data from different demographics to ensure fairer outcomes.
Bias Monitoring: Use tools designed to detect bias in AI models. Regularly review the model’s performance, especially when bias could have serious consequences, such as lending, hiring, or healthcare.
Human Oversight: AI shouldn’t operate in a vacuum. Having humans review predictions, especially in critical areas, can help catch potential biases or errors early on.
3. Developing Ethical Guidelines
Establishing a solid framework of ethical guidelines can help align your AI initiatives with your company’s values:
Form an Ethics Committee: Have a dedicated group within the company to review AI projects and ensure they align with ethical standards. This committee can oversee projects and recommend adjustments where necessary.
Ethics Training for Staff: Educate your team on responsible AI practices. This includes understanding data privacy, bias mitigation, and the ethical implications of using AI.
Accountability and Documentation: Keep clear records of how your predictive models are trained, tested, and deployed. Documenting this process helps ensure transparency and traceability in AI-driven decisions.
4. Conducting Regular Ethical Audits
Periodic audits are essential to ensure your AI tools are working as intended and are not unintentionally causing harm:
Model Accuracy Checks: Continuously test models to ensure their predictions are accurate and unbiased. This includes simulating real-world scenarios to catch potential issues before fully deploying models.
Data Security Reviews: Regularly check your data storage and protection protocols to guard against breaches. This is particularly important if sensitive user information is involved.
Ethical Impact Assessments: Conduct assessments to understand the broader impact of your AI systems on society. This helps identify and address any unintended negative consequences early on.
AI and predictive analytics have incredible potential, but using them responsibly is crucial. By incorporating these best practices, startups can leverage AI to drive value while protecting user trust and complying with regulations.
Conclusion
AI-driven predictive analytics is transforming the way startups operate, offering unparalleled opportunities to gain insights, optimize operations, and drive growth. For startup founders, understanding and leveraging this technology is no longer optional but essential for staying competitive and achieving long-term success.
Getting started with AI-driven predictive analytics involves several critical steps: establishing clear objectives, investing in data quality and management, starting with pilot projects, building a cross-functional team, leveraging the right tools, and integrating analytics into core business processes.
Additionally, continuous monitoring, fostering a culture of improvement, securing executive buy-in, and prioritizing ethical considerations are key to maximizing the value of predictive analytics.
Read Next:
- Fun and Engaging Marketing Ideas for April Fool’s Day
- How Real Estate Agents Can Market Themselves
- Festive Campaign Ideas for Christmas Marketing
- Marketing Strategies for Architecture Firms
- Catchy Name Ideas for Your Digital Marketing Company
Comments are closed.