Hello there! Are you curious about how artificial intelligence (AI) is changing our understanding of the environment? You’re in the right place. AI-driven analytics is revolutionizing how we predict and respond to environmental changes, from weather forecasting to disaster management and sustainability efforts. In this article, we’ll explore how AI can make accurate environmental predictions, help us tackle climate change, and protect our planet for future generations. Ready to dive in? Let’s get started!
The Power of AI in Environmental Predictions
AI’s Role in Transforming Environmental Predictions
AI-driven analytics is not just a tool; it’s a transformative force reshaping how we understand and interact with our environment. For startup founders, leveraging AI in environmental predictions offers an unprecedented opportunity to drive innovation, create sustainable solutions, and address some of the most pressing challenges of our time.
AI enables us to process and analyze vast amounts of data with speed and accuracy that far surpasses traditional methods. This capability is crucial in environmental science, where data from satellites, sensors, and historical records must be synthesized to predict future conditions. By automating data analysis, AI frees up human experts to focus on interpreting results and making strategic decisions.
Strategic Advantages of AI in Environmental Predictions
For startups, the strategic advantages of incorporating AI into environmental predictions are significant. AI can provide more accurate and timely forecasts, enabling businesses to anticipate and respond to environmental changes proactively. This predictive power can help startups in sectors like agriculture, energy, and urban planning to optimize their operations and reduce environmental impact.
Consider a startup in the renewable energy sector. AI-driven weather predictions can optimize the operation of wind turbines and solar panels by forecasting wind speeds and sunlight intensity. This ensures that energy production is maximized while maintenance costs are minimized. Similarly, AI can predict energy demand based on weather patterns and consumption data, allowing startups to manage resources more efficiently and reduce waste.
Enhancing Accuracy with AI
One of the key benefits of AI in environmental predictions is its ability to enhance accuracy. Traditional prediction models often struggle with the complexity and variability of environmental data. AI, however, excels at identifying patterns and correlations within large datasets, making it possible to develop more precise models.
For instance, AI can improve the accuracy of climate models by integrating data from multiple sources, such as ocean temperatures, atmospheric CO2 levels, and land use changes. These enhanced models can predict climate phenomena like El Niño and La Niña events with greater reliability, helping businesses and governments prepare for their impacts.
In agriculture, accurate weather predictions can guide planting schedules, irrigation practices, and pest control measures. AI can analyze soil moisture levels, temperature forecasts, and crop health data to recommend optimal farming practices, boosting yields and reducing resource use. For agritech startups, these capabilities can provide a competitive edge and attract investment.
Real-Time Data Processing and Decision Making
AI’s ability to process data in real-time is a game-changer for environmental predictions. Traditional models often require time-consuming data collection and analysis, delaying the availability of critical insights. AI, however, can analyze incoming data streams continuously, providing real-time updates and predictions.
For example, AI can enhance flood prediction systems by analyzing real-time data from river sensors, rainfall measurements, and weather forecasts. This enables authorities to issue timely flood warnings and coordinate emergency responses more effectively. Startups developing flood management solutions can leverage these capabilities to protect communities and infrastructure.
In urban planning, real-time air quality monitoring can inform decisions on traffic management, industrial activity, and public health measures. AI can analyze data from air quality sensors, weather stations, and traffic cameras to predict pollution levels and recommend actions to mitigate exposure. For startups focused on smart city solutions, these insights can improve urban livability and sustainability.
Scalability and Adaptability of AI Solutions
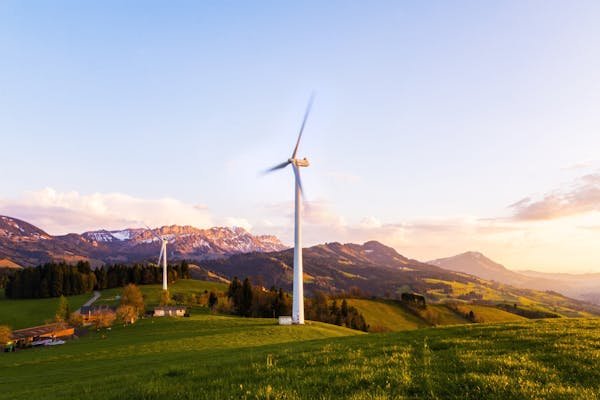
AI-driven analytics are highly scalable and adaptable, making them suitable for startups of all sizes and stages. Whether you are a small startup with limited resources or a growing company with complex needs, AI solutions can be tailored to fit your requirements.
Cloud-based AI platforms offer scalable infrastructure that grows with your business. You can start with basic predictive models and gradually incorporate more advanced capabilities as your data and needs evolve. This flexibility allows startups to invest in AI incrementally, aligning costs with benefits.
Moreover, AI solutions are adaptable to different environmental contexts and challenges. A startup working on water resource management can use AI to predict water availability based on local climate data, while another focusing on wildlife conservation can use AI to monitor animal populations and habitats. This adaptability ensures that AI can address a wide range of environmental issues, providing value across various sectors.
Driving Innovation with AI
For startup founders, AI-driven environmental predictions can be a catalyst for innovation. By leveraging AI, startups can develop new products, services, and business models that address environmental challenges in unique ways. This not only contributes to sustainability but also opens up new market opportunities.
Consider a startup developing AI-powered sensors for environmental monitoring. These sensors can be deployed in forests to detect early signs of wildfires, in oceans to monitor coral reef health, or in cities to track pollution levels. By providing real-time data and predictive insights, these solutions can help prevent environmental damage and enhance conservation efforts.
AI can also drive innovation in policy and advocacy. Startups working in environmental policy can use AI to analyze the effectiveness of regulations and recommend improvements. By providing data-driven insights, AI can support the development of policies that balance economic growth with environmental protection.
Overcoming Challenges in AI Implementation
While the benefits of AI in environmental predictions are clear, implementing AI solutions comes with its own set of challenges. Data quality and availability, algorithm transparency, and integration with existing systems are some of the key hurdles that startups must overcome.
Ensuring high-quality data is critical for accurate predictions. Startups should invest in robust data collection and validation processes to minimize errors and biases. Partnering with research institutions, government agencies, and other stakeholders can help access reliable data sources.
Algorithm transparency is another important consideration. AI models should be explainable, meaning that their decision-making processes can be understood and trusted by users. Startups should prioritize transparency and accountability in their AI development, ensuring that predictions are not only accurate but also credible.
Integrating AI solutions with existing systems requires careful planning and coordination. Startups should work closely with IT teams to ensure seamless integration and interoperability. Investing in training and capacity-building for staff can also facilitate the adoption of AI technologies.
The Future of AI in Environmental Predictions
Looking ahead, the role of AI in environmental predictions is set to expand even further. Advances in machine learning, big data, and IoT will continue to enhance the accuracy and scope of AI-driven analytics. For startup founders, staying abreast of these developments and continuously innovating will be key to leveraging AI for environmental sustainability.
Future AI models will likely incorporate even more diverse data sources, including social media, citizen science, and mobile applications. These data streams can provide valuable insights into environmental conditions and human behavior, enriching predictive models and improving decision-making.
Collaboration will be crucial for scaling AI solutions. Startups should seek partnerships with academia, industry, and government to share knowledge, resources, and expertise. Collaborative platforms and open data initiatives can foster innovation and accelerate the development of AI-driven environmental solutions.
Key Applications of AI in Environmental Predictions
Weather Forecasting
Weather forecasting is one of the most well-known applications of AI in environmental science. Traditional weather models rely on numerical simulations that can be time-consuming and resource-intensive. AI-driven models, on the other hand, can process data from weather stations, satellites, and sensors in real-time, providing more accurate and timely forecasts.
For example, AI can predict severe weather events such as hurricanes and tornadoes with greater precision. By analyzing patterns in atmospheric data, AI can identify the conditions that lead to these events and provide early warnings. This allows communities to prepare and respond more effectively, reducing the impact of such disasters.
Climate Change Modeling
Understanding and addressing climate change is one of the most pressing challenges of our time. AI-driven analytics can play a crucial role in climate change modeling by analyzing vast amounts of data from various sources, such as temperature records, greenhouse gas emissions, and land use changes.
AI can help scientists develop more accurate climate models that predict future climate scenarios based on different variables. For example, AI can simulate the impact of different levels of carbon emissions on global temperatures, sea levels, and weather patterns. These predictions can inform policy decisions and guide efforts to mitigate climate change.
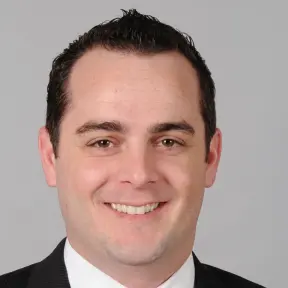
In my experience as a legal innovator with LawHustle, leveraging AI for strategic growth without increasing expenditure has been transformative.
Similarly, AI-driven climate models can efficiently improve policy development by precisely targeting areas that require intervention, just as we’ve streamlined client acquisition without boosting traditional marketing spend.
One real-world application from my work is how AI has improved lead qualification efficiency.
This parallels the potential for AI in climate modeling to accurately predict high-risk areas for natural disasters, helping governments allocate resources more effectively.
By focusing on specific predictive insights, policies can become more targeted and efficient.
I’ve seen that technology can transform traditional markets, as demonstrated by LawHustle’s success in converting leads automagically.
The same can apply to climate policies where AI-driven models enable rapid responses without overwhelming existing resources—guiding more informed decisions for carbon reduction or adaptation.
Disaster Management
AI-driven analytics can significantly improve disaster management by providing accurate predictions and real-time information. For example, AI can analyze data from seismic sensors to predict earthquakes and aftershocks. It can also monitor river levels and weather conditions to predict floods and landslides.
During a disaster, AI can help coordinate emergency response efforts by analyzing data from various sources, such as social media, satellite images, and sensor networks. This information can be used to identify affected areas, allocate resources, and provide timely updates to the public.
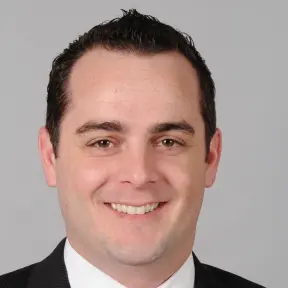
As a seasoned attorney and innovator, I’m in a unique position to discuss AI’s benefits, having developed LawHustle, a tool that uses AI to streamline client acquisition for law firms.
LawHustle’s AI component illustrates how AI can analyze data and automate processes efficiently, similar to disaster management scenarios where AI analyzes real-time data from sensors to predict events like floods or wildfires.
In developing LawHustle, AI is used to engage and qualify leads without manual intervention, dramatically reducing response times—akin to AI systems automating emergency communication in disaster management, speeding up response efforts.
LawHustle’s success demonstrates AI’s capacity to automate and improve decision-making processes, providing a concrete example of how AI can be leveraged effectively in various sectors, including disaster management.
Through LawHustle, I’ve seen how AI can handle vast datasets to predict outcomes and streamline processes, suggesting a similar approach could be applied to disaster prediction.
By anticipating potential disruptions earlier, stakeholders can allocate resources more effectively, just as law firms can allocate resources to the most promising clients through AI-driven insights.
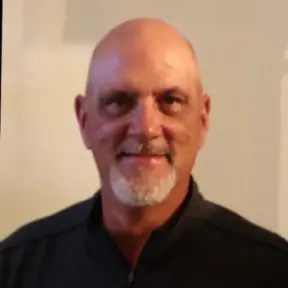
In my role as President at eSportsInsurance, I’ve experienced how critical data analysis is in predicting and managing risks within the sports sector.
We provide coverage for numerous sports activities, which requires assessing risk factors and implementing appropriate preemptive measures.
Drawing parallels from this, AI’s ability to process real-time data from sensors can significantly improve risk management in disaster scenarios.
For example, our handling of COVID-19 insurance adjustments showcased the power of real-time data analysis in decision-making.
Just as AI would interpret data from sensors to provide early disaster warnings, we relied on live data to adjust coverage and advise organizations on safety protocols.
This proactive approach can improve response times and risk assessments, mirroring the potential benefits AI brings to disaster management.
Additionally, eSportsInsurance’s ability to tailor insurance coverage to specific needs showcases how customizing AI solutions can aid in disaster prediction.
Analyzing unique datasets allows us to offer precise risk mitigation strategies, similar to how AI can be custom to improve disaster readiness through thorough data interpretation.
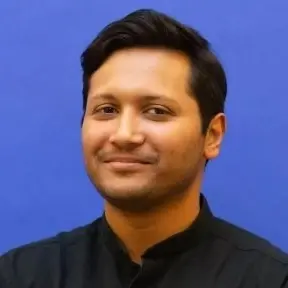
AI has been a huge part of many industries, and one area where it’s made a significant impact is inventory management.
AI-driven predictive analytics isn’t just improving how businesses manage their stock; it’s transforming the entire process to become more efficient and responsive to real-time data.
One great example of this is how AI can help balance inventory levels. Traditional inventory management usually involved looking at historical sales data and making predictions based on past trends, but AI takes it much further.
AI can analyze data in real time, pulling in not just sales data but also consumer behavior patterns, seasonal demand, and even external factors like weather or market shifts. This allows businesses to maintain the right stock levels more accurately.
Take Walmart, for example. They use AI-driven analytics to predict consumer buying patterns and adjust their inventory accordingly.
Their system analyzes countless factors like weather data, local events, and search trends to anticipate which products will be in demand and which won’t.
This has helped them reduce overstocking by more than 10%, freeing up warehouse space and cutting costs associated with holding excess inventory.
It also allows them to better meet customer demand, ensuring popular items are always available when needed.
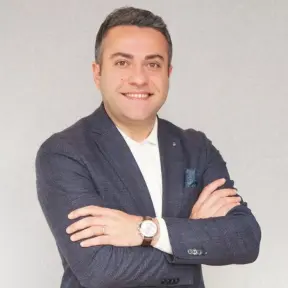
I’ve had hands-on experience with AI applications in data-driven decision-making. I’d love to share how I’ve seen AI play a crucial role in disaster management and prediction.
I’ve always been fascinated by how AI can process vast amounts of data to make sense of chaotic situations, especially when it comes to natural disasters.
One of the most impressive examples I’ve encountered is how AI was used during floods in Southeast Asia. I saw firsthand how AI systems, analyzing satellite data and real-time sensor input, predicted flood patterns well in advance.
I think the ability to provide early warnings gave local authorities extra time to evacuate and prepare resources, which saved lives.
I also believe AI’s capacity to analyze data from multiple sources (like drones, satellites, and sensors) offers a game-changing advantage in disaster management.
During wildfire season in California, AI algorithms helped firefighters anticipate wind patterns and spot high-risk zones faster than traditional methods.
I’ve seen how AI reduces response times and improves resource allocation, making it an invaluable tool.
I’d be happy to share my insights on how AI-driven analytics is enhancing real-time disaster prediction and improving response strategies. As a specialist in content marketing agency who closely works with innovative AI and disaster management teams, I’ve seen how these technologies can significantly improve disaster preparedness and recovery efforts.
AI analytics helps process massive volumes of real-time data from sources like sensors, satellites, weather forecasts, and social media to provide early warning signs and predict disasters more accurately. For instance, AI is used to predict floods by analyzing real-time water levels, rainfall patterns, and geographical data. Machine learning models can track weather changes and generate forecasts with a higher degree of accuracy, allowing local authorities to issue timely warnings and deploy resources effectively.
In the case of wildfires, AI-driven systems can analyze satellite imagery and sensor data to detect changes in temperature, humidity, and wind speed that indicate potential fire outbreaks. AI can identify patterns in historical wildfire data to predict high-risk areas and allow agencies to prioritize their response and allocate resources like fire-fighting crews and equipment.
An example of AI in action is Google’s AI-powered flood forecasting system, which provides real-time flood alerts in India, allowing communities to evacuate and emergency responders to act faster. Similarly, NASA’s Earth Observing System Data and Information System (EOSDIS) uses AI to analyze satellite imagery, providing crucial data to predict natural events like earthquakes, hurricanes, and wildfires.
AI is transforming disaster management by providing real-time, data-driven insights that support faster decision-making, resource allocation, and, ultimately, saving lives.
Air Quality Monitoring
Air quality is a critical aspect of environmental health that affects millions of people worldwide. AI-driven analytics can enhance air quality monitoring by analyzing data from air quality sensors, weather stations, and traffic patterns.
AI can predict air pollution levels based on factors such as weather conditions, traffic volume, and industrial emissions. This information can be used to issue health warnings, implement traffic control measures, and develop policies to reduce air pollution. Additionally, AI can identify pollution hotspots and monitor the effectiveness of air quality improvement initiatives.
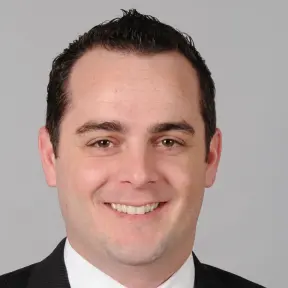
In my work developing LawHustle, an AI-powered tool for law firms, I have seen how AI can transform industries. AI models use data-driven insights to optimize operations, much like how it can be applied to predict and monitor air quality.
For example, LawHustle uses AI to streamline lead conversion by predicting which leads are most likely to convert, akin to how AI can predict pollution spikes by analyzing environmental data.
One way AI is applicable to air quality monitoring, much like in legal technology, is through process automation and data unification.
At LawHustle, we merge multiple communication channels into one unified system to streamline client interactions.
Similarly, AI in air quality can consolidate diverse data sources—like weather patterns, industrial emissions, and traffic data—to provide a comprehensive prediction model.
Another component is the personalized interaction with data. In LawHustle, AI personalizes client interactions to improve engagement.
In air quality monitoring, AI can tailor pollutant alerts and public health recommendations based on localized data, providing citizens with relevant, actionable information.
Such AI-driven strategies are crucial for enhancing both legal operations and environmental management efficiently.
Water Resource Management
Water is a vital resource that requires careful management to ensure its availability for future generations. AI-driven analytics can help manage water resources more effectively by analyzing data from water sensors, weather stations, and satellite images.
For example, AI can predict water demand based on factors such as population growth, weather conditions, and agricultural needs. It can also monitor water quality by analyzing data from sensors that detect pollutants and contaminants. This information can guide efforts to conserve water, improve water quality, and develop sustainable water management practices.
Key Technologies and Tools in AI-Driven Environmental Predictions
Machine Learning Algorithms
Machine learning (ML) algorithms are at the heart of AI-driven analytics. They can process and analyze large datasets to identify patterns, trends, and correlations that are not immediately obvious to human analysts.
For environmental predictions, supervised learning algorithms like decision trees, support vector machines, and neural networks are commonly used. These algorithms learn from labeled training data and can make accurate predictions based on new, unseen data.
Unsupervised learning algorithms, such as clustering and association, are also valuable for exploring environmental data. They can identify natural groupings and relationships within data, which can be crucial for understanding complex environmental systems.
Reinforcement learning, another subset of machine learning, can optimize resource management strategies by learning the best actions to take in dynamic environments through trial and error.
Big Data Platforms
Handling the massive volumes of data required for environmental predictions necessitates robust big data platforms. Technologies like Apache Hadoop and Apache Spark allow for the distributed processing of large datasets across multiple servers. These platforms enable the efficient storage, retrieval, and analysis of environmental data from various sources, such as weather stations, satellites, and IoT devices.
Cloud-based solutions, such as Amazon Web Services (AWS), Google Cloud Platform (GCP), and Microsoft Azure, provide scalable infrastructure for managing and analyzing big data. These platforms offer tools and services specifically designed for data analytics and machine learning, making it easier for organizations to deploy AI-driven environmental prediction models.
Geographic Information Systems (GIS)
Geographic Information Systems (GIS) are essential tools for visualizing and analyzing spatial data. GIS technology integrates various data layers, such as topography, land use, and climate data, to provide a comprehensive view of environmental conditions. AI algorithms can be integrated with GIS to enhance spatial analysis and prediction capabilities.
For instance, GIS can be used to map areas at risk of flooding by analyzing elevation data, rainfall patterns, and river levels. AI can then predict the likelihood of flooding in specific regions, allowing authorities to take preventive measures. GIS technology also plays a crucial role in monitoring deforestation, urban sprawl, and habitat loss by providing detailed visualizations of land cover changes over time.
Remote Sensing
Remote sensing technologies, such as satellites and drones, provide critical data for environmental monitoring and predictions. Satellites equipped with sensors can capture images and data on various environmental parameters, including vegetation cover, soil moisture, and sea surface temperatures. Drones offer high-resolution imagery and can access remote or difficult-to-reach areas, providing valuable data for environmental analysis.
AI-driven analytics can process and interpret remote sensing data to detect changes and trends in the environment. For example, AI can analyze satellite images to monitor glacier retreat, deforestation rates, and the spread of wildfires. By integrating remote sensing data with machine learning models, scientists can make more accurate predictions about environmental changes and their impacts.
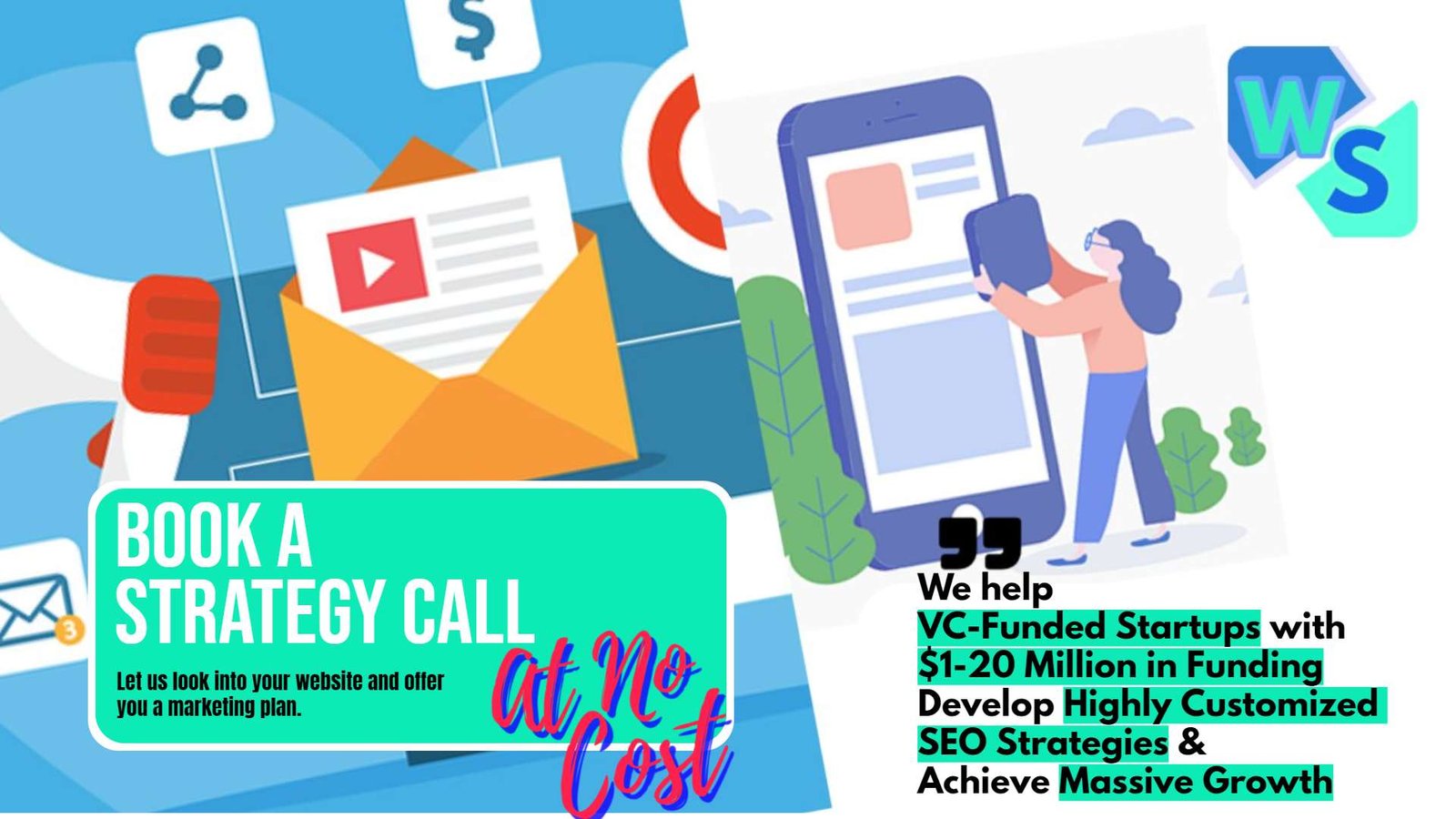
Related: Check out our free tools:
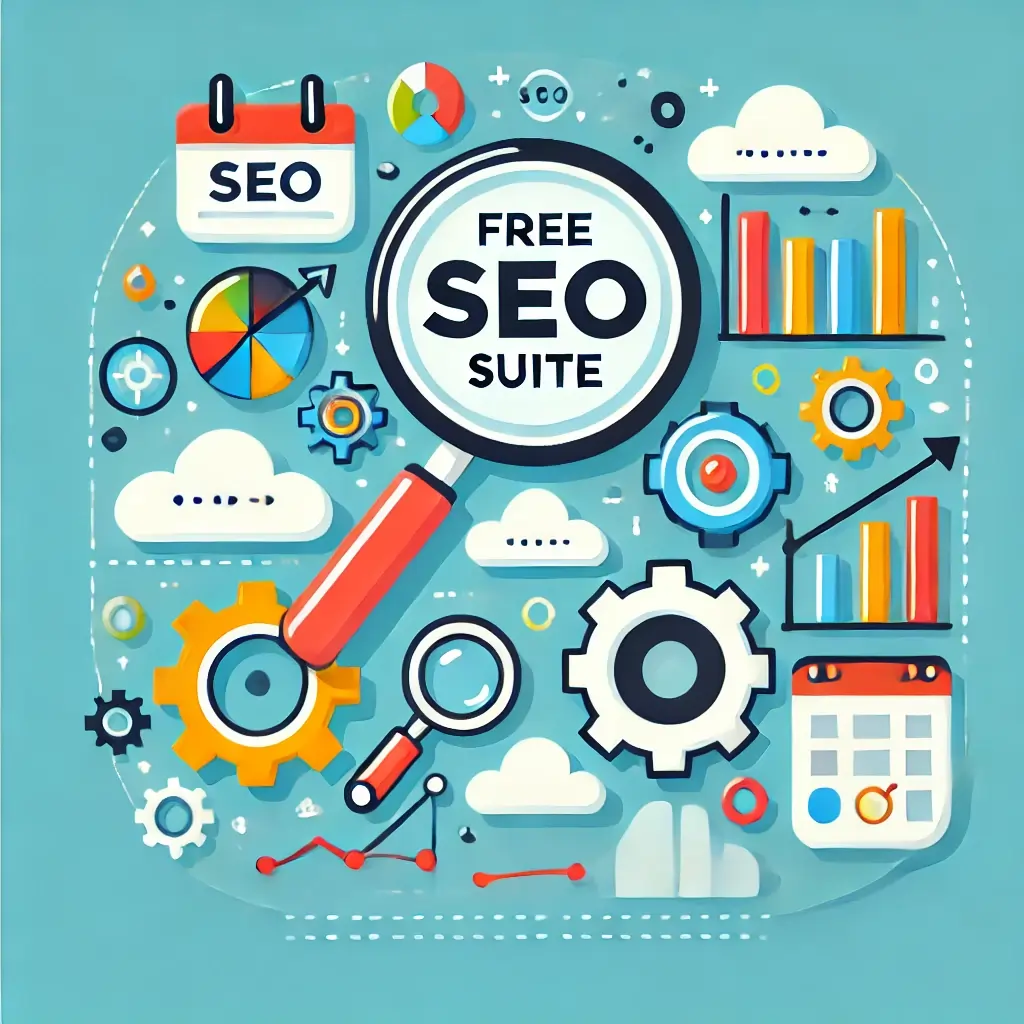
Real-World Applications of AI in Environmental Predictions
Predicting and Mitigating Climate Change
Climate change poses one of the most significant threats to our planet, and AI-driven analytics is playing a crucial role in addressing this challenge. By analyzing vast amounts of climate data, AI can identify the key drivers of climate change and predict future trends under different scenarios. These predictions are essential for developing effective mitigation strategies and policies.
For example, AI models can simulate the impact of various greenhouse gas emission reduction scenarios on global temperatures. Policymakers can use these simulations to set realistic emission targets and develop action plans to achieve them.
AI can also predict the effects of climate change on specific regions, such as rising sea levels, increased frequency of extreme weather events, and changes in agricultural productivity. This information can guide adaptation efforts to protect vulnerable communities and ecosystems.
Enhancing Biodiversity Conservation
Biodiversity is crucial for maintaining healthy ecosystems, and AI-driven analytics can support conservation efforts by providing detailed insights into species distributions and habitat conditions. Machine learning models can analyze data from camera traps, acoustic sensors, and satellite imagery to monitor wildlife populations and their movements.
For instance, AI can help track endangered species by identifying them in images captured by camera traps and drones. This enables conservationists to monitor population trends and identify critical habitats that need protection.
AI can also analyze environmental data to predict how changes in land use, climate, and human activities will affect biodiversity. These predictions can inform conservation planning and help prioritize areas for protection and restoration.
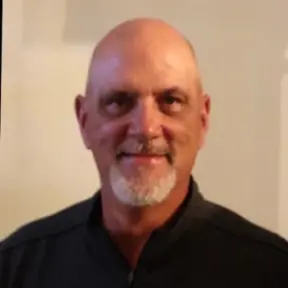
As the President of eSportsInsurance, I’ve seen how technology, particularly AI, transforms industries.
While our primary focus is sports insurance, the parallels with biodiversity conservation are striking.
In terms of wildlife monitoring, AI devices like camera traps or drones operate similarly to how we assess risks in sports environments—by gathering extensive data efficiently.
One example that’s particularly relevant is the use of AI in analyzing vast amounts of visual data to detect movement patterns. In conservation, this technology helps monitor animal migrations and population sizes, akin to how we evaluate sports event risk factors using predictive analytics.
Such AI applications create a robust understanding of ecosystem changes just like we manage insurance claims through detailed data assessments.
Furthermore, the use of AI-driven acoustic sensors in conservation—tracking animal calls to assess species presence and behavior—mirrors our use of real-time data in event management.
These AI tools don’t just automate data collection; they improve precision and response time, crucial for protecting endangered species much like they are for safeguarding sports events.
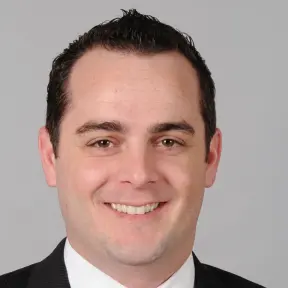
Using AI in biodiversity conservation reminds me of how I’ve used AI to transform client acquisition in law firms without hefty marketing spends.
At LawHustle, we implemented AI-driven systems to collect and analyze client data swiftly, similar to how AI manages extensive wildlife data for species tracking.
AI tools in our platform automate lead qualification, reducing manual effort and improving engagement rates, akin to AI’s role in enhancing the accuracy and efficiency of monitoring wildlife populations.
One specific parallel is with AI’s predictive capabilities. In law firms, the prediction of client behaviors from lead data streamlines follow-ups and optimizes conversion strategies.
In conservation, predictive analytics from AI could forecast species’ migration patterns and habitat changes, enabling preemptive actions to protect at-risk habitats.
This approach allows both industries to act proactively rather than reactively, paving the way for sustainable growth and conservation efforts.
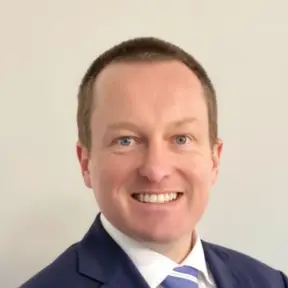
An aspect of AI in conservation that doesn’t get talked about enough is its ability to analyze audio data. Researchers are using AI to listen to sounds in natural habitats, which can help identify species based on their calls and vocalizations.
This method has been very effective for monitoring bird populations in dense forests where visibility is limited.
Instead of having to visually spot every single bird, AI can process hours of audio recordings and pinpoint the presence of specific species.
This capability saves time and resources and enables conservationists to gather data on species that may be elusive or endangered.
With the insights gained, conservationists can implement targeted actions to protect these species and their habitats, which might otherwise go unnoticed.
Monitoring and Managing Water Resources
Water scarcity and pollution are major environmental challenges that affect millions of people worldwide. AI-driven analytics can enhance water resource management by providing accurate predictions and real-time monitoring capabilities. Machine learning models can analyze data from sensors, weather stations, and satellite imagery to predict water demand, availability, and quality.
For example, AI can predict seasonal variations in water demand for agriculture, allowing farmers to plan irrigation schedules more effectively. It can also monitor water quality by analyzing data on pollutant levels and detecting anomalies that indicate contamination. This information can guide efforts to improve water treatment and distribution systems, ensuring a reliable supply of clean water.
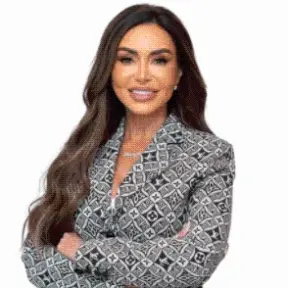
I’ve dedicated my career to understanding injury and safety law, so while I may not be a direct expert in AI for water management, I can offer insights from my experience dealing with technologies that impact safety and legal frameworks, which have parallels in regulatory compliance for AI in water systems.
At Avrek Law Firm, we handle complex evidence often involving technology, like Event Data Recorders (EDRs) in accident cases, which provide rich, analytic data crucial for accurate liability assessments.
AI-driven analytics in water management can similarly harness massive datasets to improve efficiency and reliability.
For instance, just as EDR data provides clarity in accident cases, AI can predict water demand with precision, leading to more effective resource allocation, akin to ensuring fair settlements in injury cases.
Precision in prediction and monitoring can reduce conflicts over resource use, much like the clarity we seek in personal injury claims through detailed evidence analysis.
I see AI in water management akin to using forensic reconstruction in legal cases—both require careful application of technology to assess scenarios accurately and to provide strategic solutions that prevent future issues.
The effectiveness depends on the quality of data collection and the ethical application of insights, a theme central in both legal practice and sustainable water management.
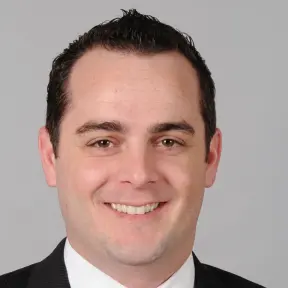
In my journey creating LawHustle, I’ve learned how powerful AI can be in changing traditional practices.
When it comes to AI in water resource management, parallels can be drawn from how we’ve custom AI-driven tools for law firms to predict client demand and optimize engagement.
Similar principles can be applied to AI predicting water demand in urban and agricultural settings.
A real-world example from LawHustle involves using AI to automate client interactions, allowing firms to focus resources effectively.
Just as AI can manage and convert leads efficiently, it can similarly monitor water quality and automate adjustments to distribution, supporting sustainability efforts without excessive manual oversight.
In leveraging AI to streamline operations within legal practices, I’ve seen the possibilities of data-driven automation.
This approach can be translated to water management, where AI monitors and responds to real-time data trends, akin to our methods of improving client acquisition processes.
By adopting such a model, water systems could improve their conservation and distribution strategies efficiently.
Improving Air Quality Forecasting
Air pollution is a significant public health concern, and AI-driven analytics can improve air quality forecasting by analyzing data from sensors, weather stations, and traffic patterns. Machine learning models can predict pollution levels based on factors such as weather conditions, traffic volume, and industrial emissions.
For instance, AI can predict periods of high pollution and issue health warnings to vulnerable populations. It can also identify pollution hotspots and monitor the effectiveness of air quality improvement initiatives. By providing accurate and timely air quality forecasts, AI can help reduce exposure to harmful pollutants and protect public health.
Practical Steps to Implement AI-Driven Environmental Predictions
Defining Your Objectives
Before diving into AI-driven analytics, it’s essential to define clear objectives. Understand what you want to achieve with AI in environmental predictions. Are you focusing on improving weather forecasts, enhancing disaster preparedness, monitoring biodiversity, or optimizing resource management? Clearly defined objectives will guide your strategy and help you measure success.
Start by identifying the specific environmental issues you aim to address. For instance, if you want to improve air quality in urban areas, your objective might be to reduce pollution levels by predicting high-risk periods and implementing mitigation measures. Setting SMART (Specific, Measurable, Achievable, Relevant, Time-bound) goals will provide a clear roadmap for your AI initiatives.
Gathering and Preparing Data
Data is the backbone of AI-driven analytics. To make accurate environmental predictions, you need high-quality, comprehensive data from reliable sources. This can include satellite imagery, sensor data, weather station records, and historical environmental data. Collaborate with governmental agencies, research institutions, and private companies to access relevant datasets.
Once you have the data, the next step is data preprocessing. This involves cleaning, normalizing, and transforming the data to make it suitable for analysis. Remove any duplicates, fill in missing values, and convert data into a consistent format. Data preprocessing is crucial for ensuring the accuracy and reliability of your AI models.
Choosing the Right AI Tools and Technologies
Selecting the right AI tools and technologies is critical for the success of your environmental predictions. There are various AI platforms and frameworks available, each with its own strengths and capabilities. Some popular options include TensorFlow, PyTorch, and Scikit-learn for machine learning, and ArcGIS for spatial analysis.
Consider the specific requirements of your project when choosing AI tools. For instance, if you need to process large volumes of satellite imagery, look for tools that offer robust image processing capabilities.
If your focus is on real-time data analysis, choose platforms that support real-time data streaming and processing. Cloud-based solutions like AWS, Google Cloud, and Microsoft Azure provide scalable infrastructure and specialized services for AI and big data analytics.
Building and Training AI Models
With your data ready and tools selected, the next step is to build and train your AI models. Start by selecting the appropriate machine learning algorithms based on your objectives and data characteristics. For example, use convolutional neural networks (CNNs) for image analysis, recurrent neural networks (RNNs) for time series forecasting, and decision trees for classification tasks.
Divide your data into training, validation, and test sets. Use the training set to train your models, the validation set to tune hyperparameters, and the test set to evaluate model performance. Monitor key metrics such as accuracy, precision, recall, and F1 score to assess the effectiveness of your models. Iterate on your models by adjusting parameters, experimenting with different algorithms, and incorporating new data to improve performance.
Integrating AI Models into Operational Systems
Once your AI models are trained and validated, integrate them into your operational systems to generate real-time predictions and insights. This involves deploying your models on a production environment and setting up data pipelines to feed live data into the models. Ensure that your infrastructure can handle the computational demands of running AI models in real-time.
Develop user-friendly dashboards and interfaces that present the predictions and insights generated by your AI models. These interfaces should provide actionable information that stakeholders can use to make informed decisions. For example, a dashboard for air quality forecasting might display predicted pollution levels, health advisories, and recommended mitigation measures.
Monitoring and Refining AI Models
Implementing AI-driven environmental predictions is an ongoing process that requires continuous monitoring and refinement. Regularly evaluate the performance of your AI models by comparing predictions with actual outcomes. Identify any discrepancies and investigate their causes to improve model accuracy.
Stay updated with the latest advancements in AI and environmental science. As new algorithms, tools, and techniques emerge, incorporate them into your models to enhance their capabilities. Encourage a culture of continuous learning and improvement within your team, and collaborate with experts in AI and environmental science to stay at the forefront of innovation.
Ensuring Ethical and Responsible AI Use
Ethical considerations are paramount when implementing AI-driven analytics for environmental predictions. Ensure that your AI models are transparent, fair, and unbiased. Address any potential biases in your data and algorithms that could lead to inaccurate or discriminatory predictions.
Prioritize data privacy and security by implementing robust data protection measures. Comply with relevant regulations and standards, such as GDPR and CCPA, to safeguard the privacy of individuals whose data you are analyzing. Promote transparency by clearly communicating how your AI models work and how predictions are generated.
Engage with stakeholders, including communities affected by environmental changes, policymakers, and environmental organizations, to ensure that your AI initiatives are aligned with broader societal goals. Consider the ethical implications of your predictions and use them to promote sustainability and environmental justice.
Case Studies: Success Stories of AI-Driven Environmental Predictions
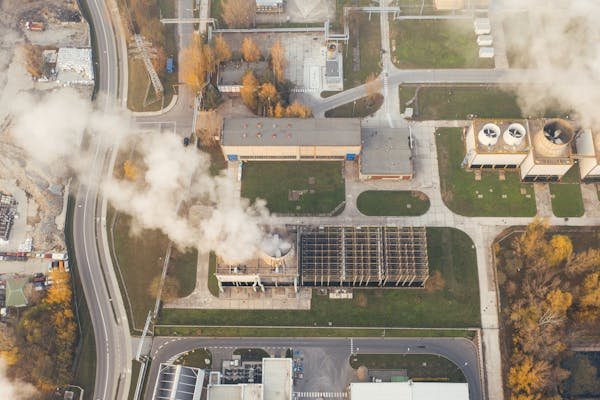
IBM’s Green Horizon Project
IBM’s Green Horizon Project is a prime example of how AI-driven analytics can transform environmental management. Launched in China, the project aimed to tackle air pollution by predicting pollution levels and identifying sources of emissions.
Using AI algorithms to analyze data from air quality monitors, weather stations, and industrial emissions, the project provided real-time pollution forecasts and actionable insights for policymakers.
As a result, authorities were able to implement targeted measures to reduce emissions, such as shutting down polluting factories during high-risk periods and adjusting traffic patterns. The project significantly improved air quality in major cities and demonstrated the potential of AI to address complex environmental challenges.
Google’s Environmental Insights Explorer
Google’s Environmental Insights Explorer (EIE) leverages AI-driven analytics to help cities measure and reduce their carbon emissions. EIE analyzes data from Google Maps, satellite imagery, and other sources to estimate transportation emissions, building energy use, and rooftop solar potential. The tool provides cities with detailed insights into their environmental impact and identifies opportunities for reducing emissions.
Cities can use EIE to develop data-driven sustainability plans, monitor progress towards emission reduction goals, and engage with residents on climate action. The tool has been adopted by numerous cities worldwide, showcasing how AI can support urban sustainability efforts.
The Nature Conservancy’s AI for Conservation
The Nature Conservancy (TNC) has harnessed the power of AI to support biodiversity conservation. TNC uses machine learning models to analyze data from camera traps, drones, and acoustic sensors to monitor wildlife populations and habitat conditions. AI algorithms identify species in images and recordings, track their movements, and assess habitat quality.
This information enables conservationists to prioritize areas for protection, monitor the effectiveness of conservation initiatives, and adapt strategies based on real-time data. TNC’s AI-driven approach has enhanced their ability to protect endangered species and preserve biodiversity.
Conclusion
Read Next:
- AI-Powered Marketing Analytics: Boost Your Campaigns
- AI in Financial Analytics: Risk Management Applications
- How AI Enhances Social Media Analytics
- The Future of AI in Data Analytics: Trends to Watch
- Everything You Need to Know About AI Data Analytics
Comments are closed.