In today’s fast-paced world, businesses need to stay ahead of the curve. Predictive analytics powered by AI tools offers a way to gain that competitive edge. These tools can help forecast trends, improve decision-making, and enhance efficiency across various operations. But with so many options available, how do you choose the best AI tools for your predictive analytics needs? This article dives deep into understanding what to look for in AI tools, and how to select the best ones for your business.
Understanding Predictive Analytics
The Evolution of Predictive Analytics
Predictive analytics has evolved significantly over the past few decades, driven by advancements in data processing power and the availability of big data. Initially, predictive analytics was limited to simple statistical models and required specialized knowledge to interpret the results. Today, AI and machine learning have transformed predictive analytics into a powerful tool accessible to businesses of all sizes.
For startup founders, understanding this evolution is crucial. Modern predictive analytics leverages complex algorithms that can analyze vast amounts of data in real-time, providing insights that were previously unattainable. This means that even startups with limited resources can now harness the power of predictive analytics to drive their business forward.
Core Components of Predictive Analytics
To effectively implement predictive analytics, it’s essential to understand its core components: data collection, data preparation, model building, and model deployment. Each component plays a critical role in the overall process, and mastering these can significantly enhance your predictive capabilities.
Data collection involves gathering relevant data from various sources, such as customer transactions, social media interactions, and market trends. As a startup, ensure you are capturing comprehensive data that reflects your business operations and customer behavior. This data forms the foundation of your predictive models.
Data preparation is the process of cleaning and organizing the collected data. This step is crucial because the quality of your data directly impacts the accuracy of your predictions. Use tools that can automate data cleaning to save time and reduce errors. Ensure that your data is consistent, free of duplicates, and properly formatted.
Model building involves selecting and training algorithms to analyze the prepared data and make predictions. This is where the power of AI comes into play. Choose algorithms that best fit your data and business needs. For instance, if you are looking to predict customer churn, use algorithms known for their accuracy in classification tasks.
Model deployment is the final step, where the trained model is put into production to generate predictions on new data. This requires integrating the model into your existing systems so it can continuously receive data and provide real-time insights. Monitor the performance of your deployed models regularly and retrain them as needed to maintain their accuracy.
Strategic Applications of Predictive Analytics for Startups
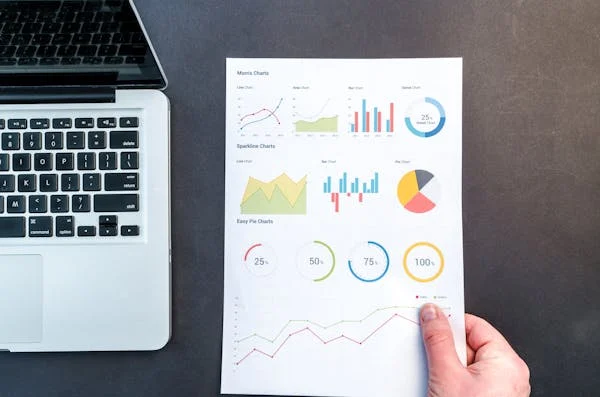
Predictive analytics can be applied to various aspects of a business, each offering strategic advantages. For startup founders, it’s essential to identify the areas where predictive analytics can provide the most significant impact.
In marketing, predictive analytics can help identify potential customers and personalize marketing campaigns. By analyzing customer behavior and preferences, you can create targeted campaigns that are more likely to convert. This not only improves your marketing ROI but also enhances customer engagement and satisfaction.
In sales, predictive analytics can forecast demand and optimize inventory levels. This ensures that you have the right products available at the right time, reducing stockouts and overstock situations. Accurate sales forecasting also helps in planning production schedules and managing supply chains more efficiently.
For customer service, predictive analytics can identify patterns in customer complaints and predict potential issues before they escalate. This allows you to proactively address problems, improving customer satisfaction and loyalty. Predictive analytics can also help in segmenting customers based on their likelihood to churn, enabling targeted retention strategies.
Overcoming Challenges in Predictive Analytics
While predictive analytics offers numerous benefits, it also comes with challenges. As a startup founder, it’s important to be aware of these challenges and develop strategies to overcome them.
One common challenge is data quality. Poor quality data can lead to inaccurate predictions, which can negatively impact decision-making. Invest in tools and processes that ensure your data is clean, accurate, and up-to-date. Regularly audit your data to identify and address any quality issues.
Another challenge is the complexity of predictive models. Building and interpreting these models require specialized knowledge, which may not be readily available in your startup.
Consider partnering with experts or using AI platforms that offer user-friendly interfaces and automated model-building capabilities. This can help you leverage predictive analytics without needing deep technical expertise.
Finally, integrating predictive analytics into your existing systems can be challenging. Ensure that your chosen AI tools offer strong integration capabilities and work seamlessly with your current infrastructure. This reduces the complexity of deployment and ensures that you can quickly start benefiting from predictive insights.
Long-Term Vision and Adaptation
Predictive analytics is not a one-time effort but an ongoing process that evolves with your business. As a startup founder, it’s essential to have a long-term vision for how predictive analytics will grow with your company. Regularly review your predictive models and adjust them based on new data and changing business conditions.
Stay informed about advancements in AI and predictive analytics to ensure that your startup remains at the forefront of technology. Encourage a culture of innovation and continuous learning within your team. This will help you adapt to new opportunities and challenges, ensuring that your predictive analytics efforts continue to deliver value over time.
Key Features to Look for in AI Tools
Comprehensive Data Integration
For startup founders, one of the most crucial features to look for in AI tools for predictive analytics is comprehensive data integration. This means that the tool should seamlessly connect with various data sources, such as CRM systems, social media platforms, ERP systems, and other relevant databases. Comprehensive data integration ensures that you can gather and analyze all necessary data without missing critical information.
When selecting an AI tool, verify its compatibility with your current systems. Look for tools that offer robust APIs and support a wide range of data formats. This will facilitate smoother integration and data flow, enabling a holistic view of your operations. Effective data integration allows for more accurate and comprehensive analysis, ultimately leading to better predictive insights.
Advanced Analytical Capabilities
While ease of use and scalability are important, the analytical capabilities of an AI tool are what truly determine its effectiveness. For startups aiming to leverage predictive analytics, it’s essential to choose tools that offer advanced analytical features such as machine learning, deep learning, and natural language processing.
Evaluate the tool’s ability to handle complex data sets and perform sophisticated analyses. Tools that support automated machine learning (AutoML) can be particularly beneficial as they simplify the model-building process and make it accessible to users with varying levels of expertise. Ensure the tool can perform predictive modeling, clustering, classification, and regression analysis to meet diverse analytical needs.
Real-Time Data Processing
In today’s fast-paced business environment, the ability to process and analyze data in real time is a game-changer. Real-time data processing allows you to make timely decisions based on the most current information. For startup founders, this means responding quickly to market changes, customer behaviors, and operational anomalies.
Choose AI tools that offer real-time analytics capabilities. These tools should be able to ingest data continuously and provide instant insights. Real-time data processing is particularly crucial for applications like dynamic pricing, fraud detection, and customer engagement, where timely responses can significantly impact outcomes. By leveraging real-time analytics, your startup can stay agile and responsive.
Strong Security and Compliance Features
Data security and compliance are paramount, especially when dealing with sensitive information. As a startup founder, it’s vital to choose AI tools that prioritize security and comply with relevant regulations such as GDPR, HIPAA, or CCPA.
Look for tools that offer robust security features like encryption, user authentication, and access controls. Ensure that the tool complies with industry standards and provides regular updates to address emerging security threats.
Additionally, the tool should offer audit trails and data lineage features, allowing you to track data usage and ensure compliance with regulatory requirements. Prioritizing security and compliance not only protects your data but also builds trust with your customers and stakeholders.
Customizable Reporting and Visualization
Effective communication of insights is critical for making data-driven decisions. AI tools should offer customizable reporting and visualization capabilities to help you present data in a clear and actionable manner. As a startup founder, you need tools that allow you to create tailored dashboards and reports that highlight the key metrics and insights relevant to your business.
Evaluate the tool’s ability to create interactive and visually appealing charts, graphs, and dashboards. Customizable reporting features enable you to adjust the presentation of data to suit different audiences, whether it’s your team, investors, or customers. The ability to drill down into specific data points and explore trends visually can enhance understanding and drive better decision-making.
Flexibility and Adaptability
The business landscape is constantly evolving, and your AI tools need to be flexible and adaptable to keep up with changes. Choose tools that can easily adjust to new requirements, whether it’s integrating new data sources, adopting new analytical techniques, or scaling up as your business grows.
Look for AI tools that offer modular and extensible architectures. This flexibility allows you to add new functionalities or integrate third-party solutions as needed. The ability to customize and extend the tool ensures that it remains relevant and useful as your business evolves. By choosing adaptable AI tools, your startup can stay ahead of technological advancements and maintain a competitive edge.
Cost-Effectiveness and ROI
For startup founders, managing costs is always a priority. While investing in advanced AI tools can provide significant benefits, it’s crucial to ensure that these tools are cost-effective and deliver a strong return on investment (ROI). Evaluate the total cost of ownership, including licensing fees, implementation costs, and ongoing maintenance expenses.
Consider the value that the AI tool brings to your business in terms of improved efficiency, better decision-making, and increased revenue. Look for case studies or testimonials from other startups to understand the potential ROI. Choosing cost-effective AI tools that deliver measurable results ensures that your investment contributes to the long-term success and sustainability of your startup.
User Community and Ecosystem
Having access to a strong user community and ecosystem can be incredibly beneficial when adopting new AI tools. A vibrant user community provides a platform for sharing best practices, troubleshooting issues, and learning from the experiences of others. Additionally, a robust ecosystem of partners, integrations, and add-ons can extend the capabilities of your AI tools.
Before selecting an AI tool, research the vendor’s user community and ecosystem. Join forums, attend webinars, and participate in user groups to gauge the level of support and engagement. A strong community and ecosystem can enhance your experience with the tool and help you maximize its potential.
Top AI Tools for Predictive Analytics
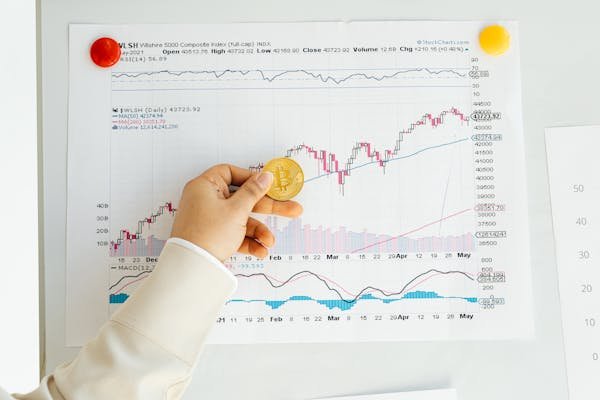
Microsoft Azure AI
Microsoft Azure AI provides a suite of AI tools that can be used for predictive analytics. It offers pre-built models and customization options, allowing businesses to tailor the tool to their specific needs. Azure AI’s strong integration capabilities and extensive support resources make it a reliable choice for businesses looking to leverage AI for predictive analytics.
SAS Predictive Analytics
SAS is well-known for its powerful analytics software, and its predictive analytics tools are no exception. SAS Predictive Analytics leverages machine learning, statistical analysis, and data mining to provide highly accurate forecasts.
It offers extensive customization options and integrates well with other SAS products and various data sources. The robust support and training resources make it easier for businesses to implement and maximize the use of this tool.
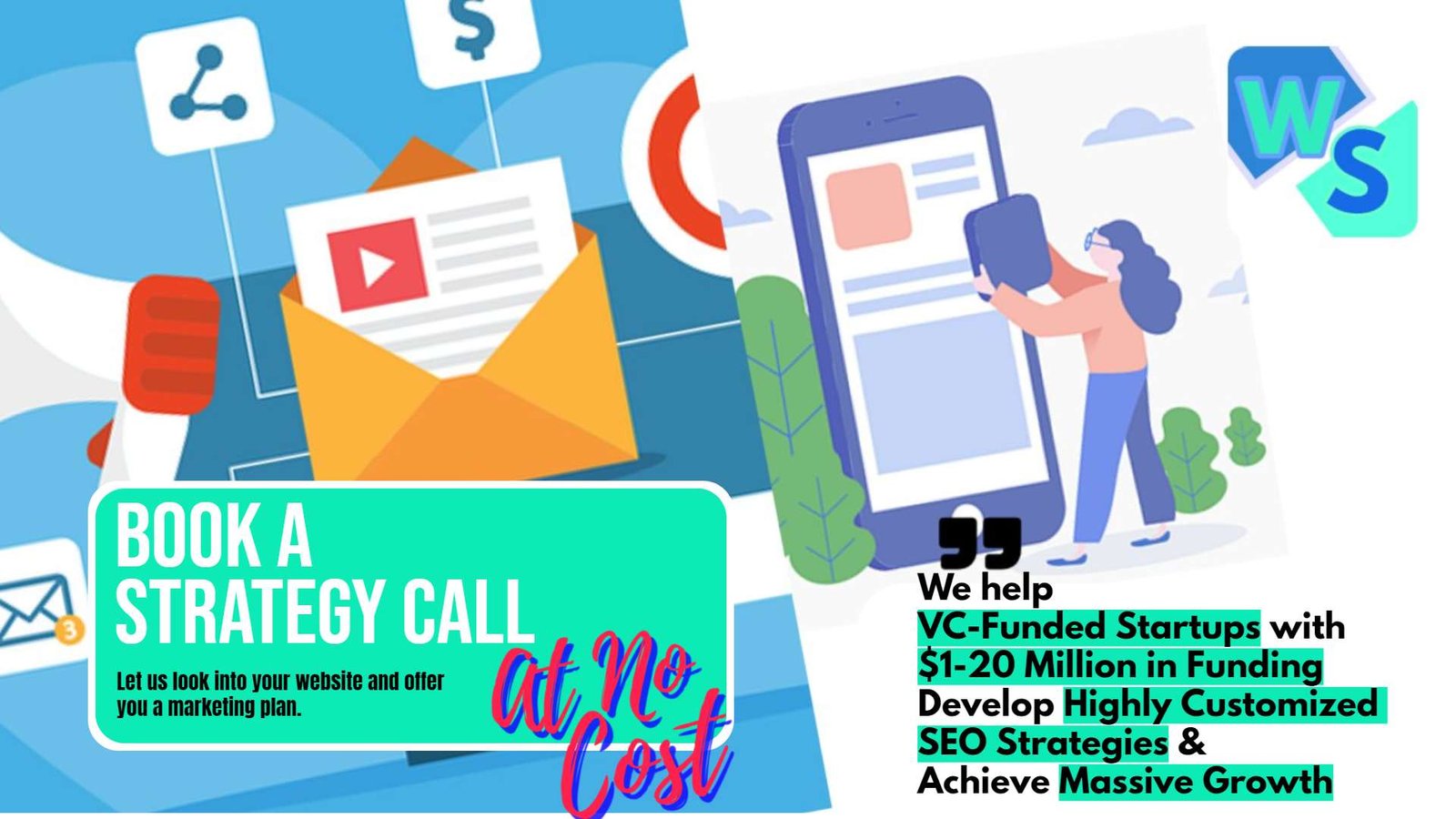
Related: Check out our free tools:
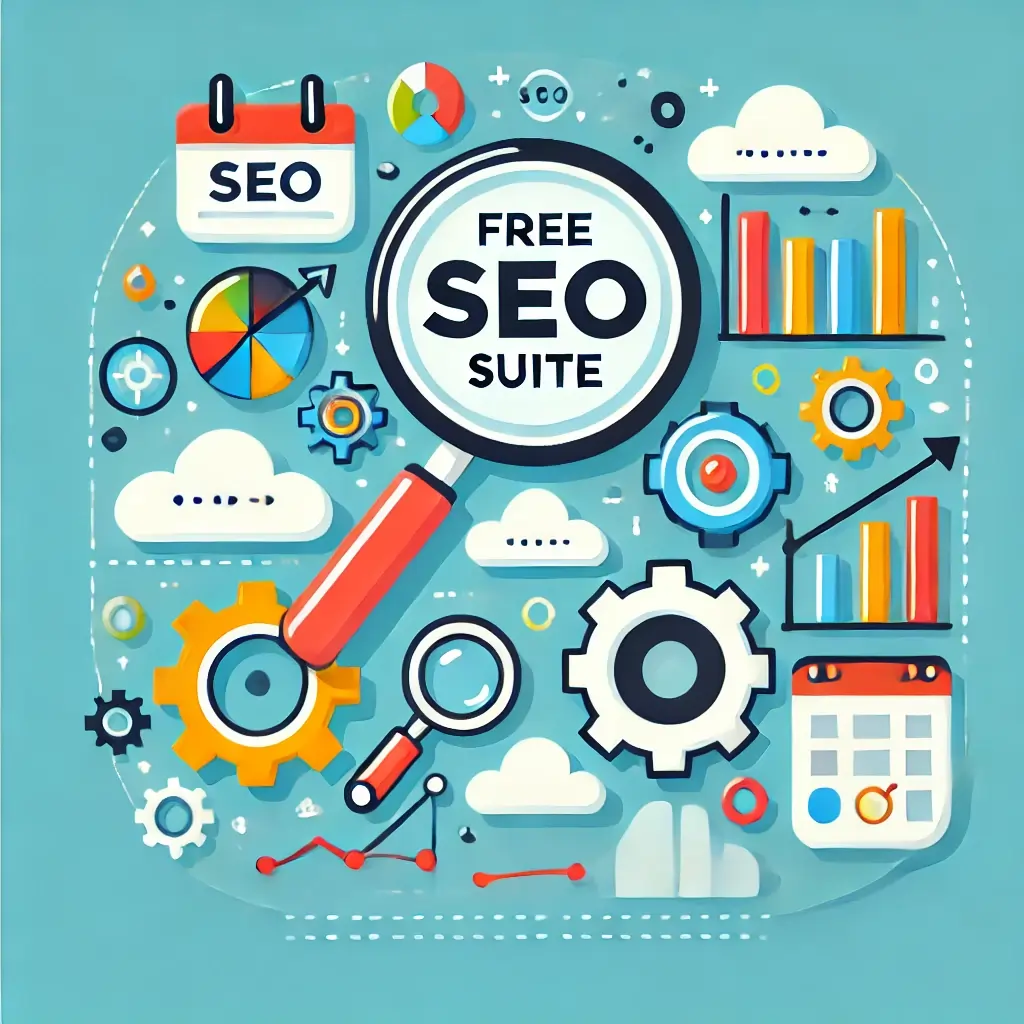
RapidMiner
RapidMiner is a versatile platform that provides end-to-end data science and machine learning solutions. It is designed to support the entire predictive analytics workflow, from data preparation to model deployment.
RapidMiner’s drag-and-drop interface makes it accessible even to users with limited coding experience. Its scalability and ability to handle large datasets make it an excellent choice for businesses looking to harness the power of AI for predictive analytics.
DataRobot
DataRobot automates the machine learning process, making it easier for businesses to build and deploy predictive models. It provides a user-friendly interface and automates many of the complex steps involved in predictive analytics, such as feature engineering, model selection, and hyperparameter tuning.
DataRobot’s automation capabilities help reduce the time and effort required to develop accurate predictive models, making it an ideal choice for businesses with limited data science resources.
H2O.ai
H2O.ai offers an open-source platform that provides robust machine learning and predictive analytics capabilities.
It supports a wide range of algorithms and integrates easily with popular data science tools like Python and R. H2O.ai’s scalability and flexibility make it suitable for businesses of all sizes. The platform also includes an AutoML feature that simplifies the process of building and deploying machine learning models.
Implementing AI Tools for Predictive Analytics
Assessing Your Needs
Before implementing AI tools for predictive analytics, it’s crucial to assess your specific needs and objectives. Identify the key areas where predictive analytics can provide the most value to your business.
This might include sales forecasting, customer behavior analysis, or operational efficiency improvements. Understanding your needs will help you choose the right tools and ensure that they align with your business goals.
Building a Skilled Team
Successful implementation of AI-driven predictive analytics requires a skilled team. This includes data scientists, analysts, and IT professionals who can manage the tools and interpret the insights generated.
Invest in training and development to build the necessary skills within your team. If needed, consider hiring external experts or consultants to provide additional support during the implementation phase.
Ensuring Data Quality
The accuracy of predictive analytics depends heavily on the quality of the data used. Ensure that your data is clean, accurate, and up-to-date. Implement processes for regular data cleaning and validation to maintain high data quality. Additionally, consider using data integration tools to combine data from different sources, providing a comprehensive dataset for analysis.
Setting Up the Infrastructure
Setting up the necessary infrastructure is a critical step in implementing AI tools for predictive analytics. This includes ensuring that you have sufficient computing power, storage, and network capabilities to handle the data and processing requirements. Cloud-based solutions can offer scalability and flexibility, making it easier to manage your infrastructure as your needs grow.
Starting with Pilot Projects
Begin with pilot projects to test the effectiveness of your chosen AI tools. Select a specific use case that can demonstrate the value of predictive analytics, such as predicting customer churn or optimizing inventory management.
Monitor the results closely and gather feedback from your team. Use the insights gained from these pilot projects to refine your approach and expand the use of AI tools to other areas of your business.
Continuous Monitoring and Improvement
AI-driven predictive analytics is not a one-time implementation but an ongoing process. Continuously monitor the performance of your predictive models and make necessary adjustments. Regularly update your data and retrain your models to ensure they remain accurate and relevant.
Encourage a culture of continuous improvement, where your team is always looking for ways to enhance the use of AI tools and derive greater value from predictive analytics.
Evaluating the ROI of AI Tools
Establishing Clear Objectives and KPIs
For startup founders, the first step in evaluating the ROI of AI tools for predictive analytics is to establish clear objectives and key performance indicators (KPIs). These objectives should align with your overall business goals and provide a framework for measuring success. Whether your aim is to improve customer retention, increase sales, or optimize operational efficiency, defining specific KPIs will help you track progress and quantify the benefits of your AI investment.
Begin by identifying the areas where AI-driven predictive analytics can have the most significant impact. Set measurable targets for each objective, such as a specific percentage increase in sales or a reduction in customer churn. These KPIs will serve as benchmarks to evaluate the performance of your AI tools and their contribution to your business outcomes.
Conducting a Cost-Benefit Analysis
Conducting a cost-benefit analysis is essential for understanding the financial implications of implementing AI tools. Start by calculating the total cost of ownership (TCO), which includes not only the initial purchase price but also ongoing expenses such as maintenance, training, and support. Compare these costs against the anticipated benefits, both direct and indirect, that the AI tools will bring to your business.
Direct benefits might include increased revenue from improved sales forecasting or cost savings from optimized inventory management. Indirect benefits could involve enhanced customer satisfaction, better decision-making, and reduced risk of human error. Quantify these benefits as accurately as possible and compare them to the costs to determine the net value added by the AI tools.
Monitoring Performance Metrics Over Time
Evaluating the ROI of AI tools is not a one-time task but an ongoing process. Continuously monitor performance metrics to assess how well the AI tools are meeting your established KPIs. This involves setting up regular review cycles to analyze data, gather feedback, and make necessary adjustments.
For example, if one of your KPIs is to reduce customer churn, track metrics such as customer retention rates, satisfaction scores, and engagement levels. Use this data to evaluate the effectiveness of your predictive analytics models and identify areas for improvement. Regular monitoring ensures that you can adapt to changing business conditions and maximize the benefits of your AI investment.
Considering the Intangible Benefits
While quantifiable metrics are crucial for evaluating ROI, don’t overlook the intangible benefits that AI tools can bring to your startup. These benefits, although harder to measure, can significantly impact your business’s long-term success. For instance, AI tools can enhance your company’s innovation capabilities, improve employee morale by automating mundane tasks, and strengthen your competitive position in the market.
Consider conducting qualitative assessments, such as employee surveys and customer feedback, to capture these intangible benefits. These insights can provide a more comprehensive view of the value that AI tools bring to your business and help you make informed decisions about future investments.
Assessing the Impact on Decision-Making
One of the significant advantages of AI-driven predictive analytics is its ability to improve decision-making processes. Evaluate how the implementation of AI tools has impacted the quality, speed, and accuracy of decisions within your organization. This can be particularly important for strategic planning, resource allocation, and risk management.
For instance, analyze how AI tools have influenced decisions related to product development, marketing strategies, and financial planning. Assess whether these decisions have led to better business outcomes, such as increased market share, higher profitability, or reduced operational risks. Improved decision-making is a critical factor in realizing the full ROI of AI tools.
Evaluating Time Savings and Efficiency Gains
AI tools can significantly reduce the time and effort required for data analysis and decision-making. Evaluate the time savings and efficiency gains achieved through the implementation of AI-driven predictive analytics. This includes assessing how much time your team spends on data preparation, model building, and interpretation of results compared to traditional methods.
Quantify the reduction in manual work and the acceleration of business processes enabled by AI tools. These efficiency gains can translate into cost savings and allow your team to focus on higher-value tasks. Understanding the impact on productivity and operational efficiency helps in assessing the overall ROI.
Benchmarking Against Industry Standards
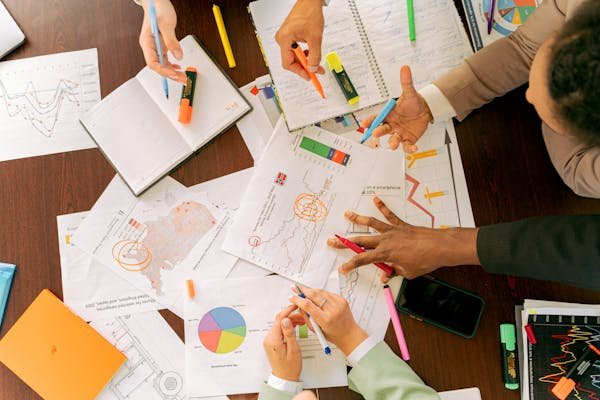
Benchmarking your AI tools against industry standards and best practices provides valuable context for evaluating ROI. Compare your performance metrics with those of similar companies in your industry to understand how well your AI initiatives stack up. This benchmarking can highlight areas where you excel and identify opportunities for further improvement.
Participate in industry forums, attend conferences, and engage with peer networks to stay informed about the latest trends and advancements in AI-driven predictive analytics. Learning from the experiences of other startups and industry leaders can provide insights into optimizing your AI investments and achieving better ROI.
Planning for Long-Term ROI
While immediate benefits are important, it’s also essential to consider the long-term ROI of AI tools. Predictive analytics is a long-term investment that can yield increasing returns as the models become more sophisticated and the data sets grow richer. Plan for continuous improvement and innovation to sustain and enhance the value of your AI tools over time.
Develop a roadmap for the ongoing development and refinement of your predictive analytics capabilities. This includes regular updates to your models, integration of new data sources, and adoption of emerging technologies. By focusing on long-term ROI, you ensure that your startup remains agile, competitive, and positioned for sustained growth.
Conclusion
Choosing the best AI tools for predictive analytics is a strategic decision that can significantly impact the success of your startup. By understanding the core components and benefits of predictive analytics, you can better appreciate how these tools can transform your business operations.
When selecting AI tools, focus on key features such as comprehensive data integration, advanced analytical capabilities, real-time data processing, strong security, customizable reporting, flexibility, cost-effectiveness, and a supportive user community.
Read Next:
- Transform Your Writing with AI Technology
- Social Media in the Workplace: Key Statistics and Best Practices
- How to Create Content with AI Tools
- How Much Time Do We Spend on Social Media? Key Statistics
- Budgeting for Small Business Marketing: Essential Statistics
Comments are closed.