This Article has been revised, edited and added to, by Poulomi Chakraborty.
- What is A/B Testing?
- Implementing A/B Testing Findings Across Digital Marketing Strategies
- Integrating Personalization into Website Content
- Expanding to Ad Campaigns
- Continuous Improvement
- Tailoring User Segmentation Based on Behavior
- Integrating Test Insights into Product Development
- Dynamic Content Personalization
- Predictive Behavioral Modeling
- Continuous Optimization Loop
- Collaboration Across Departments
- Leveraging A/B Testing for Enhanced User Experience Design
- Understanding the Role of UX in A/B Testing
- Conducting Effective UX A/B Tests
- Integrating UX Improvements Across Platforms
- Focusing on Mobile Experience Optimization
- Utilizing A/B Testing for Accessibility Improvements
- Streamlining the Checkout Process
- Integrating Real-time Feedback Mechanisms
- Leveraging Advanced Analytics for Deeper UX Insights
- Developing a Culture of Iterative Improvement
- Optimizing Social Media Strategies with A/B Testing
- The Importance of A/B Testing on Social Media
- How to Set Up Social Media A/B Tests
- Practical Application: Testing Post Timing
- Scaling Successful Tests
- Continuous Learning and Adaptation
- Crafting Targeted Messaging for Diverse Audiences
- Visual Optimization for Increased Interaction
- Timing and Frequency of Posts
- Integrating User-Generated Content
- Leveraging Social Proof for Conversion Optimization
- Integrating A/B Testing in Social Media with Broader Digital Marketing Tactics
- Advanced Analytical Techniques for Deeper Insights into A/B Testing Data
- Conclusion
In the ever-evolving world of digital marketing, the mantra for success seems to consistently boil down to one word: optimization. Among the various techniques at a marketer’s disposal, A/B testing stands out as a particularly powerful tool. This method isn’t just about changing elements at random; it’s about making informed, strategic decisions that lead to better results, be it in email marketing, website design, or ad campaigns. Today, we’ll dive deep into how A/B testing can be a game-changer, enhancing your marketing efforts through meticulous experimentation and analysis.
What is A/B Testing?
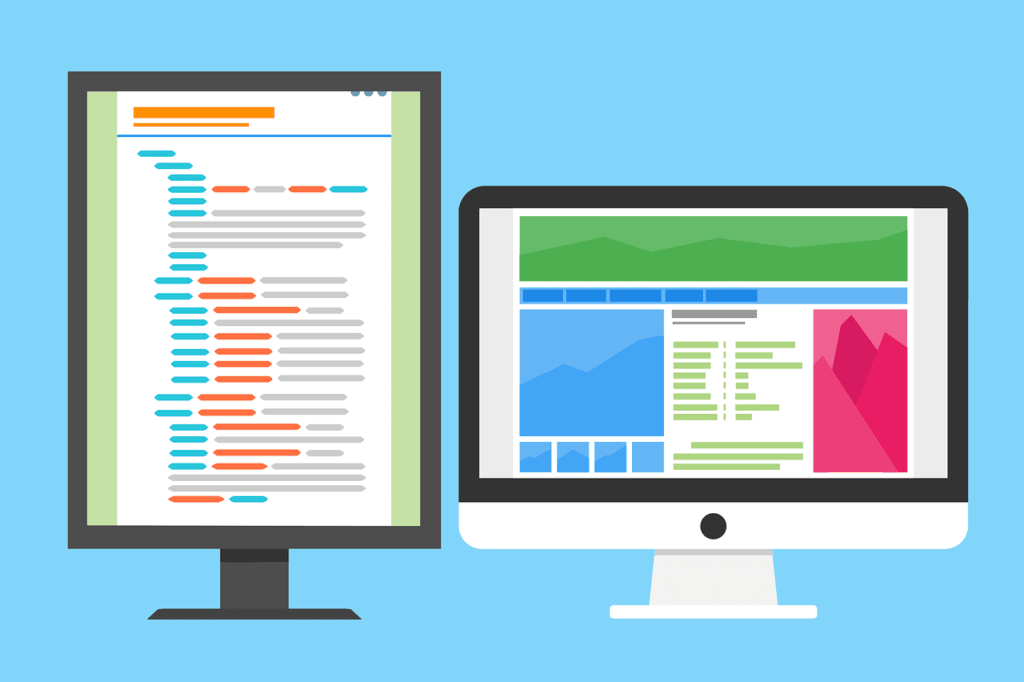
Imagine you’re a chef tweaking recipes to find the perfect balance of spices. A/B testing in digital marketing isn’t much different. It involves comparing two versions of a webpage, email, or ad to see which one performs better in terms of measurable outcomes like click-through rates, conversion rates, or any other specific goal you might have.
Let’s break down the essence of A/B testing through a practical scenario—optimizing an email campaign. We’ll explore the impact of different subject lines on open rates. This will give us a clear view of how small changes can lead to significant improvements in engagement.
Crafting the Perfect Email Subject Line
Email marketing remains one of the most effective channels for direct communication with customers. However, getting your emails opened is a challenge every marketer faces. Here, A/B testing comes into the picture, particularly in optimizing email subject lines, which are crucial for making a first impression.
Starting with a Hypothesis
The first step in any A/B test is to formulate a hypothesis. For our email campaign, our hypothesis could be: “Personalizing the email subject line with the recipient’s first name will increase open rates compared to a generic greeting.”
Designing the Test
To test this hypothesis, you would create two versions of your email:
- Version A (Control): “Our Biggest Sale Yet – Don’t Miss Out!”
- Version B (Variant): “John, Don’t Miss Our Biggest Sale Yet!”
Both versions would be identical in content and layout; the only difference is how the subject line addresses the recipient.
Executing the Test
You’ll send these emails to a small, statistically significant percentage of your total email list to ensure reliable results. Typically, this would be split 50/50 between the two versions, but the actual numbers can vary based on your list size and segmentation.
Analyzing the Results
After a set period, say 24 hours, you’d measure the open rates of both emails. Let’s assume the following results:
- Version A (Control): 15% open rate
- Version B (Variant): 22% open rate
With these results, it appears that personalizing the subject line has a clear advantage.
Why Does This Matter?
This outcome tells us not just about preferences, but also about behavioral trends. Personalization, as indicated by the results, can significantly impact engagement. By conducting this A/B test, you not only have a clearer path forward for your email campaigns but also validate the importance of tailoring communications in a way that resonates personally with recipients.
Implementing A/B Testing Findings Across Digital Marketing Strategies
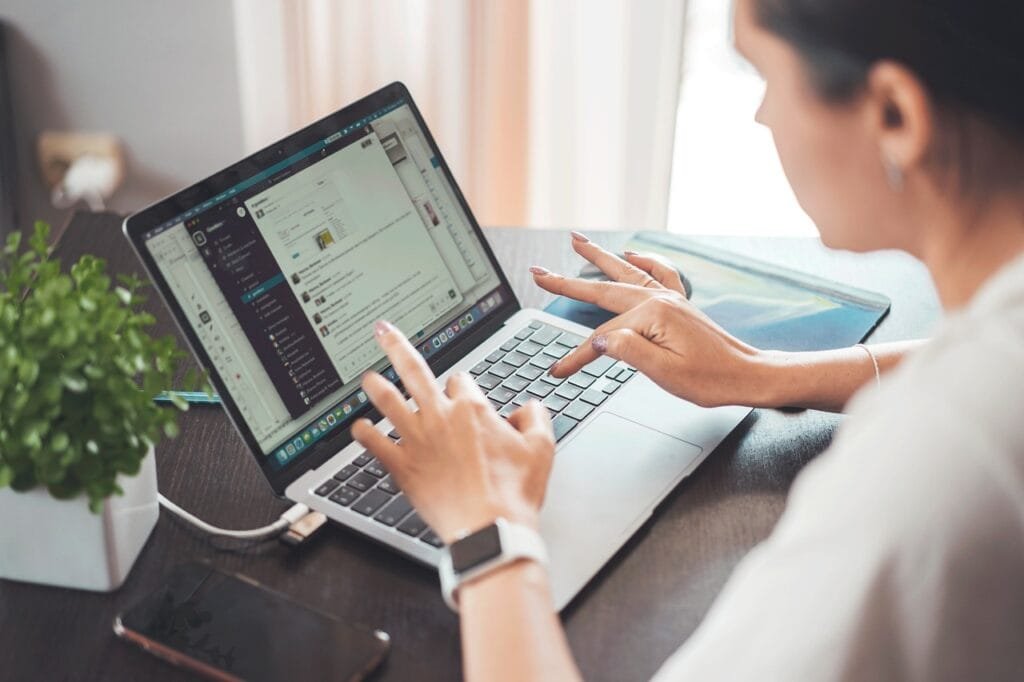
Having observed the positive impact of personalized email subject lines through A/B testing, the next step is leveraging these insights to enhance other areas of your digital marketing strategy. The power of personalization doesn’t stop with emails—it extends to every touchpoint in your customer journey.
Integrating Personalization into Website Content
The logic behind personalizing email subject lines can also be applied to website content. A/B testing can help you determine how personalized content affects user behavior on your site. For instance, you might test two versions of your homepage:
- Version A (Control): A standard welcome message.
- Version B (Variant): A welcome message that changes based on the visitor’s previous interactions with your site.
By analyzing metrics such as time on site, bounce rates, and conversion rates, you can gauge the effectiveness of personalized content in engaging visitors more deeply.
Developing a Test for Website Personalization
- Hypothesis: Adding personalized recommendations based on user browsing history increases engagement and sales.
- Test Design: Version A remains as your current homepage, while Version B incorporates elements like “Recommended for You” based on user’s past behavior.
- Execution: Similar to email testing, direct a segment of your traffic to each version and measure engagement.
- Analysis: Determine which version achieved higher engagement and sales.
Expanding to Ad Campaigns
A/B testing can similarly revolutionize your advertising efforts. Whether it’s Google Ads, Facebook, or Instagram, using insights from your email testing can help tailor your ad copies.
Example Test in Ad Campaigns
Suppose your email A/B test showed that a more direct, action-oriented call to action (CTA) performed better. You could apply this insight to your ads by testing:
- Version A (Control): A generic CTA, like “Learn More.”
- Version B (Variant): A more personalized and urgent CTA, like “Get Your Custom Plan Today!”
This kind of testing can reveal which phrases drive more clicks and conversions, allowing you to optimize your ad spend more effectively.
Continuous Improvement
The key to successful digital marketing lies in continuous optimization. Each A/B test provides data that refine your understanding of what resonates with your audience. Over time, these insights build a robust framework that guides all your marketing efforts, making each campaign more effective than the last.
Establishing a Testing Culture
To truly benefit from A/B testing, it’s essential to foster a culture of testing and learning within your organization. Encourage teams to:
- Regularly hypothesize based on data insights.
- Design and implement tests across different channels.
- Learn from test results and rapidly apply lessons.
Tailoring User Segmentation Based on Behavior
An often overlooked yet highly effective strategy following A/B testing is the refinement of user segmentation. Startups should consider how behaviors differ across customer segments in response to the tested elements.
For instance, if A/B testing reveals that younger audiences respond better to video content on social media platforms, a startup should consider customizing its marketing strategy to feature more dynamic, video-based content specifically targeted at this demographic on platforms they frequent. This strategic shift can lead to increased engagement and, consequently, higher conversion rates.
Integrating Test Insights into Product Development
Startups particularly benefit from integrating marketing insights into product development. If A/B testing indicates a strong preference for certain features or presentations in the marketing content, these preferences might suggest modifications or enhancements in the product itself.
For example, if users respond more favorably to a version of a web interface that highlights user-friendly features of a product, the product development team should consider bringing these features to the forefront in upcoming versions of the product.
Dynamic Content Personalization
Using A/B testing findings to drive dynamic content personalization is another powerful strategy. Startups can develop a system that adjusts the content displayed to users in real-time based on the preferences they have shown in their interactions with various test versions.
This approach not only makes the marketing efforts more relevant but also enhances user experience by aligning with the users’ known preferences, potentially increasing customer loyalty and lifetime value.
Predictive Behavioral Modeling
Building on A/B testing data with predictive behavioral modeling can propel a startup’s marketing strategy to new heights. By analyzing how different variables affected user actions, startups can create models that predict future behavior, allowing for more precise targeting and timing of marketing efforts.
For instance, if an A/B test shows that customers are more likely to purchase on weekends, predictive models can be used to ramp up advertising spend during these periods.
Continuous Optimization Loop
Creating a continuous optimization loop is crucial for sustained improvement. This process involves regularly scheduled A/B testing across all channels, ongoing analysis of the results, and the swift implementation of findings into marketing campaigns.
Moreover, it includes the measurement of the impact of those changes to determine if the expected improvements are realized. This method ensures that startups remain agile, with marketing strategies that evolve in tandem with changes in consumer behavior and market conditions.
Collaboration Across Departments
Finally, it is essential for startups to foster collaboration across departments—marketing, product development, customer service, and IT—to ensure that insights from A/B testing are shared and utilized effectively.
This collaborative approach ensures that strategic decisions are informed by data, are comprehensive, and consider multiple facets of the user experience.
Leveraging A/B Testing for Enhanced User Experience Design
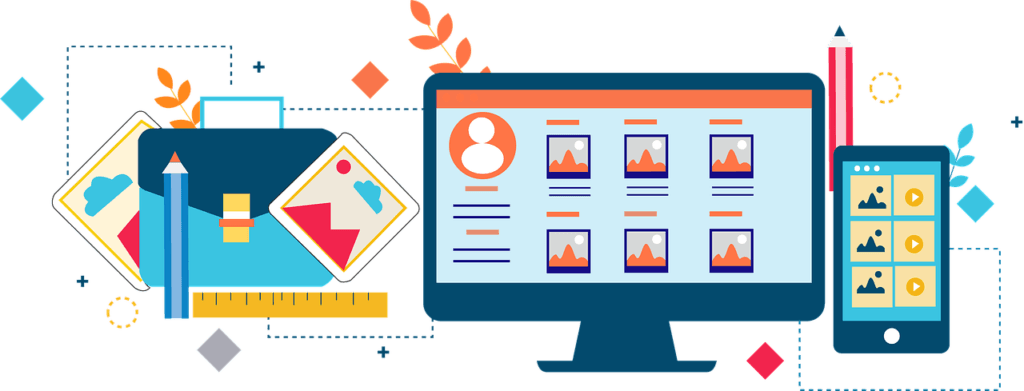
A crucial but often overlooked aspect of A/B testing in digital marketing involves enhancing the user experience (UX) design of websites and apps. A/B testing isn’t just about tweaking copy or adjusting layouts; it’s also about understanding how these changes affect the way users interact with your digital properties. By focusing on UX, you can significantly improve satisfaction, retention, and ultimately, conversion rates.
Understanding the Role of UX in A/B Testing
User experience is the sum of a user’s interactions with a company’s products or services, which directly influences their overall perception and satisfaction. In the context of A/B testing, improving UX means ensuring that every touchpoint within the digital environment is optimized for ease of use, efficiency, and enjoyment.
Example: Streamlining Navigation
Suppose you want to test whether simplifying the navigation of your online store affects customer satisfaction and sales. You might set up an A/B test like this:
- Version A (Control): The current, more complex navigation structure.
- Version B (Variant): A simplified navigation scheme with fewer, more clearly defined categories.
By tracking metrics such as page views per visit, time spent on the site, and conversion rates, you can assess whether a streamlined navigation leads to a better user experience and higher conversions.
Conducting Effective UX A/B Tests
Here are some critical steps to follow when setting up UX-focused A/B tests:
- Identify Friction Points: Use analytics and user feedback to identify areas where users seem to struggle or drop off. These are your prime candidates for testing.
- Develop Clear Hypotheses: Each test should start with a clear hypothesis about how a change will improve user experience. For example, “Reducing the number of steps in the checkout process will increase the checkout completion rate.”
- Create Measurable Outcomes: Define what success looks like in measurable terms. If the goal is to improve checkout completion, the primary metric could be the percentage of completed transactions.
- Test and Learn: After running the test, analyze the data to see which version performed better and why. Use these insights to make informed decisions about permanent changes.
Integrating UX Improvements Across Platforms
Once you’ve identified a successful change through A/B testing, it’s important to apply these learnings across all platforms where users interact with your brand. This consistency ensures that all users have a uniformly positive experience, whether they’re using a mobile app, desktop website, or anything in between.
Example: Optimizing Mobile Experience
If A/B testing on your website indicates that larger buttons improve navigation, consider applying this change to your mobile app as well. Since mobile users rely on touch interaction, larger buttons could significantly enhance usability and satisfaction on mobile devices.
Focusing on Mobile Experience Optimization
In today’s mobile-first world, ensuring that your digital products deliver a seamless mobile experience is crucial. Startups should prioritize A/B testing for their mobile interfaces, considering the smaller screen sizes and varying user conditions.
Testing elements like button size, menu navigation, and content layout on mobile devices not only improves usability but also affects overall engagement rates. For instance, experimenting with the placement and style of call-to-action buttons on a mobile app could reveal significant differences in user interaction, guiding more intuitive design adjustments.
Utilizing A/B Testing for Accessibility Improvements
Enhancing accessibility not only broadens your market but also improves the overall user interface for everyone. Startups should use A/B testing to experiment with different color contrasts, font sizes, and navigation structures to discover which configurations best support users with disabilities. This focus not only helps in complying with legal standards but also demonstrates a commitment to inclusivity, potentially increasing brand loyalty among a wider audience.
Streamlining the Checkout Process
For e-commerce startups, optimizing the checkout process is essential for reducing cart abandonment rates. By employing A/B testing on various checkout page designs, startups can identify friction points and test alternative methods of presenting checkout options that simplify the user’s journey.
Whether it’s testing the effectiveness of guest checkout options versus mandatory account creation or experimenting with the number of steps in the checkout process, each test can lead to a smoother, faster, and more user-friendly checkout experience.
Integrating Real-time Feedback Mechanisms
Incorporating real-time feedback mechanisms into A/B testing scenarios allows startups to gather immediate insights into user preferences and pain points. For example, implementing a feature that prompts users to rate their experience after using a newly tested feature can provide direct feedback and additional data points that inform further UX refinements. This method encourages continuous interaction with users, fostering a sense of involvement and satisfaction as they see their input actively shaping the product.
Leveraging Advanced Analytics for Deeper UX Insights
Advanced analytics tools and techniques, such as heatmaps and session recordings, can complement traditional A/B testing by providing a deeper understanding of how users interact with different versions of a webpage or app.
By analyzing where users click, how far they scroll, and where they spend most of their time, startups can gain a more comprehensive view of user behavior that goes beyond simple conversion metrics. These insights can then be used to refine the hypotheses for future A/B tests, ensuring that each iteration brings a closer alignment with user expectations.
Developing a Culture of Iterative Improvement
For startups, building a culture that embraces iterative improvement through regular A/B testing is fundamental. This approach ensures that the product evolves in response to actual user behavior and feedback, promoting gradual enhancements that accumulate over time.
It also fosters an environment where innovation is continuous and data-driven, allowing startups to stay agile and responsive to market demands and technological advancements.
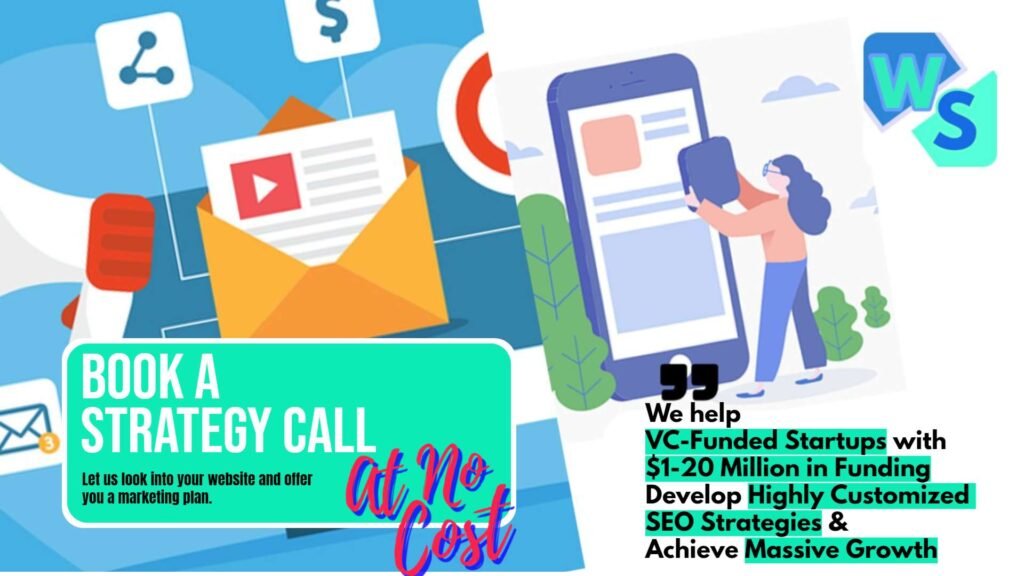
Related: Check out our free SEO suite
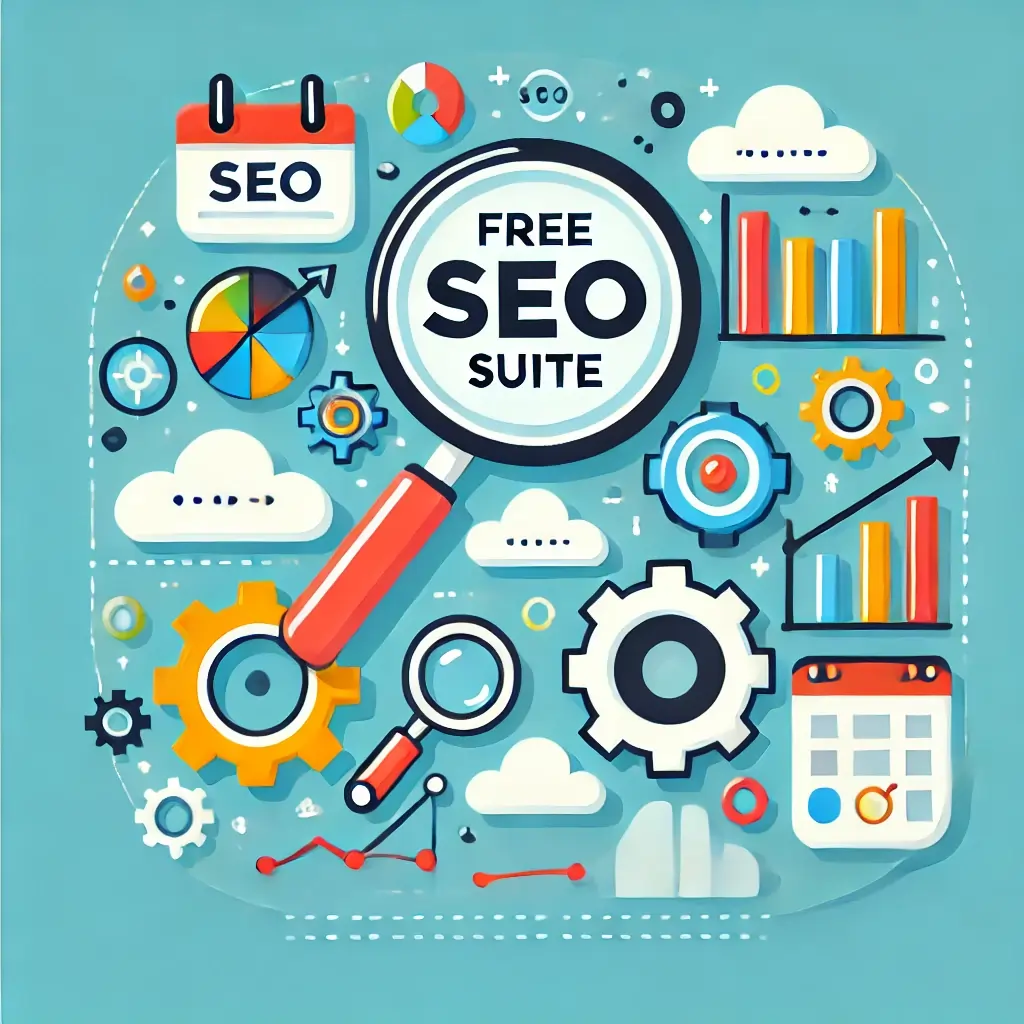
Optimizing Social Media Strategies with A/B Testing
Social media platforms provide a dynamic and highly visual environment for digital marketing, where even small changes can significantly impact user engagement and conversion rates. A/B testing in this context allows marketers to refine their strategies, ensuring content resonates with their audience while maximizing reach and effectiveness.
The Importance of A/B Testing on Social Media
Social media’s fast-paced nature requires that content not only captures attention quickly but also aligns closely with the interests and behaviors of targeted users. A/B testing here can involve elements such as post timing, content format, messaging, and visuals, each of which can be tuned to optimize engagement.
Testing Different Content Formats
Consider a scenario where you want to determine the most effective content type for your audience on a platform like Instagram. You could set up an A/B test comparing:
- Version A (Control): Photo posts
- Version B (Variant): Video posts
By measuring engagement metrics such as likes, comments, shares, and duration of interaction, you can identify which format keeps your audience more engaged.
How to Set Up Social Media A/B Tests
Here’s a step-by-step approach to implementing A/B testing for your social media campaigns:
- Select Your Variable: Choose one element to test at a time, such as post type, caption length, hashtags, or call-to-action (CTA) buttons.
- Create Two Versions: Develop two variants of the post, changing only the selected variable.
- Define Your Audience: Use the platform’s targeting features to ensure each post variant is seen by a similar and relevant segment of your audience.
- Measure Results: Use the platform’s analytics tools to track which version performs better based on predefined KPIs (Key Performance Indicators), such as engagement rate, click-through rate, or conversion rate.
Practical Application: Testing Post Timing
Timing can significantly affect social media performance, given the varying activity levels of users throughout the day. To find the optimal time for posting:
- Hypothesis: Posts published at 8 PM garner more engagement compared to those at 8 AM.
- Test Design: For a week, alternate posting times between 8 AM and 8 PM for similar types of content.
- Analysis: Compare engagement rates to see which timing yields better results.
Scaling Successful Tests
Once you identify a winning strategy through A/B testing, the next step is to scale this approach across similar campaigns and other social media platforms. However, keep in mind that each platform has its unique characteristics and audience behaviors, so what works on one may not work on another without adjustments.
Cross-Platform Testing
For instance, if video posts perform well on Instagram, test if the same applies on Facebook or TikTok, adjusting for any platform-specific nuances like video length or style. This holistic approach ensures your social media strategy is both robust and adaptable.
Continuous Learning and Adaptation
Social media trends and algorithms evolve rapidly, making continuous testing and adaptation crucial. Regularly schedule A/B tests to explore new hypotheses and refine your approach, staying ahead of changes in user behavior and platform algorithms.
Crafting Targeted Messaging for Diverse Audiences
Startups often face the challenge of addressing diverse audience segments within the same platform. A/B testing allows for the exploration of different messaging and content strategies tailored to specific demographic or psychographic segments.
For example, a startup could test two different tones in their posts—one informal and youthful, the other more polished and professional—to see which resonates better with different age groups within their audience. This approach not only increases the effectiveness of the content but also helps in refining the startup’s overall communication strategy.
Visual Optimization for Increased Interaction
Visual content plays a crucial role in social media engagement. By A/B testing various elements such as image styles, colors, and formats, startups can determine which visuals capture the most attention and encourage interaction.
Testing different video lengths might reveal optimal engagement times, while experimenting with different thumbnail images for the same video could show which is more likely to be played. This data-driven approach ensures that the visual content is not only attractive but also strategically aligned with user preferences.
Timing and Frequency of Posts
The timing and frequency of posts can dramatically affect their performance. Startups should use A/B testing to find the ideal times and frequencies for posting on each social media platform.
This could involve testing the engagement of posts at different times of the day or on different days of the week, as well as experimenting with more frequent posts versus quality-focused, less frequent updates. These tests help optimize the visibility and engagement of the content without overwhelming the audience.
Integrating User-Generated Content
Incorporating user-generated content (UGC) can significantly boost engagement and trust in a brand. Startups can use A/B testing to find the most effective ways to integrate such content into their social media strategy.
Testing how often and in what context UGC is shared can provide insights into how it impacts follower perception and interaction. For instance, alternating posts between company-generated content and UGC could reveal the perfect balance that maximizes engagement and encourages more users to share their own experiences with the brand.
Leveraging Social Proof for Conversion Optimization
Social proof, such as testimonials and reviews, can be powerful in converting followers into customers. A/B testing different methods of presenting social proof on social media—such as featuring customer testimonials in a video versus a quote graphic—can help startups identify the most compelling format.
Furthermore, testing the placement of these posts in the content calendar could optimize their impact, ensuring they reach the audience at a time when they are most effective.
Integrating A/B Testing in Social Media with Broader Digital Marketing Tactics
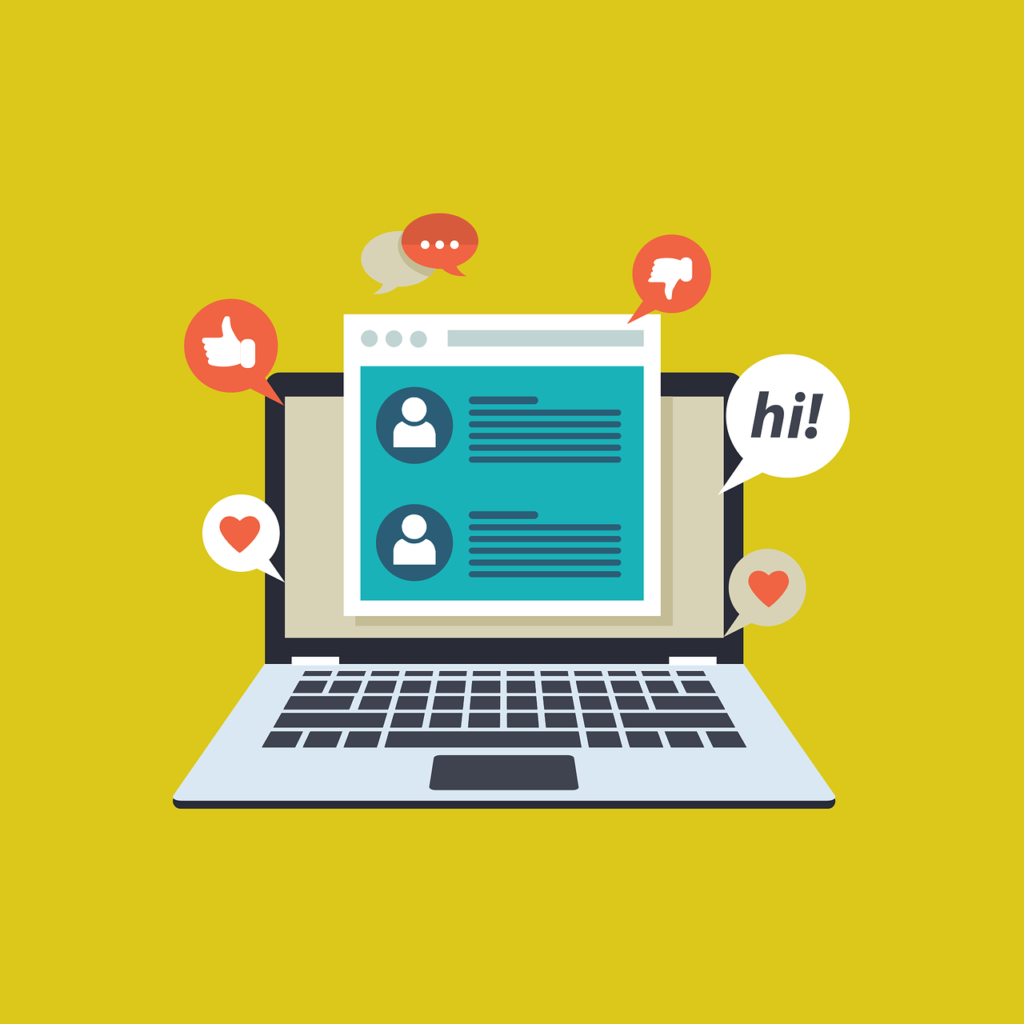
To achieve a holistic digital marketing strategy, it’s essential to not only understand how A/B testing can enhance individual channels, such as social media, but also how these findings can be integrated across all marketing efforts. This creates a cohesive user experience and amplifies your overall marketing impact.
Leveraging Insights Across Channels
The insights gained from A/B testing on social media can be incredibly valuable when applied to other areas of digital marketing. Here’s how you can leverage these insights:
Email Marketing
Suppose A/B testing on your social media platforms reveals that a particular type of messaging or visual content significantly boosts engagement. You can apply these successful elements to your email campaigns. For instance, if video content in social media posts leads to higher engagement rates, consider incorporating video into your email newsletters or promotional emails.
Website Content
Insights about which calls-to-action (CTAs) or content formats perform best on social media can inform the design and content of your website. For example, if you find that a specific CTA like “Learn More” outperforms others like “Buy Now” on social media, you might test the same approach on your website’s landing pages to see if it increases click-through rates.
Online Advertising
The visuals, headlines, and ad copy that yield the best results in social media A/B tests can also be used to enhance your online advertising campaigns. If a particular headline or image garners more clicks on Facebook, try using similar elements in your Google Ads or other PPC (pay-per-click) campaigns.
Coordinated Testing Across Platforms
To maximize the effectiveness of A/B testing across different digital marketing channels, coordinate your tests to ensure that you are learning from each platform and applying these lessons across the board.
Synchronized Testing Example
- Hypothesis Creation: Based on social media testing, hypothesize that shorter, action-oriented video ads will increase engagement on other platforms.
- Cross-Platform Testing: Implement this type of video on YouTube, in PPC ads, and on landing pages.
- Analysis and Adjustment: Measure performance across platforms and adjust based on which variations perform best in different contexts.
Building a Data-Driven Marketing Culture
Creating a culture that embraces data-driven decisions is fundamental to integrating A/B testing into your broader digital marketing strategy effectively. This involves:
- Regular Training: Ensure that all team members understand the importance of A/B testing and are trained in creating, implementing, and analyzing tests.
- Sharing Insights: Develop a system for documenting and sharing insights from A/B tests across teams and departments.
- Incorporating Feedback: Use customer feedback and behavioral data to continuously refine hypotheses and testing strategies.
Leveraging Technology and Tools
Utilize digital marketing tools and platforms that support A/B testing and analytics integration. Tools like Google Analytics, Optimizely, and various social media analytics platforms can help track performance and provide insights that can be shared across channels.
Advanced Analytical Techniques for Deeper Insights into A/B Testing Data
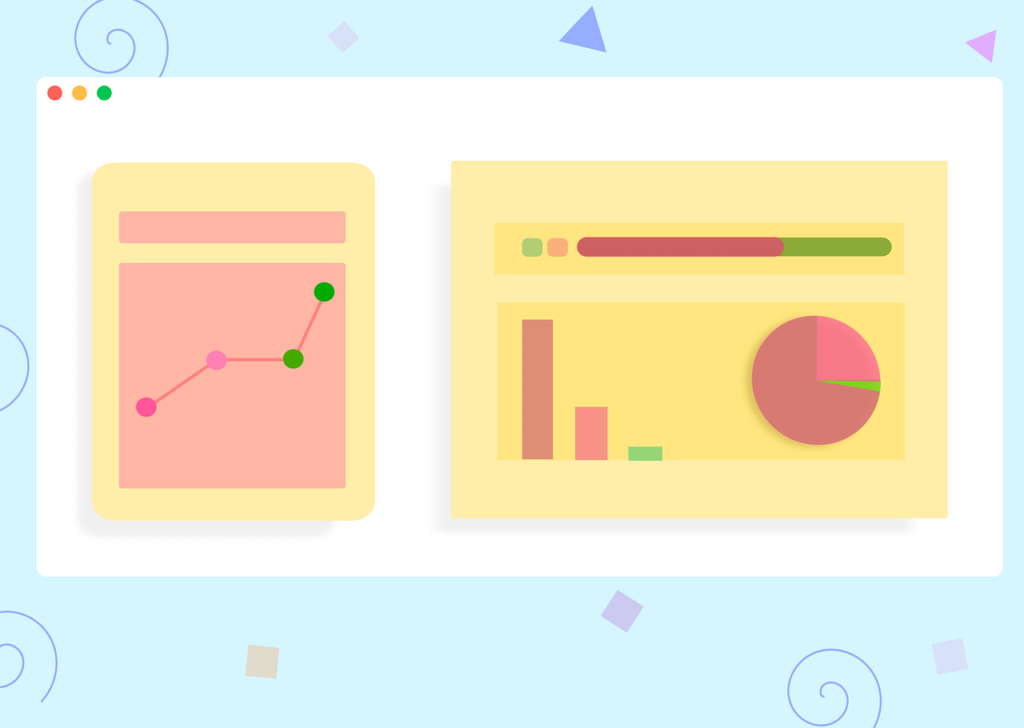
Diving deeper into the data from A/B testing can unlock valuable insights, enabling marketers to make more informed decisions. Advanced analytical techniques can help identify subtle patterns, measure long-term effects, and optimize marketing strategies more effectively. Here are some sophisticated methods to consider integrating into your A/B testing analysis.
Multivariate Testing
While A/B testing traditionally compares two versions of a single variable, multivariate testing allows you to test multiple variables simultaneously. This approach can reveal how different elements interact with each other and their combined effect on user behavior.
Implementation Example:
Suppose you’re testing a landing page. Instead of just testing two versions of a headline (A/B testing), you test two headlines, two images, and two CTA buttons in various combinations. This can help you understand which combination works best and why, providing a more comprehensive view of how these elements influence user decisions.
Statistical Significance and Confidence Intervals
To ensure that the results from your A/B tests are not due to random chance, it’s crucial to calculate statistical significance. This involves using statistical tests like the t-test or chi-squared test, depending on the type of data you have.
- T-test: Used for comparing the means from two groups (e.g., conversion rates from two versions of a webpage).
- Chi-squared test: Used for categorical data (e.g., click/no-click across different versions).
Additionally, using confidence intervals helps you understand the range within which the true effect likely lies, giving you a clearer picture of the potential impact of changes.
Predictive Analytics
Predictive analytics involves using historical data to predict future outcomes. In the context of A/B testing, predictive models can forecast the long-term effects of changes based on short-term test results.
Example of Predictive Analytics in Use:
Using data from previous A/B tests on email marketing campaigns, build a model to predict which types of subject lines are likely to result in higher open rates in the future. This can guide your strategy for crafting subject lines, making your campaigns more effective.
Bayesian Methods
Bayesian statistics provide another approach to interpreting A/B testing data. Unlike traditional methods, which treat the data as fixed to produce a single ‘best estimate’, Bayesian methods incorporate prior knowledge and update the probability of a hypothesis as more data becomes available.
Practical Application:
If you have historical data suggesting that personalization improves email engagement, you can use Bayesian methods to update the probability that personalization will be effective in your current campaign, based on the ongoing A/B test results.
Machine Learning Techniques
Machine learning can automate the analysis of complex A/B testing data, especially useful in large-scale tests with multiple variables. Algorithms can detect patterns and interactions between variables that might be missed through manual analysis.
Example:
Use clustering algorithms to segment your audience based on how different groups respond to variants in an A/B test. This can reveal more nuanced insights, like which segments are most sensitive to changes in a website’s UX design.
Integration with Big Data
Integrating A/B testing frameworks with big data technologies can help you manage and analyze large volumes of data from various sources (social media, website interactions, etc.). This approach enables a more holistic view of how changes affect user behavior across all touchpoints.
Implementation:
Deploy a data management platform (DMP) to collect and analyze data from all digital marketing channels. Use this integrated data to conduct A/B tests that are informed by a comprehensive understanding of your audience’s behaviors and preferences across channels.
Conclusion
In the world of digital marketing, A/B testing serves as a cornerstone for optimization, allowing marketers to refine strategies with precision. By extending beyond simple A/B tests to incorporate advanced analytical techniques such as multivariate testing, statistical significance assessments, predictive analytics, Bayesian methods, and machine learning, marketers can uncover deeper insights into consumer behavior and interaction patterns. These approaches not only enhance the immediate effectiveness of campaigns but also pave the way for more informed, data-driven decision-making processes in future strategies.
Furthermore, integrating these advanced methods with big data ensures a comprehensive analysis across multiple channels, providing a holistic view of the impact of marketing efforts. As we harness these sophisticated tools and techniques, the potential to tailor and optimize digital marketing initiatives becomes virtually limitless, promising not only to meet but exceed the evolving expectations of our target audiences.
Read Next
- Beginner’s Guide: How to Conduct Effective Keyword Research
- The Ultimate Guide to Keyword Research for Startup SEO
- 9 UX Design Principles that Boosts SEO for Finance Websites
- How to Leverage Social Media Reviews for SEO
- Facebook SEO for Financial Services: Our Take!
Comments are closed.